What does LAD mean in UNCLASSIFIED
LAD (Least Absolute Deviations) is a statistical method used to estimate the parameters of a statistical model by minimizing the sum of the absolute differences between the observed and predicted values. It is a robust alternative to the more commonly used least squares method, which minimizes the sum of the squared differences between the observed and predicted values.
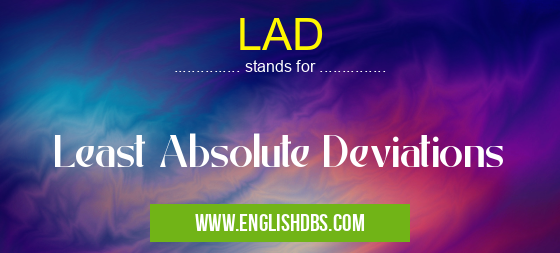
LAD meaning in Unclassified in Miscellaneous
LAD mostly used in an acronym Unclassified in Category Miscellaneous that means Least Absolute Deviations
Shorthand: LAD,
Full Form: Least Absolute Deviations
For more information of "Least Absolute Deviations", see the section below.
Characteristics of LAD
- Robustness: LAD is less sensitive to outliers in the data than least squares, as the absolute deviations are not squared. This makes it more suitable for data sets that contain extreme values or outliers.
- Interpretability: The LAD estimate is the median of the residuals, which is often more interpretable than the least squares estimate, which is the mean of the residuals.
- Computational efficiency: LAD is often computationally faster than least squares, especially for large data sets.
Applications of LAD
LAD is used in various applications, including:
- Regression analysis: Estimating the parameters of a regression model.
- Time series analysis: Modeling and forecasting time series data.
- Robust statistics: Identifying and dealing with outliers in data sets.
- Non-parametric statistics: Analyzing data without assuming any specific distribution.
Essential Questions and Answers on Least Absolute Deviations in "MISCELLANEOUS»UNFILED"
What is the Least Absolute Deviations (LAD)?
LAD, an abbreviation for Least Absolute Deviations, is a robust statistical technique used to estimate a regression model by minimizing the sum of the absolute differences between the observed values and the fitted values. LAD is less sensitive to outliers and extreme values compared to other estimation methods like Ordinary Least Squares (OLS).
What are the advantages of using LAD?
LAD has several advantages:
- Robustness: LAD is less affected by outliers and extreme values in the data, making it suitable for datasets with potential data contamination.
- Simplicity: The LAD objective function is straightforward to understand and compute, making the estimation process relatively simple.
- Interpretability: The LAD estimator can be interpreted as the median of the residuals, providing a clear understanding of the model's central tendency.
What are the limitations of LAD? A: LAD also has limitations: 1. Loss of Efficiency: LAD is generally less efficient than OLS when the data follows a normal distribution. In such cases, OLS produces more precise estimates. 2. Non-Gaussian Dat
LAD also has limitations:
- Loss of Efficiency: LAD is generally less efficient than OLS when the data follows a normal distribution. In such cases, OLS produces more precise estimates.
- Non-Gaussian Data: LAD assumes that the residuals follow a Laplacian distribution. If this assumption is violated, the LAD estimator may not be optimal.
- Computational Complexity: For large datasets, the LAD estimation process can be computationally more demanding than OLS.
When is it appropriate to use LAD?
LAD is suitable in the following situations:
- Outliers: When the dataset contains outliers or extreme values that could significantly influence the OLS estimates.
- Heavy-Tailed Distributions: If the residuals are expected to follow a heavy-tailed distribution, such as the Laplacian or Cauchy distribution.
- Robustness: When the focus is on obtaining robust estimates that are not easily affected by data contamination.
Final Words: LAD is a powerful statistical method that offers several advantages over least squares, particularly in the presence of outliers or extreme values. Its robustness, interpretability, and computational efficiency make it a valuable tool for a wide range of data analysis tasks.
LAD also stands for: |
|
All stands for LAD |