What does MAP mean in MATHEMATICS
Mean Average Precision or MAP is an important evaluation metric used in the machine learning and data science fields. It helps to measure the performance of predictive systems, including recommender systems, classifiers, and ranking models. It measures the average precision of a system's predictions over multiple queries. The higher the MAP value, the more accurate your system is at making predictions.
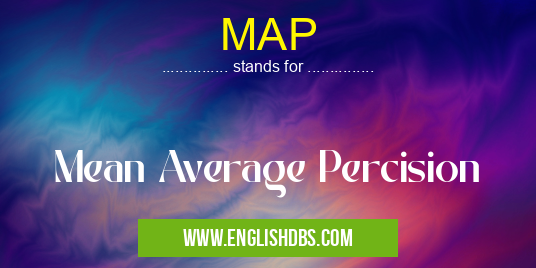
MAP meaning in Mathematics in Academic & Science
MAP mostly used in an acronym Mathematics in Category Academic & Science that means Mean Average Percision
Shorthand: MAP,
Full Form: Mean Average Percision
For more information of "Mean Average Percision", see the section below.
Essential Questions and Answers on Mean Average Percision in "SCIENCE»MATH"
What is MAP?
MAP stands for Mean Average Precision. It is a measure of the accuracy and performance of a computer vision algorithm on a given dataset. MAP measures how well an algorithm can correctly identify objects in an image or video, in terms of precision and recall.
What is the difference between Precision and Recall?
Precision measures how accurate an algorithm can classify objects in an image or video. Recall measures how complete the algorithm's classifications were; if there were any objects it failed to recognize that were actually present.
How do you calculate MAP?
The calculation for MAP involves computing the average precision over multiple queries or images. This precision is computed as the ratio of true positive matches divided by all correct predictions, including false positives.
Is MAP calculated with only one query?
No, MAP requires multiple queries to be calculated accurately. This allows for more reliable results and a better understanding of the overall accuracy and performance of an algorithm on a given dataset.
Does MAP require labeled data?
For meaningful measurements, yes, labeled data is necessary when calculating Mean Average Precision (MAP). A labeled dataset can include ground truth annotations such as object classes labels, bounding boxes coordinates etc., which are important for accuracy measurement purposes.
Does MAP take into account False Negative predictions?
Yes, False Negative predictions are taken into consideration when calculating MAP since they are considered incorrect predictions when evaluating overall accuracy and performance for an algorithm on a given dataset.
Is there a limit to the number of queries used when calculating MAP?
No, there isn't necessarily any limitation on the number of queries used in order to calculate Mean Average Precision (MAP). However, using too many queries could potentially slow down computations and resulting measurements may not be reliable due to over-fit models.
What types of datasets are suitable for calculating MAP?
Any type of dataset containing labeled data would be suitable for calculating Mean Average Precision (MAP). Examples include benchmark datasets with images containing various objects and scenes such as PASCAL VOC or ImageNet.
Final Words:
In conclusion, Mean Average Precision (MAP) is an important evaluation metric used in machine learning and data science fields to measure the performance of predictive systems such as recommender systems, classifiers, and ranking models. MAP looks at each data point being predicted and calculates a precision score for each query that was made. These scores are then averaged to get an overall mean average precision value which indicates how successful our predictions were when compared to actual results. By knowing this information we can make further improvements to our predictive models if needed or else use them with confidence that they will provide us with useful insights in real-world scenarios.
MAP also stands for: |
|
All stands for MAP |