What does MPFH mean in UNCLASSIFIED
Model Point Feature Histograms (MPFH) is a specialized type of histogram that is used for representing multi-scale image features. This tool can be used for applications such as object recognition, tracking, and clustering. It uses an array of feature points to create a histogram of the values at each point, which allows for a better understanding of the relationship between them. By taking into account multiple scales in its approach, MPFH can give more accurate results than traditional histograms.
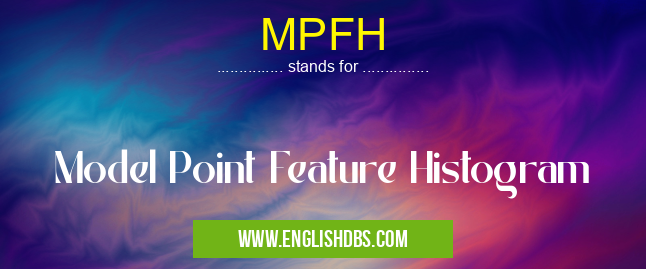
MPFH meaning in Unclassified in Miscellaneous
MPFH mostly used in an acronym Unclassified in Category Miscellaneous that means Model Point Feature Histogram
Shorthand: MPFH,
Full Form: Model Point Feature Histogram
For more information of "Model Point Feature Histogram", see the section below.
Benefits
The main benefit of MPFH is that it allows us to detect significant patterns within an image or video quickly and accurately. By combining information from multiple scales in one model point feature histogram, we can have better understanding of how these features interact with each other and gain insights into how objects appear in an image or video sequence over time. Additionally, MPFH requires very little data preprocessing compared to other visualization techniques thus making it suitable for use in real-time applications where speed is important.
Essential Questions and Answers on Model Point Feature Histogram in "MISCELLANEOUS»UNFILED"
What is a Model Point Feature Histogram (MPFH)?
A Model Point Feature Histogram (MPFH) is a type of histogram that models the feature relationships between different data points in an image or multidimensional space. It allows for the representation of multiple features from a single model point, making it easier to identify regions of interest and cluster similar features.
How is MPFH useful?
MPFH can be used to detect areas of similarity in datasets which may help uncover valuable insights into complex datasets such as recognizing patterns, clustering similar objects, and analyzing trends. Additionally, MPFHs can be used to find outliers or anomalies more quickly and easily.
What are the benefits of using MPFH?
Utilizing MPFH enables faster data analysis with less effort due to its ability to represent numerous features simultaneously. Additionally, by using MPFHs rather than traditional histograms, one can more easily identify patterns between objects while having greater accuracy im comparison with traditional methods such as clustering algorithms.
What types of datasets are suitable for analyzing with MPFHs?
Any dataset that involves multiple attributes can benefit from an analysis utilizing Model Point Feature Histograms (MPFH). This includes applications from images to speech recordings and anything in between.
Is there any special software I need to use MPFHs?
No special software is required for creating and visualizing Model Point Feature Histograms (MPFH); however, some specialized programs have been created that may simplify the process even further. These programs may also offer additional features such as parameter optimization capabilities.
Are there any limits on the size of datasets that can be analyzed using MPFHS?
Generally speaking, there are no limits on the size of datasets that can be analyzed with MPFHS; however, if your dataset is large enough that it takes longer than a reasonable amount of time to process, you should consider using other methods or breaking up your dataset into smaller subsets before running an analysis with an MPFHS.
Are there any disadvantages associated with using Model Point Feature Histograms (MPFHS)?
Yes there are some potential drawbacks associated with using Model Point Feature Histograms (MPFHS). Firstly, because this method models point feature relationships rather than their individual values it may not provide as accurate results when compared to other methods such as clustering algorithms. Secondly, when handling larger datasets it may take a long time to process due to its complexity.
Can I reuse my Model Point Feature Histogram (MPFHS) data for other projects?
Yes definitely! The data from your initial Model Point Feature Histogram (MPFHS) analysis can be used again in subsequent projects if needed without having to recreate the whole histogram each time.
How do I interpret my results from a Model Point Feature Histogram (MFPH)?
Your results will appear in the form of a graphical representation which displays various points representing different categories or classes across different dimensions. By interpreting this graph you should be able to identify both similarities between classes and also outliers which could signify interesting findings.
Final Words:
Model Point Feature Histograms (MPFH) are useful tools for image analysis due to their ability to accurately investigate relationships between multiple feature points while simultaneously being relatively easy to use and understand. The advantages of this technique are significant when it comes to analyzing objects over time in videos or still photos as well as recognizing new objects quickly and efficiently while using minimal data preprocessing steps. As technology continues to advance rapidly, Model Point Feature Histograms will continue to be instrumental in helping improve various computer vision tasks such as surveillance systems or automated driving software.