What does DDRO mean in UNCLASSIFIED
Data Driven Robust Optimization (DDRO) is a powerful technique used in various fields for decision-making under uncertainty. It combines data-driven approaches with robust optimization methods to enhance the reliability and robustness of optimization models.
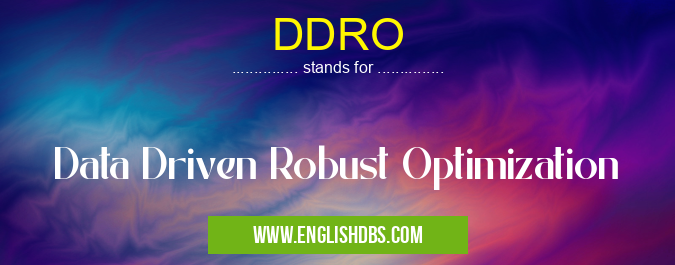
DDRO meaning in Unclassified in Miscellaneous
DDRO mostly used in an acronym Unclassified in Category Miscellaneous that means Data Driven Robust Optimization
Shorthand: DDRO,
Full Form: Data Driven Robust Optimization
For more information of "Data Driven Robust Optimization", see the section below.
DDRO Meaning
DDRO involves leveraging historical data or real-time observations to inform and improve the optimization process. By incorporating data, DDRO aims to:
- Capture uncertainties: Identify and quantify uncertainties in the optimization problem.
- Improve model accuracy: Enhance the accuracy of optimization models by incorporating real-world data.
- Increase robustness: Design solutions that are resilient to uncertain conditions.
- Optimize decision-making: Make informed decisions that consider both historical data and potential uncertainties.
Applications
DDRO finds application in a wide range of domains, including:
- Supply chain management
- Risk management
- Financial planning
- Engineering design
- Energy optimization
Benefits of DDRO
- Enhanced decision-making: Provides more accurate and reliable decision-making under uncertain conditions.
- Improved robustness: Designs solutions that are less sensitive to uncertainties and disruptions.
- Data-driven insights: Leverages data to gain insights into system behavior and make better predictions.
- Adaptive optimization: Allows for ongoing model updates and adjustments based on new data.
Essential Questions and Answers on Data Driven Robust Optimization in "MISCELLANEOUS»UNFILED"
What is Data Driven Robust Optimization (DDRO)?
Data Driven Robust Optimization (DDRO) is an optimization approach that combines data-driven modeling with robust optimization techniques. It addresses the challenge of optimizing decisions under uncertainty by leveraging historical data to capture the variability and uncertainty in the problem parameters.
What are the benefits of using DDRO?
DDRO offers several benefits, including:
- Improved decision-making: By incorporating data into the optimization process, DDRO can provide more accurate and reliable decisions.
- Increased robustness: DDRO considers uncertainty by generating multiple scenarios based on historical data, leading to more robust solutions that are less sensitive to parameter variations.
- Data-driven modeling: DDRO leverages historical data to build probabilistic models of uncertain parameters, providing a more realistic representation of the problem.
How does DDRO differ from traditional optimization methods?
Traditional optimization methods typically assume that problem parameters are known with certainty. However, DDRO recognizes that parameters are often uncertain and incorporates this uncertainty into the optimization process by generating multiple scenarios. This leads to more conservative and resilient solutions.
What types of problems can DDRO be applied to?
DDRO is applicable to a wide range of problems involving uncertainty, such as:
- Supply chain management
- Finance and risk management
- Energy systems
- Healthcare
- Engineering design
How can I implement DDRO in my organization?
Implementing DDRO requires expertise in optimization, data modeling, and statistical techniques. It typically involves the following steps:
- Collect and analyze historical data.
- Build probabilistic models of uncertain parameters.
- Formulate the optimization problem as a DDRO model.
- Implement the model using an appropriate optimization solver.
Final Words: DDRO is a valuable technique that enhances the reliability and robustness of optimization models. By incorporating data and considering uncertainties, DDRO enables organizations to make informed decisions, mitigate risks, and improve performance in various applications. Its data-driven approach and adaptive nature make it a powerful tool for optimizing decision-making under uncertainty.