What does DPS mean in UNCLASSIFIED
DPS stands for Dynamic Point Selection. It is a technique used in computer graphics to improve the quality of rendered images by dynamically selecting the points that are used to represent the surface of a 3D model.
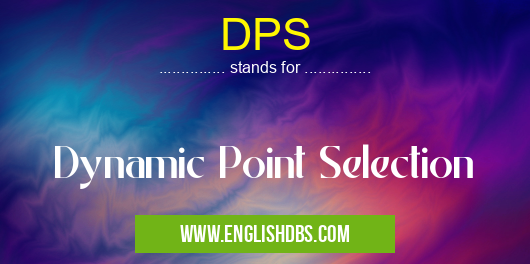
DPS meaning in Unclassified in Miscellaneous
DPS mostly used in an acronym Unclassified in Category Miscellaneous that means Dynamic Point Selection
Shorthand: DPS,
Full Form: Dynamic Point Selection
For more information of "Dynamic Point Selection", see the section below.
DPS: Introduction
How DPS Works
DPS works by dividing the surface of the 3D model into a set of points. The points are then used to create a mesh, which is a collection of triangles that approximate the surface of the model. The points are selected in such a way that the mesh is as accurate as possible while using as few points as possible.
Benefits of DPS
DPS has a number of benefits over traditional point selection methods. These benefits include:
- Improved image quality: DPS can produce images that are more accurate and realistic than images that are rendered using traditional point selection methods.
- Reduced rendering time: DPS can reduce the time it takes to render an image by using fewer points to represent the surface of the model.
- Improved performance: DPS can improve the performance of 3D applications by reducing the amount of memory that is required to store the mesh.
Conclusion
DPS is a powerful technique that can be used to improve the quality, speed, and performance of 3D rendering. It is a valuable tool for anyone who works with computer graphics.
Essential Questions and Answers on Dynamic Point Selection in "MISCELLANEOUS»UNFILED"
What is Dynamic Point Selection (DPS)?
DPS is a technique used in machine learning to automatically select the most informative and discriminative points from a dataset for training a predictive model.
What are the advantages of using DPS?
DPS offers several advantages:
- Improved model performance: By selecting the most relevant points, DPS helps improve the accuracy and generalization capabilities of the trained model.
- Reduced training time: Since DPS focuses on the most informative points, it reduces the number of training instances required, leading to faster training times.
- Enhanced interpretability: DPS helps identify the key features or instances that contribute most to the model's predictions, enhancing its interpretability.
How does DPS work?
DPS typically involves the following steps:
- Data sampling: A subset of data points is randomly sampled from the original dataset.
- Model training: A predictive model is trained using the sampled data.
- Point evaluation: The informativeness and discriminative power of each data point are evaluated based on the trained model.
- Point selection: The most informative and discriminative points are selected based on the evaluation results.
What are the criteria used for point evaluation in DPS?
Different criteria can be used for point evaluation, including:
- Prediction error: The difference between the predicted and actual labels for each point.
- Entropy: A measure of the uncertainty associated with the predictions for each point.
- Distance to the decision boundary: The distance between each point and the boundary separating different classes.
How is DPS applied in practice?
DPS can be applied to various machine learning tasks, such as:
- Classification: Selecting the most informative data points for training a classifier.
- Regression: Identifying the most relevant points for predicting continuous values.
- Anomaly detection: Detecting unusual or anomalous points in a dataset.
DPS also stands for: |
|
All stands for DPS |