What does BMAS mean in UNCLASSIFIED
BMAS stands for BioClinica Multi Atlas Segmentation, a type of segmentation technique used to analyze medical image data. This method uses multiple images from the same modality to make an accurate and reliable segmentation of medical images. It is a highly effective technique used in both clinical and research settings. BMAS is used to identify areas of interest in images such as tissue or organs for further analysis and diagnostics.
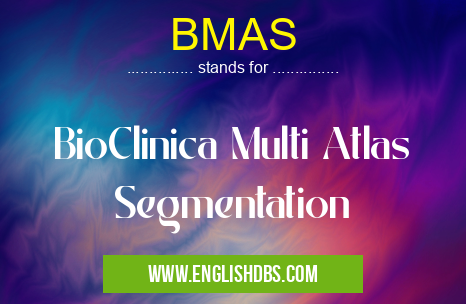
BMAS meaning in Unclassified in Miscellaneous
BMAS mostly used in an acronym Unclassified in Category Miscellaneous that means BioClinica Multi Atlas Segmentation
Shorthand: BMAS,
Full Form: BioClinica Multi Atlas Segmentation
For more information of "BioClinica Multi Atlas Segmentation", see the section below.
Working Principle
The BioClinica Multi Atlas Segmentation (BMAS) technique consists of using multiple atlas images that are acquired from the same imaging modality to guide the segmentation task on a target image. The concept is based upon the idea that several atlases can be compared with each other, and then combined using various algorithms like majority voting, masking or merging techniques. After this process, a refined result can be obtained which allows to accurately delineate anatomical regions within the target image. This method can also be applied in conjunction with machine-learning techniques such as artificial neural networks (ANN) or support vector machines (SVM). These techniques allow for faster computation times when compared with traditional methods like manual contouring due to their ability to learn from given examples quickly and accurately. Additionally, these techniques are less prone to errors caused by human subjectivity when making decisions related to segmentation tasks.
Advantages
One main advantage of using BMAS over traditional methods is its accuracy rate; this method has been shown to produce more accurate results than manual contouring and other existing automated methods like snakes or active shape models. Additionally, it requires fewer resources because it only needs access to multiple atlas images instead of large datasets of labeled data that many machine learning methods require. Lastly, this technique is also faster than some manual approaches since it does not need manual tracing of objects in each image. Therefore, BMAS presents an attractive option for dealing with medical imaging tasks efficiently while maintaining accuracy levels comparable with traditional methods.
Final Words:
In conclusion, BMAS is a powerful tool for analyzing medical imaging data due its high accuracy rate, resource savings potential and speed advantages over manual annotation techniques. The combination of using multiple atlas images along with various computational algorithms such as ANNs, SVMs or simple merging processes allows for highly reliable segmentations that can be used in clinical applications as well as research settings easily and rapidly.