What does BLRM mean in LOGISTICS
Bayesian logistic regression is a powerful tool that allows for predictive modeling of categorical variables. BLRM is used to build complex models using Bayesian inference techniques and further understanding the effects of predictors on outcomes. It uses data from historical observation or experiment to come to statistically valid conclusions about future events. BLRM is widely applied in many fields, such as banking, finance, insurance, healthcare, marketing and many more areas in business. It can be used to determine customer segmentation or credit risk scoring for example. In addition, its ability to handle large datasets makes it ideal for use in artificial intelligence (AI) algorithms and deep learning networks.
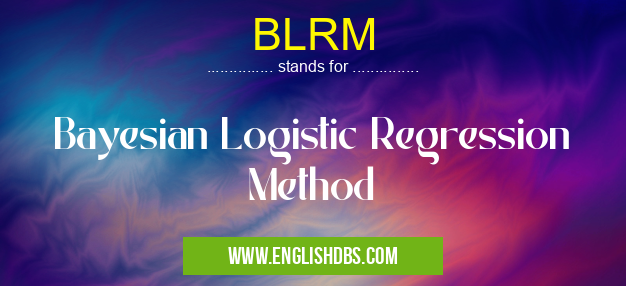
BLRM meaning in Logistics in Business
BLRM mostly used in an acronym Logistics in Category Business that means Bayesian Logistic Regression Method
Shorthand: BLRM,
Full Form: Bayesian Logistic Regression Method
For more information of "Bayesian Logistic Regression Method", see the section below.
Meaning
BLRM stands for Bayesian Logistic Regression Method which helps to build accurate predictions through discrete probability distributions within a statistical model. This method combines probabilistic principles with a linear regression technique and uses Bayesian theory in order to determine the probability distribution of an event given a set of predictor values. The result of the model provides information about how certain predictor variables influence the likelihood of an outcome occurring or not occurring.
Benefits
The main advantage of BLRM over traditional logistic regression techniques is its capacity to provide insight into uncertainty associated with predicted outcomes by incorporating prior knowledge regarding the problem domain into calculations. Additionally it offers flexibility when dealing with outliers or multiple data sources since it does not require assumptions about how data was generated - meaning results are more reliable when analyzing complex datasets like those found in business settings. Furthermore it allows investigators to incorporate prior beliefs or concerns about predictors into predictions which provides meaningful insights quickly and accurately without having to collect additional data points.
Essential Questions and Answers on Bayesian Logistic Regression Method in "BUSINESS»LOGISTICS"
What is Bayesian Logistic Regression Method?
Bayesian logistic regression is a machine learning method for classifying data using bayesian statistics. This method incorporates prior knowledge about the data being analyzed to make more accurate predictions. It’s typically used in classification tasks, such as facial recognition, sentiment analysis, and text analysis.
How does Bayesian Logistic Regression Method work?
Bayesian logistic regression works by combining bayesian statistics with a logistic curve to form an equation that can be used to classify data. The equation takes into account prior knowledge of the data set to make predictions from unseen data. By taking into consideration different types of information, such as age, gender and location, the model is able to make better decisions when classifying data points.
What are the benefits of using Bayesian Logistic Regression Method?
One of the biggest advantages of using bayesian logistic regression is that it takes prior knowledge of the dataset into account when making predictions. This allows the model to be more accurate because it can better predict the behavior of unseen data points based on existing patterns in known data points. Additionally, this method offers good scalability and flexibility for different types of datasets without needing major adjustments in code.
How do we assess the performance of Bayesian Logistic Regression Method?
Performance assessment for bayesian logistic regression models can be done through a few different methods like cross validation or out-of-sample testing. Cross validation involves splitting a given dataset into two parts and then testing each part against one another to see which performs better on unseen test cases. Out-of-sample testing is similar but uses separate datasets instead of subsets within a single dataset. Both methods are useful for assessing how well a bayesian logistic regression model performs in real-world scenarios.
Is there any limitation with Bayesian Logistic Regression Method?
Yes, there are several potential limitations with bayesian logistic regression models including overfitting and computational complexity issues due to large amounts of input parameters for the model to handle. Additionally, models trained using this method can suffer from decay over time due to changes in underlying trends or datasets used for training.
What type of datasets are suitable for use with Bayesian Logisitic Regression Method?
Generally speaking, any type of dataset with numerical or categorical values can be used in bayesia logisitc regression models. However, certain types may provide more accurate results than others depending on what information is being pulled from them (e.g., image recognition from an image dataset). Additionally, datasets should contain meaningful labels so that they can be correctly classified by the model.
How often should I retrain my Bayesain Logisitic Regeession Model? A: There isn't necessarily an exact answer here as it depends on many things such as how quickly your dataset changes, what type of accuracy you need from your model and other factors like environmental conditions that may affect its performance over time. As a general rule however it's usually recommended that you retrain your model at least once every month if not sooner.[END] Q: Are there any special considerations when working with Bayesain Logisitic Regeession Model?
There isn't necessarily an exact answer here as it depends on many things such as how quickly your dataset changes, what type of accuracy you need from your model and other factors like environmental conditions that may affect its performance over time. As a general rule however it's usually recommended that you retrain your model at least once every month if not sooner.
Final Words:
In conclusion, BLRM (Bayesian Logistic Regression Method) is an effective tool for predicting categorical outcomes using probabilistic principles within a linear regression framework. It provides useful insights by allowing prior knowledge concerning factors influencing outcomes to be incorporated into predictions while also providing better accuracy than traditional methods when dealing with complex datasets in business contexts such as customer segmentation or risk scoring models.