What does WSPM mean in PHYSICS
Wavelet-based Statistical Parametric Mapping (WSPM) is a powerful method for analyzing and interpreting complex data in the field of neuroscience, medical imaging, and other related disciplines. It combines an advanced wavelet transform with statistical parametric mapping techniques to enable researchers to identify important features in their data. This technique can be used to reveal spatial patterns and relationships between variables that would otherwise be impossible to detect. WSPM allows researchers to delve deep into the complexities of their data and uncover hidden insights that may prove invaluable in furthering their research goals.
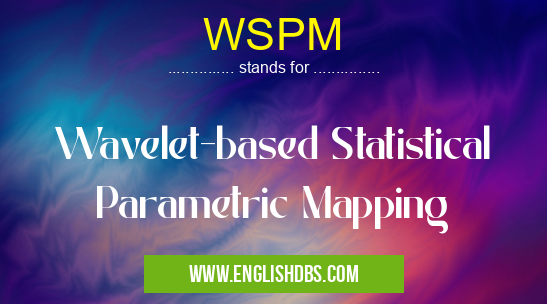
WSPM meaning in Physics in Academic & Science
WSPM mostly used in an acronym Physics in Category Academic & Science that means Wavelet-based Statistical Parametric Mapping
Shorthand: WSPM,
Full Form: Wavelet-based Statistical Parametric Mapping
For more information of "Wavelet-based Statistical Parametric Mapping", see the section below.
Explanation
WSPM is a sophisticated technique developed to extract meaningful information from complex datasets. It starts by using an advanced wavelet transform, which is a mathematical tool for decomposing signals or images into their component parts. This helps researchers identify key features in their datasets without having to introduce any assumptions about the underlying structure of the data itself. Once the decomposition has been completed, a statistical parametric mapping algorithm is then used to derive additional insight from the results. Statistical parametric mapping involves taking measurements or observations on variables or factors within a dataset, applying certain transformations where required, then comparing the results across multiple cases or scenarios. The aim is to highlight areas of significance within the dataset which would otherwise remain hidden beneath the noise of individual observations. By combining these two techniques, WSPM offers a powerful solution for analysing complex datasets and finding new correlations between variables that might otherwise have gone unnoticed. By manipulating wavelets and analysing them through statistical models, WSPM is able to unlock valuable insight with unprecedented accuracy and detail.
Essential Questions and Answers on Wavelet-based Statistical Parametric Mapping in "SCIENCE»PHYSICS"
What is Wavelet-based Statistical Parametric Mapping?
Wavelet-based Statistical Parametric Mapping (WSPM) is a statistical mapping technique used to analyze and visualize data. WSPM breaks down signals into individual frequency components for analysis, incorporating the power of wavelets in order to better understand complex patterns in data. WSPM allows for a more comprehensive interpretation of data than traditional linear models.
How does WSPM differ from traditional statistical mapping techniques?
Traditional statistical mapping techniques rely on linear models for analysis, but WSPM takes advantage of the nonlinear nature of wavelets to make more complex interpretations. With WSPM, individual frequency components are broken down from a signal in order to better understand its structure. This allows for a more comprehensive understanding of the data than what would be achieved through traditional linear models.
What are the benefits of using WSPM?
The major benefit of using WSPM is that it provides greater insight into complex patterns within data. By breaking down signals into their individual frequency components and analyzing them with wavelets, users can gain greater insight into how each component contributes to or affects the overall picture. Additionally, by relying on wavelet-based analysis as opposed to traditional linear models, users may gain deeper understanding by accounting for any nonlinearities present in their data set.
Are there any limitations associated with using WSPM?
As with any data analysis tool, there are certain limits and constraints associated with using WSPM. For one thing, it may produce inaccurate results when dealing with very noisy signals or weak signals that lack sufficient information content for meaningful interpretation. Additionally, since it relies on heavier computational resources than some other methods, it can sometimes be slower or not applicable when working with large datasets. Lastly, due to its complexity and reliance on mathematical theory beyond basic statistics knowledge, some users may find it difficult to understand how this method works or why certain results were produced.
Can I use WSPM without prior knowledge or experience?
While many researchers who have experience in programming and theoretical mathematics may find it easier to use WSPM without extensive training, those without such skills may need some guidance in order to properly utilize this tool effectively. It's important for less experienced researchers to understand the key concepts behind wavelets theory as well as how the process works before attempting any application of this tool.
Who can benefit from using Wavelet-based Statistical Parametric Mapping tools?
Anyone who seeks a greater level of understanding from their data can benefit from utilizing WSPM tools. Researchers looking to analyze audio signals, image processing professionals seeking new ways to detect abnormalities or features within images they are processing; statisticians looking for an alternate way to query large datasets; all these individuals can leverage the insights provided by this powerful methodology.
Does WSPM require special hardware or software?
Generally speaking, no specialized hardware is required in order to use Wavelet-based Statistical Parametric Mapping tools - however if you intend on utilizing parallel computing techniques (such as GPUs) then you will need additional hardware compatible with your chosen framework/platform/toolkit (e.g Tensorflow). In terms of software requirements - many popular open source libraries exist that allow you access these types of methods (ePyWavelets is one example).
Is Wavelet-based Statistical Parametric Mapping only used in research labs?
While most commonly used by academic researchers and statisticians interested in making sense out of their datasets - this technique has seen recent applications outside academia - such as autonomous vehicle platform engineering where signal processing technologies like sWPT help guide decision making algorithms.
How does using Wavelet-based Statistical Parametric Mapping compare against standard filtering techniques?
Standard filtering approaches discard parts of a signal based on assumptions about what's important vs what isn't - while wWPM retains all parts considered relevant based on how they interact both across and within frequency bands (i.e maximizing correlations while minimizing redundancies).
Final Words:
In conclusion, Wavelet-based Statistical Parametric Mapping (WSPM) is an innovative technique specifically designed to aid researchers as they attempt to uncover meaningful insights from complex datasets. By leveraging advanced wavelet transforms combined with statistical modelling algorithms, WSPM offers unparalleled accuracy when it comes to identifying important features or relationships within a given dataset that may provide valuable information for further study. In this way, WSPM provides researchers with a powerful tool for unravelling complex datasets and discovering new correlations between variables that may previously have gone unnoticed or undetected.
WSPM also stands for: |
|
All stands for WSPM |