What does WNQN mean in MATHEMATICS
Weighted Non-parametric Quantile regression (WNQN) is a powerful tool for non-linear data analysis that can be used to quickly estimate the parameters of a non-linear functional relationship. This method uses weighted data points to accurately model the underlying functional relationship between two variables or groups of variables. The weighted quantiles form an efficient way to address non-linear relationships and provide superior accuracy compared to other methods of non-linear regression. WNQN has been successfully applied to many areas, including finance, economics, engineering, and biostatistics.
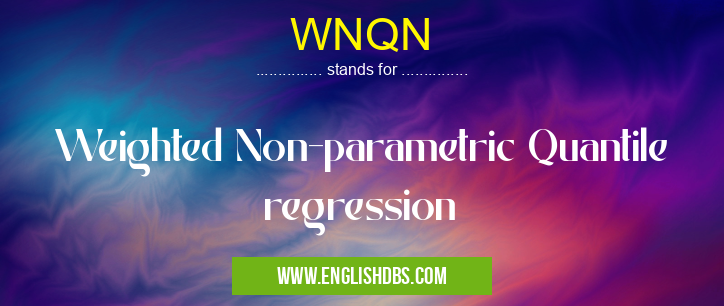
WNQN meaning in Mathematics in Academic & Science
WNQN mostly used in an acronym Mathematics in Category Academic & Science that means Weighted Non-parametric Quantile regression
Shorthand: WNQN,
Full Form: Weighted Non-parametric Quantile regression
For more information of "Weighted Non-parametric Quantile regression", see the section below.
Explanation
WNQN is a type of quantitative analysis that uses weighting techniques to determine the best fit of a given set of data points according to one or more predetermined criteria. The technique relies on weighted quantiles which are then used to calculate the best fitting parameters of the underlying function being studied. This enables researchers and practitioners in various fields to find accurate relationships between different variables without having to rely completely on linear regression models. Weighted Non-parametric Quantile regression is useful in accounting for any irregularities that may occur in the data due to outliers or other factors as it is able to identify and effectively weigh these factors accordingly.
Essential Questions and Answers on Weighted Non-parametric Quantile regression in "SCIENCE»MATH"
What is Weighted Non-parametric Quantile regression?
Weighted Non-parametric Quantile regression (WNQN) is a statistical method that uses weighted observations to estimate the parameters of nonlinear models. It can also be used to measure how a particular variable changes as another variable changes, such as predicting the price of a product given specific market conditions. WNQN allows for more precise predictions than traditional linear regression methods which assume all variables are independent and follow a linear pattern.
What are the benefits of using WNQN?
WNQN is beneficial because it allows for better predictions by taking into account the effect of multiple variables on one another. It takes into account nonlinear relationships between different variables, as well as heteroscedasticity, or unequal variance among observation groups. In addition, WNQN is robust to outliers, meaning it can still produce accurate results even with unusual observations.
How does WNQN differ from traditional linear regression methods?
Compared to traditional linear regression methods, WNQN does not assume that all variables are independent and have a linear relationship. Instead, it considers the interaction between different variables and their effects on other variables in order to produce more accurate predictions. Furthermore, WNQN is robust to outliers while traditional regression methods may produce invalid results when confronted with extreme data points.
When should I use Weighted Non-parametric Quantile regression?
You should use Weighted Non-parametric Quantile regression when you need to make accurate predictions that take into account nonlinear relationships between different variables or if you suspect there may be unequal variances among observation groups. WNQN is also recommended when there may be outliers present in your data set because it will still produce reliable results despite the presence of such data points.
How do I implement Weighted Non-parametric Quantile Regression?
To implement WNRQ you first need to determine which model you want to fit your data with and then choose appropriate weights for each observation within your dataset according to its importance or relevance in determining an outcome. Next, select an appropriate algorithm for computing quantiles and apply it on your dataset along with the weights assigned in step one in order to obtain parameter estimates for your chosen model type which can be used for predictions or further analysis.
How accurate can Weighted Non-parametric Quantile Regression be?
The accuracy of WNRq depends on several factors such as the number of observations included in your dataset and complexity of the model being fit by your data set but generally speaking we can expect more accurate results compared to traditional linear regression methods when dealing with nonlinear relationships and heteroscedasticity among observation groups.
Are there any limitations associated with using Weighted Non-parametric Quantile Regression?
One limitation associated with using this method lies in its reliance on user provided weights which may introduce bias into the resulting parameter estimates if chosen incorrectly or without consideration for confounding factors like correlation between different variables in your dataset.. Additionally, since this method relies heavily on computing power it may not be suitable if speed of processing is a concern such as real time analytics applications where faster alternatives would need to be sought after instead.
Does Weighted Non-parametric Quantile Regression require any special software or hardware requirements?
No special software or hardware requirements apart from having access to a computer powerful enough enough computations needed by this method are necessary when utilizing this method though specialized programs like MATLAB may provide certain helpful features depending on what one needs out of this method.
Can I use Weighted Non-parametric Quantile Regression for online applications such as real time analytics?
As mentioned previously due its reliance on computational power this method may not always be suitable for very fast applications like real time analytics where faster alternatives would need to be considered instead.
Can I use Weighted Non-parametric Quantile Regression over other types of quantile regressions?
Depending upon what kind problem you're trying solve and given other criteria like available resources some tasks might be best suited for other forms quantiles regressions like parametric quantiles regressions whereas others could ideally solved via weighted non parametric ones.
Final Words:
Weighted Non-parametric Quantile Regression has proven itself time and time again as an invaluable research tool in many aspects within science and business related fields. It allows researchers to take into account any irregularities within their datasets while still being able to accurately determine how these variables may interact with each other when looked at as a whole. WNQN is highly recommended for anyone looking for more accurate results from their research.