What does TNG mean in MATHEMATICS
TNG stands for Total Number of Gaussians and is a term used in statistics to describe the number of distinct Gaussian probability distributions used to model a set of data. It is particularly useful when dealing with univariate or multivariate datasets, as it helps to determine the best number of distinct probability distributions needed to adequately represent the data.
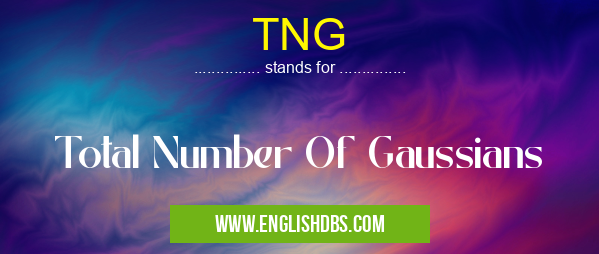
TNG meaning in Mathematics in Academic & Science
TNG mostly used in an acronym Mathematics in Category Academic & Science that means Total Number Of Gaussians
Shorthand: TNG,
Full Form: Total Number Of Gaussians
For more information of "Total Number Of Gaussians", see the section below.
Explanation
A Gaussian probability distribution is an important tool in statistics which describes a range of potential outcomes using a bell-shaped curve that has a mean, standard deviation, and variance. The total number of Gaussians (TNG) can be used to measure the amount of variation observed in a set of data by estimating how many different types of distributions are needed to best explain it. TNG indicates the complexity of the underlying probabilistic structure while also providing insights into the data's properties such as whether it contains outliers or clusters. Furthermore, TNG can be used as a criterion for model selection and provide evidence for overfitting or underfitting models when comparing various models fit to the same data.
Essential Questions and Answers on Total Number Of Gaussians in "SCIENCE»MATH"
What is Total Number Of Gaussians?
Total Number Of Gaussians (TNG) is a metric used to describe the mathematical shape of an image. It applies a Gaussian distribution to each pixel, and then sums those distributions to give an overall indication of the complexity of the image.
How is TNG used?
TNG is typically used in image processing applications to measure the complexity of an image or to compare different images with each other. This can be helpful in tasks such as texture classification, object recognition, and facial recognition.
What are some advantages of using TNG?
TNG provides a simple way to represent and compare images without needing large amounts of data or computing power, making it ideal for applications such as mobile phones. Additionally, it allows for comparisons between complex images that have vastly different sizes or resolutions.
Is there any downside to using TNG?
As with any metric, there are tradeoffs when using TNG; while it works well for comparing images with simple shapes or textures, it struggles when more intricate details are present. Additionally, since it relies heavily on pixel values and contrast levels, subtle differences in similar-looking images may not be reflected accurately.
In what ways can I use TNG?
TNG can be applied in many areas involving image analysis and comparison, such as facial recognition, texture detection/classification, object recognition/tracking, and content-based image retrieval. By understanding how these metrics work together to form an overall representation of an image's features, better decisions can be made about which images should be selected from a given set.
How does TNG compare to other metrics for measuring complexity?
Compared to metrics such as Shannon Entropy or Twin-Spiral Test results (which measure randomness rather than overall complexity), TNG provides a more detailed representation that takes into account both local features and global patterns within an image. Additionally, since it only uses the intensity values from each pixel within the image itself (and not any external data sources), it is useful for quickly comparing large collections of images without needing access to voluminous additional files.
Are there limitations when using TNG?
One potential limitation with using TNG is that depending on the size and resolution of the input images being compared, results may vary significantly; this means that if one wants consistent results across different datasets they may need to consider rescaling their inputs accordingly. Additionally, there is still work being done regarding incorporating nonimage characteristics into this measurement principle; while excellent progress has been made in recent years towards achieving this goal (such as developing algorithms utilizing motion capture videos alongside traditional static frames) certain corner cases may still cause issues when relying solely on information extracted from still photographs.
Does changing my camera settings affect how accurate my measurements will be?
Yes — having larger photos will generally result in more accurate measurements when using TNG because all pixels contribute equally regardless of their physical location within the frame; however optimizing your settings by considering various factors such as light levels & exposure time can help ensure you get the best possible results from your captures. Finally due note that increased compression ratios during capture/saving stages tend decrease accuracy since important details that convey texture & patterning may get lost during this process.
Final Words:
In conclusion, TNG stands for Total Number of Gaussians and is an important concept in statistics that provides insight into how complex a dataset is by estimating how many different types of probability distributions are required to adequately represent it. It can be used for model selection and identifying issues with overfitting or underfitting, making it an invaluable tool for working with both univariate and multivariate datasets.
TNG also stands for: |
|
All stands for TNG |