What does TFAR mean in IEEE
TFAR stands for Time-Frequency-Autoregressive. It is a mathematical model used in signal analysis and processing to model the behavior of a time series in frequency and time domains. TFAR models are used to characterize the relationships between components of a system such as temperature, pressure, or other physiologic signals in an optimal and objective fashion. They are also useful for detecting patterns of cyclic behavior that might otherwise be difficult to explain.
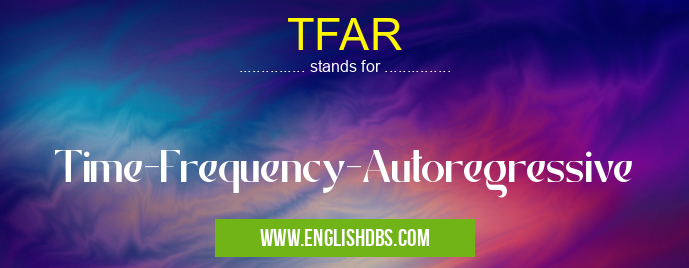
TFAR meaning in IEEE in Academic & Science
TFAR mostly used in an acronym IEEE in Category Academic & Science that means Time-Frequency-Autoregressive
Shorthand: TFAR,
Full Form: Time-Frequency-Autoregressive
For more information of "Time-Frequency-Autoregressive", see the section below.
Essential Questions and Answers on Time-Frequency-Autoregressive in "SCIENCE»IEEE"
What is Time-Frequency-Autoregressive (TFAR)?
Time-Frequency-Autoregressive (TFAR) is a statistical modeling technique used in the analysis of nonstationary signals. It combines autoregressive models with time-frequency representations of data to provide a more accurate description of signal behavior than other techniques. TFAR can be applied to areas such as bioengineering, telecommunications, and economics.
How does TFAR work?
TFAR works by analyzing a signal in terms of its frequency components which are then modeled using an autoregressive model. This model describes the evolution of the signal over time and allows for an improved understanding of how the signal changes as a function of time. The model also incorporates a frequency representation which captures key characteristics in the signal's behavior over multiple frequencies.
What types of signals can be analyzed with TFAR?
TFAR is particularly useful for nonstationary signals, which are signals that change their shape or behavior over time. These include medical and biological signals such as electrocardiograms, speech signals, audio recordings, large financial datasets, seismic data and others.
What advantages does TFAR have over other analysis techniques?
Compared to traditional methods such as Fourier transform analysis, TFAR offers several advantages including higher accuracy in describing varying signals behavior over different frequencies, increased capacity for detecting features in nonstationary systems, more efficient processing and faster computation times. Additionally it provides more informative graphical representations which can be used to gain insights into underlying processes that are not easily visible using traditional techniques.
Are there any limitations to using TFAR?
Although it offers many advantages compared to other analytical tools, certain limitations must be kept in mind when applying this technique. These include inadequate model capacity in some cases due to insufficient data points or noise levels too high for reliable modeling; poor scalability with increased complexity; memory constraints due to the large amounts of data; and computational complexity when dealing with very large datasets.
How can we reduce the limitations associated with implementing TFAR?
To reduce potential limitations related to implementing TFAR it is important to consider preprocessing steps such as denoising before beginning the modelling process itself. Additionally selecting appropriate parameters based on the type of data being analysed will ensure better performance while allowing for complete control over resulting models’ behaviour and facilitate comparison between different models if desired. Finally having enough computing resources available will help speed up processing times resulting from increased complexity.
What types of applications may use TFAR?
As previously mentioned several fields may benefit from using this technique including bioengineering – apoptosis detection or heart rate variability monitoring - telecommunications – speech recognition or likely interference sources identification - finance – predicting stock market trends or finding market anomalies - meteorology – short term forecasting or wind profiling studies - sound engineering – noise reduction or reverberation effects modelling amongst others.
What kinds of software programs are available for running analyses using TFAR?
Several software packages like GNU Octave , MATLAB , Python , R offer implementations suitable for carrying out analyses involving this technique alongside specific addons/libraries designed specifically for this purpose such as TFA toolbox (MATLAB), pylogit (Python) or RTFr (R). Other freeware options exist too like Sripflux Toolbox (MATLAB) which could also prove useful depending on individual needs.
Final Words:
Time-Frequency-Autoregression (TFAR) is a powerful tool for time series analysis and has been successfully applied to many different areas where rhythmic or periodic phenomena need to be studied or predicted accurately. It offers numerous advantages over traditional single frequency spectra approaches due it's ability to analyze various frequencies simultaneously along with factoring in previous values for more accurate predictions about future outcomes. The insights gained from this method can greatly improve our understanding of how certain systems or phenomena evolve over time within specific frequencies.