What does SSE mean in UNCLASSIFIED
SSE, short for Sums of Squares Error, is an important statistic that measures how well a model fits the data used as input. It is also known as the residual sum of squares (RSS) and indicates how much variation there is between the actual data points and the predicted values generated by the model. SSE can be used to compare different models to see which performs better in terms of accuracy. Generally, a model with a lower SSE is considered to be better fitted to the dataset.
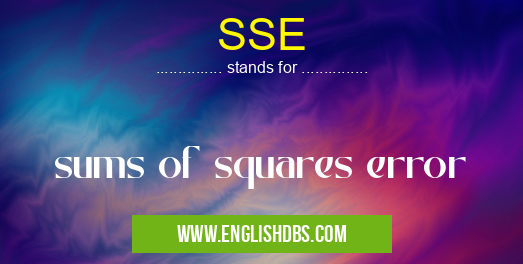
SSE meaning in Unclassified in Miscellaneous
SSE mostly used in an acronym Unclassified in Category Miscellaneous that means sums of squares error
Shorthand: SSE,
Full Form: sums of squares error
For more information of "sums of squares error", see the section below.
Definition
In statistics, SSE stands for Sums of Squares Error. It refers to a measure that reflects how well a model fits given data points. It is calculated by finding the square difference between each observed value and its corresponding predicted value from a given model. These differences are then cumulative added together resulting in an SSE value which quantifies deviation from expected values.
Application
An important application of SSE lies in regression analysis where it can be utilized to select the most appropriate regression equation among several competing models that best fits given data points. The lower the overall SSE value, the better fit of the equation and this helps researchers decide whether or not they should use that particular model to explain their findings or results from their experiments. Additionally, this statistic can further be utilized to test hypotheses and check if assumptions made during experiments hold true or not depending on what type of fitting equation was used.
Essential Questions and Answers on sums of squares error in "MISCELLANEOUS»UNFILED"
What is the meaning of Sums of Squares Error (SSE)?
Sums of Squares Error (SSE) refers to the measure of a residual error in a model. It is calculated by summing up all the squared differences between the observed values and the predicted values. SSE helps explain how well a model fits the data, so it can be used to compare different models or techniques.
How is Sums of Squares Error (SSE) calculated?
The calculation for SSE involves subtracting each observed value from its corresponding predicted value, squaring that result for each pair, and then summing across all of them. In other words, it is the sum of all squared differences between an observed value and its corresponding predicted value.
What are some common uses for Sums of Squares Error (SSE)?
SSE is commonly used in regression analysis as goodness-of-fit measures or to compare different models or techniques. It can also help decide whether more variables need to be included in the model or not. Additionally, SSE can be used to find out which independent variable has more explanatory power.
What does a low Sums of Squares Error (SSE) indicate?
A low SSE indicates that there are few discrepancies between your predictions and observations; i.e., that your model is a good fit for the data set given. This means that any further attempts at improvement would only yield marginal benefits, if at all.
What does a high Sums of Squares Error (SSE) indicate?
A high SSE indicates that there is room for improvement in your model because there are many discrepancies between your predictions and observations; i.e., your model does not fit well with the data given. This means you should attempt to improve upon your current model so as to decrease this discrepancy and bring it closer to zero.
Is there an efficient method to reduce Sums of Squares Error (SSE)?
Yes, there are several methods which can be used to reduce SSE such as incorporating more appropriate independent variables into your model, refining existing independent variables or transforming them into polynomial terms, simplifying your linear regression equation by removing collinearity etcetera.
How does one use Sums of Squares Error (SSE) for comparing different models?
When comparing two competing models based on their respective SSE values, higher SSE indicates that one model has greater discrepancies from observed values than another - this means lower SSE will indicate a better fitting/more accurate model than one with higher SSE.
Final Words:
In conclusion, Sums of Squares Error (SSE) provides an important statistic for statistical practitioners when trying to identify which statistical model will fit best with respect to given data points. This metric helps them evaluate various models based on their R-squared values (coefficient of determination). In general, when you select one model over another based solely on its smaller SSE value, it ensures that your prediction errors have been minimized thereby improving accuracy.
SSE also stands for: |
|
All stands for SSE |