What does BBMM mean in UNCLASSIFIED
Brownian bridge movement model (BBMM) is a statistical modeling technique used to simulate continuous random motions. It was first developed by mathematician Norbert Wiener and physicist Robert Lee Moffat in the 1950s. BBMM is used in various fields, such as economics, finance, marketing science, and engineering. Its purpose is to predict the evolution of a system's stochastic behavior over time. The term BBMM stands for Brownian Bridge Movement Model and it has become an important part of modern statistics.
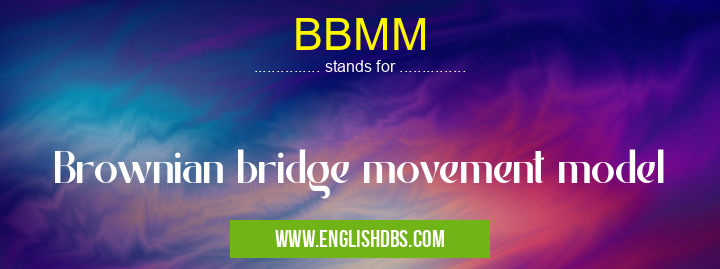
BBMM meaning in Unclassified in Miscellaneous
BBMM mostly used in an acronym Unclassified in Category Miscellaneous that means Brownian bridge movement model
Shorthand: BBMM,
Full Form: Brownian bridge movement model
For more information of "Brownian bridge movement model", see the section below.
Applications
BBMM is widely used across many domains and has practical applications in areas such as marketing science, economics, finance, engineering, medicine and robotics research among others. In economics for example the model can be applied to analyze inventory management issues or portfolio optimization strategies while in robotics it can be used to better understand motion trajectories or optimize control algorithms for robots’ movements based on their environment over time. Additionally, this type of model can be found utilized within medical research studies; specifically through assessing effects of drug treatments on patients over long periods of time through taking multiple readings over intervals rather than relying solely on single snapshots taken at one instant in time as well as tracking trends around patient health/symptomology at various stages during treatments etc..
Essential Questions and Answers on Brownian bridge movement model in "MISCELLANEOUS»UNFILED"
What is a Brownian Bridge Movement Model (BBMM)?
A Brownian Bridge Movement Model (BBMM) is a spatially explicit statistical model used to detect animal movement patterns and analyze the effects of environmental drivers on these movements. BBMM relies heavily on GPS and telemetry data, which it uses to reconstruct trajectories and then identify features such as turning angles or speed changes in the trajectory over space and time.
How does BBMM work?
The Brownian Bridge Movement Model works by using telemetry data to estimate an appropriate animal movement trajectory between two points. First, GPS coordinates are used to define two identifiable points: the starting point and the destination. Then, a Brownian bridge-like path is constructed that best fits these two locations. This model can also incorporate further constraints such as minimum distance traveled, which allows users to apply natural movement boundaries into the reconstruction process.
What are some applications of BBMM?
BBMM has many potential applications across different scientific disciplines including ecology, conservation biology, wildlife management, epidemiology, marine sciences, geography, and more. For example, it can be used to predict animal behavior in response to changing environmental conditions or assess how different populations interact with each other over space and time. In addition, this model can be used for habitat selection studies by quantifying environmental variables related to individual behaviors or population dynamics at larger spatial scales.
What are the advantages of using BBMM?
By providing an accurate representation of animal movement trajectories based on GPS/telemetry data analysis – as well as incorporating other constraints – Brownian Bridge Movement Models offer great accuracy in predicting spatial patterns over time compared with more simplistic models that rely only on geostatistical methods or least-cost path optimizations. Another advantage is that this model offers greater flexibility when it comes to incorporating specific constraints during model construction such as maximum speed or minimum distance traveled for example. As a result, BBMM offers an effective way of analyzing highly dynamic heterogeneous landscapes at multiple scales in order to gain insight into correlations between space occupancy characteristics and various factors driving animal movement patterns.
Is there software available specifically designed for BBMM?
Yes! There are several specialized software packages available that provide users with powerful tools for constructing and analyzing detailed Brownian bridge movement models based upon telemetry data collected from animals in their natural environment. These programs allow users to apply various filters/constraints when constructing their models while also providing options for visualizing results in maps and graphs for easy interpretation of results.
What type of data does BBMM require?
To effectively construct a proper brownian bridge movement model requires detailed GPS/telemetry data from moving animals over space and time along with any additional relevant information such as species identity, date/time stamps etc... It’s important that these datasets be consistent between individuals being tracked in order to properly analyze results.
Can I use BBMM on individual tracks or do I need multiple?
While it’s possible to construct a single track using this kind of analysis techniques – multiple tracks offer much greater flexibility when it comes to intended application purposes such as analyzing group movements across large landscapes or understanding habitat selection processes etc…
Final Words:
Overall Brownian Bridge Movement Model is an important technique in modern statisticians toolbelt - offering valuable insights into tracking changes over time for variably uncertain systems or processes when more traditional methods lack sufficient accuracy due to capturing too little data information points at any given instant to generate reliable predictions upon which decisions can then be made with confidence - therefore providing financial institutions, marketers etc., with better forecasts about potential outcomes before they occur under certain circumstances based off real-world historical information connected via 'bridges' so-to-speak that appear between data points.