What does PHDD mean in IEEE
Power Hybrid Decision Diagrams (PHDD) are a type of diagram used in artificial intelligence, software engineering, and logic programming to represent and manipulate Boolean functions. PHDDs combine both binary decision diagrams (BDDs) and algebraic decision diagrams (ADDs). They provide a powerful modeling language for solving complex problems.
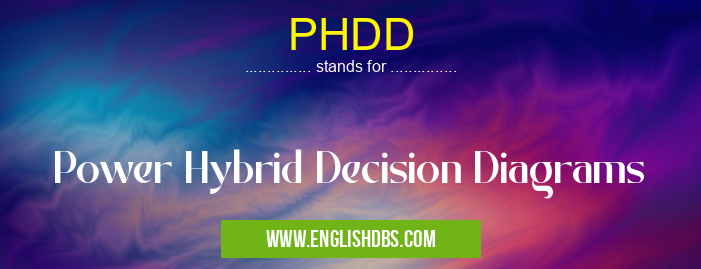
PHDD meaning in IEEE in Academic & Science
PHDD mostly used in an acronym IEEE in Category Academic & Science that means Power Hybrid Decision Diagrams
Shorthand: PHDD,
Full Form: Power Hybrid Decision Diagrams
For more information of "Power Hybrid Decision Diagrams", see the section below.
Essential Questions and Answers on Power Hybrid Decision Diagrams in "SCIENCE»IEEE"
What is a Power Hybrid Decision Diagram?
A Power Hybrid Decision Diagram (PHDD) is a type of diagram used in artificial intelligence, software engineering and logic programming to represent and manipulate Boolean functions. PHDDs utilize the features of both binary decision diagrams (BDDs) and algebraic decision diagrams (ADDs).
Why are Power Hybrid Decision Diagrams useful?
PHDDs are used for simplifying logical expressions or solving complex problems. They enable efficient manipulation of variables with large domains, as well as providing an effective graphical representation of the problem's solution. Additionally, they can be used to visualize the effects of changes on a system over time.
How do you create a Power Hybrid Decision Diagram?
To create a Power Hybrid Decision Diagram, the user must first create the nodes and edges associated with each element in the problem. Each node should represent an individual operation or state while each edge should represent how these components interact with each other. Once all these elements have been defined, the user can then construct their PHDD to visualize and solve their problem.
Final Words:
In conclusion, Power Hybrid Decision Diagrams are an excellent tool for solving complex problems or simplifying logical expressions. They effectively combine both BDDs and ADDs to provide an efficient modeling language that can accurately represent state changes over time. With PHDDs, users can easily construct representations of their systems by creating nodes and edges that associate with each component in them.