What does NRMH mean in UNCLASSIFIED
Non-reversible Metropolis-Hastings (NRMH) is an alternate approach to the classical Metropolis-Hastings algorithm. It is an iterative and stochastic simulation method for sampling from a probability distribution, with applications in various fields such as probability theory, statistics, science, engineering and other areas. NRMH has been used to construct Markov chain Monte Carlo (MCMC) methods, which are iterative algorithms that generate sample sequences with key properties of the target distribution by randomly transitioning from one state to another in a Markov chain.
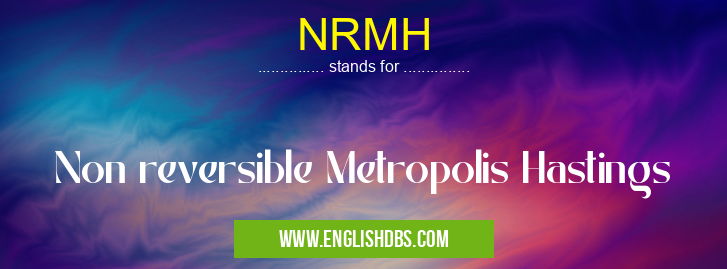
NRMH meaning in Unclassified in Miscellaneous
NRMH mostly used in an acronym Unclassified in Category Miscellaneous that means Non reversible Metropolis Hastings
Shorthand: NRMH,
Full Form: Non reversible Metropolis Hastings
For more information of "Non reversible Metropolis Hastings", see the section below.
Key Features
The main features of NRMH are that it can be tuned differently for different problems; it allows users to arbitrarily control their proposals; it can handle high dimensionality quite well; and it reduces dependence on irreversible steps while still producing good results in sampling complicated distributions. The performance of NRMH depends significantly on how well users tune its parameters, so its output may vary depending on the application or problem being solved. Additionally, compared to other MCMC methods like Gibbs sampling or Hamiltonian Monte Carlo, NRMH tends to be more computationally expensive due to its greater reliance on optimisation techniques and need for tuning parameters.
Advantages & Disadvantages
The main advantage of NRMH is its flexibility - it can be applied widely across different areas where other MCMC methods might fail due to assumptions about distributions or difficulty in gaining sufficient sample size within an acceptable amount of time. However, there are also several disadvantages associated with NRMH - since it uses multiple proposals at once rather than just one at each step, this increases computational complexity significantly; since parameters must be tuned properly for optimal performance, there is potential for user error; and there is always some risk associated with not knowing whether or not the proposal will succeed.
Essential Questions and Answers on Non reversible Metropolis Hastings in "MISCELLANEOUS»UNFILED"
What is Non Reversible Metropolis Hastings?
Non Reversible Metropolis Hastings (NRMH) is a type of Markov Chain Monte Carlo (MCMC) algorithm which combines the traditional Metropolis-Hastings method with ideas from Hamiltonian mechanics in order to facilitate efficient sampling from complicated probability distributions. NRMH utilizes a “Hamiltonian dynamics” approach rather than the standard random number generator based selection employed by traditional MCMC techniques. The result is an improved ability to explore complicated target distributions, as compared to straight Metropolis-Hastings.
How does NRMH differ from other MCMC techniques?
Unlike other MCMC algorithms which rely on random number generators to select proposed moves, NRMH instead uses Hamiltonian dynamics or Hamiltonian Monte Carlo (HMC) techniques to generate proposals. HMC utilizes momentum and energy variables as it evolves towards a more probable state and has been shown to be more reliable for exploring complicated target distributions than the classic Metropolis-Hastings algorithm alone.
Is NRMH easy to use?
Yes, implementations of NRMH in popular programming languages such as Python are widely available and relatively straightforward to use. Developers interested in exploring this technique further can find helpful tutorials in most programming language communities.
What applications can benefit from using NRMH?
Many applications can benefit from employing the power of Non Reversible Metropolis-Hastings algorithms including Bayesian inference, natural language processing tasks involving latent variables modeling, and clustering problems that require sampling from multidimensional distributions with strong correlations among components. Furthermore, these improvements have been demonstrated in a variety of different scenarios ranging from simple flame simulations such as diffusion limited aggregation models all the way up through complex probabilistic graphical models such as Bayesian networks.
Can I modify NRMH for my own project?
Yes! In fact, many researchers have modified non reversible Metropolis-Hastings algorithms specifically for their own projects such as probabilistic graphical model inference and reinforcement learning tasks requiring exploration of large state spaces. These modified algorithms often combine elements of both traditional Metropolis-Hastings and Hamiltonian mechanics in order to add additional sophistication to the learning process while still ensuring that samples drawn are accurate representations of the underlying distribution being explored.
Is there any research which supports non reversible metropolised hasting algorithm?
Yes, there is an increasing amount of research which suggests that non reversible metropolised hasting algorithms are superior compared to standard MCMC approaches when exploring complex target distributions due to their incorporation of energy terms and momentum variables which act as guides during exploration. This research has been published both independently by academic institutions and by organizations specializing in machine learning development such as Google AI Research Labs who recently released results suggesting improved performance when using an adapted version incorporating particle swarm optimization techniques alongside NRMH sampling strategies.
Final Words:
Non reversible Metropolis Hastings (NRMH) offers great flexibility compared to other MCMC methods when dealing with complex distributions or large datasets that require extensive exploration due to its ability to combine multiple proposals in one step without sacrificing accuracy or introducing additional assumptions about underlying distributions. While cautious tuning of parameters may be required in order ensure optimal performance from the algorithm and a certain degree of risk associated with non-reversibility exists, these drawbacks can be minimized given suitable implementational knowledge and understanding of the particular application at hand.