What does NOLH mean in UNCLASSIFIED
Nearly orthogonal Latin hypercubes (NOLH) are a type of design used to conduct tests that generate a set of points with multiple dimensions in order to explore the response surface. A NOLH is derived from the concept of a Latin Hypercube, but instead of sampling points randomly, they are designed to maximize the relative distance between points in high dimensional spaces. By doing so, this allows for efficient and accurate statistical analysis when measuring the effects of various inputs on output responses.
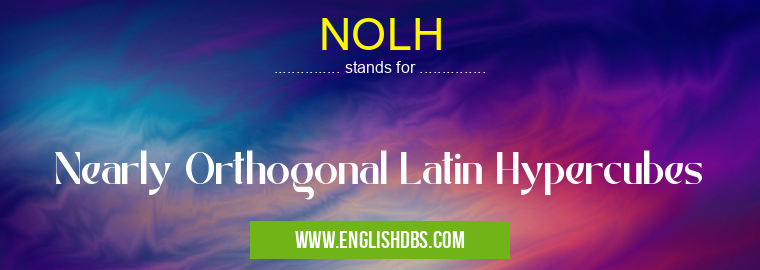
NOLH meaning in Unclassified in Miscellaneous
NOLH mostly used in an acronym Unclassified in Category Miscellaneous that means Nearly Orthogonal Latin Hypercubes
Shorthand: NOLH,
Full Form: Nearly Orthogonal Latin Hypercubes
For more information of "Nearly Orthogonal Latin Hypercubes", see the section below.
Essential Questions and Answers on Nearly Orthogonal Latin Hypercubes in "MISCELLANEOUS»UNFILED"
What are nearly orthogonal Latin hypercubes?
What is the main goal in using a NOLH?
The main goal when using a NOLH is to minimize correlation and optimize patterns of distribution between each point in multiple dimensions. This helps ensure that there are no bias or “clustering” within any one area of interest. With this type of design, one can gain a detailed understanding into how various input factors can affect an output response while reducing computational time and cost.
How do you construct a NOLH?
To construct a NOLH, start by taking an existing Latin Hypercube and modifying it in order to maximize the relative distance between each point over all dimensions. This process involves rearranging variables within each dimension such that they form nearly orthogonal blocks across all factors, creating more evenly spaced and distinct samples than what you would have through random sampling alone.
What are some advantages associated with using NOLHs?
Due to their construction method, NOLHS offer several advantages over standard Latin Hypercubes. These include increased accuracy due to better representation of data points across different variables, improved precision when testing for correlations between variables, reduced run-time for simulations due to fewer required replicates per factor level as well as fewer total runs needed overall, and greater efficiency due to reduced computational resources required.
Are there any drawbacks associated with using a Nearly Orthogonal Latin Hypercube?
Although there are many advantages associated with using this type of design, there may be some drawbacks related to its construction depending on certain scenarios. The trade-off for achieving higher orthogonality could result in unevenly distributed sample sizes which could potentially lead to biased results if not accounted for properly prior to beginning your experiment or simulation. In addition, since it relies heavily on algorithms for optimization purposes, incorrect implementation or modifications made during experimentation could also lead faulty results if not done properly.
How many replication/run is typically enough when conducting experiments using Nearly Orthogonal Latin Hypercubes?
The number of replications/runs needed depends heavily on the size/scope your experiment as well as your desired level accuracy and confidence intervals you wish achieve with your results; however typically no more than ten replicates per factor level should suffice when constructing your NOLH structure. Furthermore having too many runs can thus adversely affect accuracy as too much repetition can induce unnecessary noise into your experiment which will skew results.
Are there certain situations where using a Standard Latin Hypercube (SLH) might be more suitable than creating an NLOH structure?
Yes; SLH’s can still be used depending upon objectives that need addressed during experimentation such as modeling nonlinear responses or obtaining specific variable levels combinations within replications etc... Also SLHS may be preferred if computation times are limited or if instrumentation does not allow efficient replication.
What are some tips you should consider before conducting experiments with NLOHs?
Before conducting experiments with NLOHs it is important plan ahead and assess which factor levels need addressed first so that optimal distributions plots can be achieved prior to actual experimentation; it's also important make sure proper randomization methods are implemented if necessary when replicating samples along different factor levels otherwise bias will occur bring inaccurate results . Furthermore always check data structures prior running simulations in order to confirm correctnesses – mistakes done at this stage cannot be reversed post run time!
: Are there any tools available help create , modify , or view designs generated by NLOH structures?
: Yes ; variety software programs exist today assist researchers model simulate , analyze ,and view models built off existing NLOH structures . Such programs include DOE++ , Ninja Design Studio -- both freely open source -- as well commercial offline solutions Lonix Design Manager . Each these provides unique features users explore further construction variability /modification capabilities offered by these types designs.