What does AAUC mean in MATHEMATICS
The abbreviation AUC (Area Under the Curve) is an indicator of how well a system, model or algorithm performs in making accurate predictions. It is used to measure the accuracy of a model by plotting the observed class labels (true positives and true negatives) against the predicted probabilities for different classes, and then calculating the area under this curve. The more accurate the model, the larger the area under the curve will be. AUC gives us an indication of how well our model performs on unseen data. This means that it is one way to measure a model's ability to generalize from training data to unseen test data.
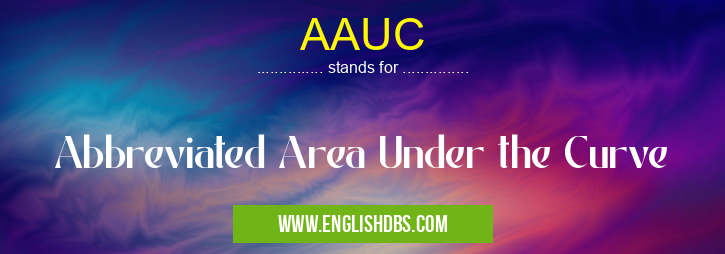
AAUC meaning in Mathematics in Academic & Science
AAUC mostly used in an acronym Mathematics in Category Academic & Science that means Abbreviated Area Under the Curve
Shorthand: AAUC,
Full Form: Abbreviated Area Under the Curve
For more information of "Abbreviated Area Under the Curve", see the section below.
What Is AUC
AUC (Abbreviated Area Under the Curve) is often used when making predictive models, such as those used in machine learning and artificial intelligence applications. The AUC measures how well a system or algorithm can correctly classify values into two categories by plotting actual values against predicted probabilities for each category. The higher the number, the better performance of the system or algorithm; meaning it is capable of accurately predicting which class a value belongs to with greater accuracy when compared with other systems/algorithms that may have been tested against it.
Essential Questions and Answers on Abbreviated Area Under the Curve in "SCIENCE»MATH"
What is an AUC?
AUC is an acronym for Area Under the Curve. It is a measure of how well a model can discriminate between two classes, usually "positive" and "negative". The closer to 1 the AUC is, the better the model can accurately differentiate between the two classes.
What does abbreciated AUC mean?
Abbreviated AUC (AUCA) refers to a measure of performance that only partially evaluates the ability of a model to differentiate between positive and negative classes. Instead of evaluating all of the values along a curve, AUCA looks at only a subset, or “abbreviation†of them.
When is AUCA used?
AUCA is typically used when time or resources are limited. Because it evaluates only a subset of values along a curve, it can provide an understanding of how well a model performs in less time than other methods such as calculating the complete Area Under the Curve (AUC).
How do you calculate AUCA?
AUCA is calculated by measuring how much area is under a given portion of the curve from 0 to 1 on both axes. This can be done using numerical integration formulas or by manually counting rectangles along those portions of the curve.
Can I use AUCA for more than two classes?
No, AUCA is designed for models with only two classes — “positive†and “negative†— and cannot be extended to include more than two categories. To evaluate models with more than two possible outcomes, another method such as ROC analysis may be more appropriate.
What tool do I use to calculate AUCA?
There are many statistical software packages available that allow you to calculate AUCA quickly and easily. These include R (free open source), Python (open source), MATLAB (commercial software), SAS (commercial software), SPSS (commercial software), Stata (commercial software), etc.
Does my model have to be linear for me to use AUCA?
No, nonlinear models can also benefit from calculating an abbreviated area under the curve as long as they have only two classes present in their data set. Most statistical programs offer multiple algorithms that are suitable for different types of data sets and models.
Final Words:
Knowing what AUC (Abbreviated Area Under the Curve) stands for can help you interpret results from your predictive models more accurately and allow you to make adjustments where necessary in order to improve their performance when tested on unseen data. Having a good understanding of this acronym will also enable you to interpret your results correctly when using machine learning and artificial intelligence algorithms so that you can make informed decisions about their potential effectiveness for your business objectives.
AAUC also stands for: |
|
All stands for AAUC |