What does MNBE mean in UNCLASSIFIED
MMNE stands for Mean Normalized Bias Error. This concept is typically used in data science, artificial intelligence and machine learning contexts, where it is applied to analyze and optimize the accuracy, performance and results of predictive models.
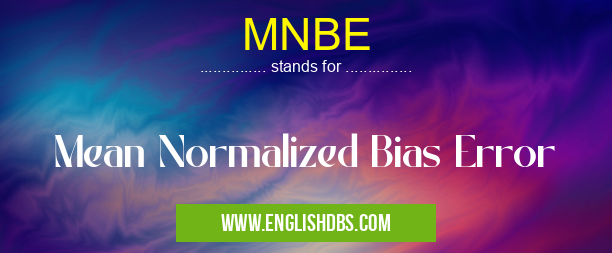
MNBE meaning in Unclassified in Miscellaneous
MNBE mostly used in an acronym Unclassified in Category Miscellaneous that means Mean Normalized Bias Error
Shorthand: MNBE,
Full Form: Mean Normalized Bias Error
For more information of "Mean Normalized Bias Error", see the section below.
Essential Questions and Answers on Mean Normalized Bias Error in "MISCELLANEOUS»UNFILED"
What is a Mean Normalized Bias Error?
A Mean Normalized Bias Error (MMNE) is an evaluation process that measures the difference between true values and predicted values in a predictive model, usually expressed as a percentage. It helps identify areas for improvement in a machine learning algorithm or other type of predictive model.
How is a Mean Normalized Bias Error calculated?
MMNE calculations measure the bias of prediction differences by comparing absolute errors with normalizing constants such as the mean or standard deviation of all prediction errors within a given dataset. Errors are then summed up and divided by the total number of predictions.
What is an acceptable level of precision for a Mean Normalized Bias Error?
An acceptable precision level will vary depending on the nature of the project, but generally speaking an error rate below 10% can be considered good enough for most practical applications. However, some industry standards may require higher levels of precision depending on their requirements.
What are some strategies to reduce Mean Normalized Bias Errors?
Strategies that can be used to reduce MMNE include increasing training data quantity, checking data quality and relevance, analyzing hyperparameters like learning rate or regularization techniques to optimize model performance, as well as using techniques like cross-validation for further testing purposes.
Final Words:
By understanding what MMNE stands for and how it works, data scientists can improve their predictive models by lowering their bias errors through various methods discussed above. This will result in more reliable models which can provide greater insights into data patterns without introduction significant levels of error into the results.