What does MLLM mean in LANGUAGE & LITERATURE
Machine Learning for Language Modelling (MLLM) is a branch of Artificial Intelligence that focuses on training machines to understand and generate natural language sentences. It uses machine learning algorithms to create statistical models of language, in order to help computers understand the meaning of text. Through the use of data, MLLM enables computers to recognize patterns in text, identify relationships between words, and create meaningful statements from large volumes of textual data.
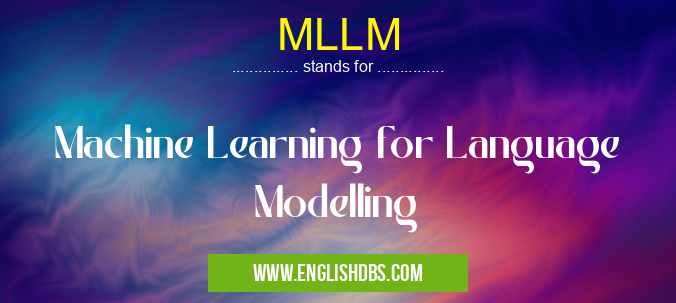
MLLM meaning in Language & Literature in Academic & Science
MLLM mostly used in an acronym Language & Literature in Category Academic & Science that means Machine Learning for Language Modelling
Shorthand: MLLM,
Full Form: Machine Learning for Language Modelling
For more information of "Machine Learning for Language Modelling", see the section below.
Process
At its core, MLLM involves training a machine learning algorithm with large amounts of data in order to build a model capable of generating meaningful phrases and sentences. To do this, MLLM begins by looking at sentence-level structures such as grammar and syntax. By analyzing how words are used within sentences, MLLM can learn how words are related and the context in which they are used. From here it identifies which words tend to occur together more often than others. This knowledge is then applied to generate new sentences that more accurately reflect the same meanings. The next step involves capturing the nuance and style of language through an understanding of word perspectives such as positive or negative connotations or topical relevance. With this deeper level of understanding, MLLM is able to produce more accurate results beyond simply recognizing patterns in text. Lastly, sentiment analysis takes into account people's emotions when processing language models which allows machines to provide meaningful response based on user sentiment towards certain topics or phrases.
Benefits
The benefits of using MLLM extend beyond simply producing accurate language models; it also has applications in various fields such as customer service and medical diagnosis where accuracy is especially important for success. In customer service for example, MLLM can be used to train automated chatbots. These bots could respond intelligently to customer inquiries using natural language processing techniques learned from data gathered by human interactions with customers over time.
Essential Questions and Answers on Machine Learning for Language Modelling in "SCIENCE»LITERATURE"
What is Machine Learning for Language Modelling?
Machine Learning for Language Modelling (MLLM) is a type of artificial intelligence (AI) application that uses language data to teach machines how to learn, recognize, and use language. It uses natural language processing (NLP) techniques to create algorithms that can recognize patterns in language input and then generate appropriate responses. MLLM helps machines understand the nuances of human languages, making it easier for them to interact with humans in a more natural way.
How does MLLM work?
MLLM uses natural language processing algorithms to analyze text data and identify patterns within it. Once the algorithm has identified patterns, it can use this information to predict what will likely come next in a sentence or an entire document. The goal of MLLM is to help machines identify these patterns so they can generate better results when asked questions or given tasks related to language understanding.
What are some applications of MLLM?
MLLM has numerous potential applications ranging from automated customer service agents and chatbots, sentiment analysis services, search engine optimization tools, question-answering services, voice recognition systems, natural language interfaces for machine learning systems, among many others.
What are some common techniques used in MLLM?
Common techniques used in MLLM include neural networks such as long short-term memory (LSTMs), convolutional neural networks (CNNs), recurrent neural networks (RNNs), attention models, named entity recognition (NER), sentiment analysis and semantic analysis.
What types of data typically used for training an MLLM algorithm?
Most commonly used data sources for training an MLLM algorithm include natural language databases such as Wikipedia articles or books; audio files such as dialogues between people; videos with subtitles; and webpages containing written text. Other datasets may also be used depending on the specific task at hand.
Is there any easy way to create an MLLM model?
Yes! There are several frameworks available that allow developers to quickly set up and train their own ML/LM models with little effort. Popular frameworks include TensorFlow by Google; PyTorch by Facebook; Scikit Learn by Python; Flair by Zalando Research; and BERT from Google AI Research Team. Additionally there are several pre-trained models publicly available that developers can download & fine tune for their own use cases.
What evaluation metrics are used for measuring the performance of an ML/LM model?
Common evaluation metrics used when measuring the performance of ML/LM models include accuracy (how often does the model provide the correct output?), precision & recall scores (how well does it capture true positives & false negatives?) and perplexity scores (measures how well the model predicts new unseen words). Additionally qualitative methods such as manual inspection or qualitative surveys may be employed depending on the specific scenario being evaluated.
Are there any best practices I should follow when creating my own ML/LM model?
Yes! Always start off by gathering sufficient training data that is representative of your desired outcomes followed by feature engineering & selection based on domain knowledge - this will ensure your model has enough variation & complexity which will generally lead to improved accuracy scores later down the line during testing stage. Further if developing a deep learning based approach always consider applying regularization techniques during training phase e.g dropout layers which greatly reduce overfitting while allowing greater flexibility in designing complex architectures required for complex tasks such as Natural Language Processing.
Final Words:
Overall, Machine Learning for Language Modelling (MLLM) offers a wide range of potential applications across many different industries due its ability to analyze vast amounts of data quickly and accurately while providing insights related to subtle nuances contained within textual data that may not be easily noticeable through traditional analysis methods. As technology continues to advance rapidly so too will our understanding of these powerful tools providing us with increasingly improved methods for interpreting and generating language.