What does MLG mean in CHEMISTRY
MLG is an acronym that stands for Maximum Likelihood Goodness. It is a measure of the ability of a statistical model to explain the observed data accurately and efficiently. MLG measures how well the model fits the data, which can then be used to assess whether or not it is appropriate for use in research studies or other applications. It is an important statistic to consider when selecting a model for use in data analysis.
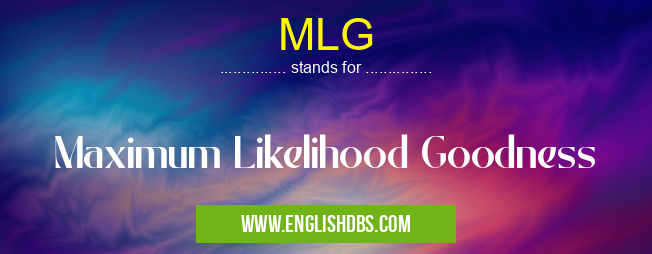
MLG meaning in Chemistry in Academic & Science
MLG mostly used in an acronym Chemistry in Category Academic & Science that means Maximum Likelihood Goodness
Shorthand: MLG,
Full Form: Maximum Likelihood Goodness
For more information of "Maximum Likelihood Goodness", see the section below.
Definition
Maximum Likelihood Goodness (MLG) measures the accuracy of a particular statistical model by calculating how well it fits the observed data given its assumptions. The MLG score is used to determine how well the model explains or predicts what we observe in real life. A higher MLG score indicates a better fitting model and vice versa.
Methods
The calculation of MLG involves several steps and components, namely, estimating parameters from observed data, evaluating goodness-of-fit statistics, and determining parameter adequacy (such as confidence intervals). The method also includes 3 essential components; namely selection criteria, hypothesis testing, and optimization procedures. In selection criteria, the best fit models are selected based on their log-likelihood scores; hypothesis testing helps determine if the explanatory variables have significant impact on results; finally optimization procedures make sure that no relevant explanatory variable has been omitted from consideration.
Essential Questions and Answers on Maximum Likelihood Goodness in "SCIENCE»CHEMISTRY"
What is Maximum Likelihood Goodness (MLG)?
Maximum Likelihood Goodness (MLG) is a mathematical method used to derive the probability of an event, given some known data. It relies on the notion that, in any set of data, there exists a model that best explains the phenomenon observed. MLG works by maximizing the likelihood of this model given what is known about the data.
What types of situations can MLG be used for?
MLG can be applied to a variety of data sets and scenarios. For example, it can be used to determine whether or not a given hypothesis is true based on some existing evidence, or to discriminate between competing models when trying to explain some observed phenomenon.
Is MLG always the most appropriate tool?
Not necessarily; it depends on the type of problem you're trying to solve. If your goal is simply to estimate parameters in a model, then another technique may make more sense than MLG. However, when trying to understand which model best explains an observation, or if you need to compare multiple different models, then MLG may be more suitable due to its ability to assess how well different models fit the data.
How does one calculate MLB?
Calculating MLG involves evaluating several equations that sum up all possible observed outcomes according to each model being tested. The equation will yield a value indicating how probable each outcome is under each model. This value can then be compared across different models and hypotheses in order to identify which one best explains what was observed in the data set.
Are there any assumptions involved with using MLG?
Yes; when performing calculations using MLG techniques, certain assumptions must be taken into account such as assumptions regarding independence and distributions among parameters. Also, depending on the nature of the problem being solved, it may be necessary to impose additional constraints on the parameter values before calculating goodness-of-fit values.
Does MLG work with small sample sizes?
Yes; while large sample sizes are helpful as they provide more reliable results due to improved precision, MLG still works just fine with smaller datasets as long as they contain enough information for accurate estimation of probabilities.
What are some potential drawbacks associated with using MLB?
One potential drawback with MLS is that it assumes that all variables within a given dataset are independent from one another — meaning that changes in one variable do not affect changes in another variable — which could lead to inaccurate results if certain interactions between variables exist but go unnoticed. Additionally, determining which specific elements control performance can sometimes become tedious and time consuming
How does MLG differ from other statistical approaches?
Unlike other statistical approaches such as maximum likelihood estimation (MLE), MLG does not require any prior knowledge about the underlying distribution being studied; rather it looks at all available data points simultaneously and makes inferences based solely on what has been observed.
How accurate are Maximum Likelihood Goodness calculations typically?
Due accuracy depends heavily on proper implementation factors such as correctly predicting all sources of variability present within a given dataset and choosing meaningful parameters for comparison; however when applied correctly MLG typically yields highly reliable results.
Final Words:
MLG is an important measure when selecting a statistical model for use in research studies or other applications as it helps identify models with higher accuracy levels desirable for valid conclusions. Due to its wide applicability across various domains such as medicine and economics, scholars rely on MLG coupled with other related statistics like Akaike Information Criterion (AIC) or Bayesian Information Criterion (BIC) to select appropriate models.