What does MFCC mean in UNCLASSIFIED
Mel Frequency Cepstral Coefficients (MFCC) is a feature extraction technique commonly used in the field of audio processing. It extracts an acoustic representation of a sound that can be used for various applications such as speech recognition, music classification and others. MFCCs are widely used and considered to be one of the most important tools for extracting relevant information from sounds.
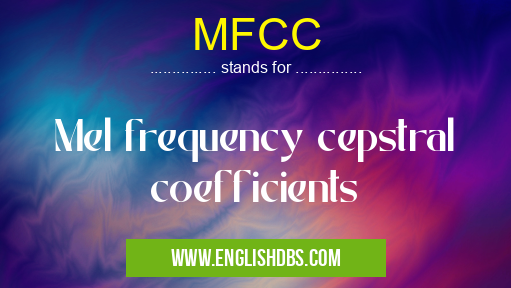
MFCC meaning in Unclassified in Miscellaneous
MFCC mostly used in an acronym Unclassified in Category Miscellaneous that means Mel frequency cepstral coefficients
Shorthand: MFCC,
Full Form: Mel frequency cepstral coefficients
For more information of "Mel frequency cepstral coefficients", see the section below.
Applications Of MFCC
As mentioned above,MFCCs have several practical applications when it comes to audio processing and analysis. One common use case is with automatic speech recognition systems which use this technique to analyse and recognise spoken words using computational models trained on data sets containing labelled examples of voice recordings labelled by word type. Another application of MFCC includes music genre classification, where this technique helps identify certain characteristics within different genres which create unique signatures making them distinguishable from each other. Additionally, MFCCs can also be used for audio source separation - a task which involves separates two or more signals applied to the same microphone input or recording — as well as speech synthesis and tonal detection in music files.
Essential Questions and Answers on Mel frequency cepstral coefficients in "MISCELLANEOUS»UNFILED"
What are Mel Frequency Cepstral Coefficients?
Mel Frequency Cepstral Coefficients (MFCC) are a set of coefficients commonly used in speech recognition systems. They capture important characteristics of speech and help in distinguishing between different sounds. The coefficients are derived from the power spectrum of a sound by taking the logarithm and then applying the discrete cosine transform (DCT). By doing so, MFCCs identify certain frequency areas that have importance for speech recognition.
What is the purpose of using MFCCs?
MFCCs are widely used to represent acoustic features because they enable computers to identify complex patterns in audio that otherwise would be difficult or impossible with other methods. Using MFCCs, computers can accurately recognize human speech and distinguish it from other types of noises. This makes them very useful for many applications such as automatic language translation, speaker identification and automatic music transcription among others.
How are MFCCs calculated?
The calculation process starts by transforming an audio signal into a spectrogram which represents its frequency content across time. The various sound amplitudes represented by this spectrogram are then filtered and combined into Frequency Bands common to human perception of sound. Finally, those frequencies bands undergoes a Discrete Cosine Transform which produces the final set of coefficients known as the MFCCs.
Why do we use logarithms in computing MFCCs?
Logarithms play an important role in computing MFCCs since they compress large ranges of amplitude values into a smaller range more suitable for analysis by computer systems. This compression also allows us to focus on specific regions of audibility, thus enhancing our ability to detect subtle differences between sounds that would otherwise be lost due ot the relatively large range required when dealing with without logarithmic compression.
What type of data does an MFCC represent?
An MFCC represents information about an audio signal such as its frequency content, amplitude and how these change over time but does so within certain limitations due to its nature as a mathematical representation rather than containing all information from the original signal itself.
What advantage does using an array of values provide?
Arrays provide the advantage of representing a wide variety of data quickly and efficiently which is especially useful when dealing with complex problems like recognizing human speech or distinguishing between different types of noise.
Can we use other transforms instead of DCT for computing MFCCs?
In principle yes, any transform capable producing coefficients that retain useful information about the original signal could be used to compute MFCCs although DCT remains one of the most popularly used options due to its favorable computational complexity.
Final Words:
In conclusion, Mel Frequency Cepstral Coefficients (MFCC) is an effective feature extraction procedure that can help extract relevant information from sound signals for many different tasks such as automatic speech recognition, music genre classification and much more. This procedure works by mapping linear frequencies into logarithmic scales known as mel scales, followed by applying Discrete Cosine Transform (DCT) to generate cepstrums representing properties within sound signals in terms time and frequency domain.
MFCC also stands for: |
|
All stands for MFCC |