What does MCGA mean in HUMAN GENOME
Monte Carlo Genetic Algorithm (MCGA) is an advanced evolutionary approach that combines principles from both Monte Carlo and genetic algorithms to solve complex optimization problems. MCGA is a probabilistic search technique modeled after the evolutionary cycle of life, and utilizes both stochastic processes as well as heuristic methods to optimize a given problem. At its core, MCGA can be thought of as a two-step optimization process where random samples are first generated by Monte Carlo simulations, and then a genetic algorithm is applied on these samples to identify the most optimal solution in terms of meeting predefined criteria.
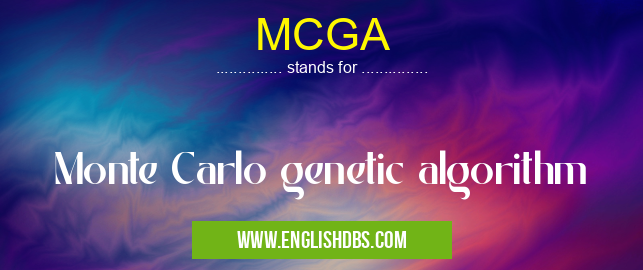
MCGA meaning in Human Genome in Medical
MCGA mostly used in an acronym Human Genome in Category Medical that means Monte Carlo genetic algorithm
Shorthand: MCGA,
Full Form: Monte Carlo genetic algorithm
For more information of "Monte Carlo genetic algorithm", see the section below.
» Medical » Human Genome
Essential Questions and Answers on Monte Carlo genetic algorithm in "MEDICAL»GENOME"
What is a Monte Carlo Genetic Algorithm (MCGA)?
A Monte Carlo genetic algorithm (MCGA) is an evolutionary algorithm that uses probabilistic techniques to search for a suitable solution in large, complex problem spaces. In this technique, randomness is incorporated into the search process to discover new solutions and to identify which of these are potentially better than existing solutions. MCGAs have been successfully applied to problems such as designing robots, scheduling jobs, optimizing airplane routes, and creating computer images.
How does a Monte Carlo Genetic Algorithm work?
An MCGA works by simulating natural selection on a population of random solutions. Through mutations, crossover operations, and other procedures based on selection pressure, the best solutions tend to survive while weaker ones are discarded. As the algorithm progresses over successive generations of solutions, it slowly converges towards an optimal solution based on the user-defined criteria.
How is an MCGA different from other optimization algorithms?
Unlike other optimization algorithms such as gradient-based methods or simulated annealing that focus on refining existing solutions via local search operations, MCGAs focus on exploring new regions of solution space using probabilistic methods. This approach makes them well suited for solving complex problems with multiple objectives or with unknown constraints or discontinuities.
What factors should be considered when using an MCGA?
When using an MCGA for problem solving, it's important to consider several factors including population size; mutation rate; crossover rate; selection intensity; and stopping criteria such as number of iterations or target value. These parameters can influence the results significantly and must be chosen carefully depending on the specific problem at hand.
What are some examples of applications where MCGAs are used?
Monte Carlo genetic algorithms have been successfully used in a variety of applications including robotics design, job scheduling, airline route optimization and image processing. For example, they can be used by roboticists to design more natural-looking robots while considering various physical constraints such as weight and joint limits. Similarly they can also be used in network optimization tasks such as airport flight scheduling or airline route planning.
How long does it take for an MCGA to solve a problem?
The running time for an MCGA will vary depending on several factors such as population size, mutation rate and selection intensity etc., but typically it takes around 10-100 iterations before convergence begins to occur. After this initial period the algorithm tends to reach convergence rapidly if all parameters have been chosen appropriately.
What are some challenges associated with using Monte Carlo genetic algorithms?
One of the main challenges associated with using Monte Carlos genetic algorithms is ensuring there's sufficient diversity within the population of possible solutions during each iteration so that new potential optimal candidates can emerge without affecting earlier progressions too much. It's also important to ensure appropriate selection pressure is applied to guide mutations and crossovers towards more promising regions in solution space lest the process become stuck in local optima too early.
Is there any way I can speed up the convergence process?
Yes - one common approach is increasing mutation rate slightly higher than what would normally be accepted when using conventional genetic algorithms since this gives more opportunities for discoveries but may require extra computing resources or time depending on the complexity of your problem space.
Are Monte Carlo genetic algorithms always necessary?
Not necessarily; depending on your particular use case you might need certain features that could only be achieved through applying probabilistic methods like ones used by Monte Carlo GAs but otherwise simpler approaches like hill climbing may suffice in many instances.
Final Words:
In summary, Monte Carlo Genetic Algorithm (MCGA) is an intelligent optimization tool that marries the capabilities of both Monte Carlo simulation and Genetics algorithms in order to find near-optimal solutions for difficult problems in an efficient manner. By utilizing local search heuristics rather than relying solely on crossover and mutation, MCGAs can afford greater flexibility when dealing with unknown and uncertain systems than traditional genetic algorithms can provide. As such, this evolutionary computing tool has become increasingly popular for tackling many complicated real-world problems.
MCGA also stands for: |
|
All stands for MCGA |