What does LKDE mean in UNCLASSIFIED
Localized Kernel Density Estimation (LKDE) is a type of nonparametric statistical technique. It relies on a kernel-based approach to estimate the probability density function of a given dataset. Unlike parametric methods, which use predetermined formulas and assumptions about the data distribution, LKDE does not make any underlying assumptions about how the data is distributed. Instead, it uses individual data points as well as their local neighborhood to calculate the probability density estimates for each point. In this way, it gives more accurate estimates than parametric methods, resulting in better predictive performance.
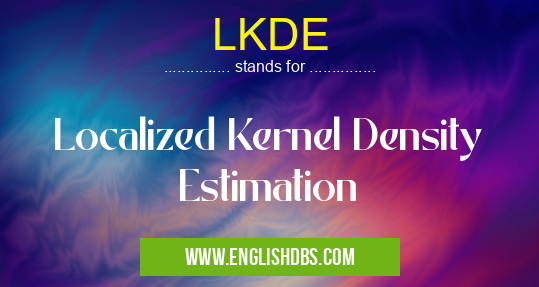
LKDE meaning in Unclassified in Miscellaneous
LKDE mostly used in an acronym Unclassified in Category Miscellaneous that means Localized Kernel Density Estimation
Shorthand: LKDE,
Full Form: Localized Kernel Density Estimation
For more information of "Localized Kernel Density Estimation", see the section below.
Essential Questions and Answers on Localized Kernel Density Estimation in "MISCELLANEOUS»UNFILED"
What is Localized Kernel Density Estimation?
Localized Kernel Density Estimation (LKDE) is a type of nonparametric statistical technique that uses kernel-based approaches to estimate the probability density function of a given dataset without making underlying assumptions about its distribution.
How is LKDE different from Parametric Methods?
Unlike parametric methods, which use predetermined formulas and assumptions about the data distribution, LKDE does not make any underlying assumptions about how the data is distributed. Instead, it uses individual data points as well as their local neighborhood to calculate the probability density estimates for each point.
What advantages does LKDE have over Parametric Methods?
The main advantage of using LKDE over parametric methods is that it results in more accurate estimates leading to better predictive performance.
Is there any disadvantage of using LKDE?
The primary disadvantage of using LKDE over parametric methods is that it requires more computational resources since it needs to deal with an entire dataset instead of just predefined formulas and models. Also, due to its reliance on local neighborhoods for calculation probabilities can lead to inconsistencies when dealing with datasets with outliers or extreme values.
Is LKDE suited for all kinds of datasets?
No, LKDE might not be suitable for certain types of datasets such as imbalanced or skewed distributions where other estimating techniques may be preferred.
Final Words:
Overall,Localized Kernel Density Estimation (LKDE) can be seen as an effective nonparametric statistical technique when used correctly on appropriate datasets due to its ability to accurately estimate probabilities without making any prior assumptions about the underlying data distribution and providing superior predictive performance compared to other techniques involving predefined formulas and models.