What does LFDE mean in UNCLASSIFIED
Local Fisher Discriminant Embedding (LFDE) is a method used for supervised data dimensionality reduction. It is a linear transformation technique that projects multidimensional data onto a lower-dimensional space while preserving the neighborhood structure and local discriminant information contained in the original dataset. The goal of LFDE is to map samples of multiple classes from high dimensional feature spaces into lower dimensional feature spaces while preserving their relative distances. In this way, it can effectively reduce the dimensionality of datasets while simultaneously preserving or even improving the discriminative power of its features.
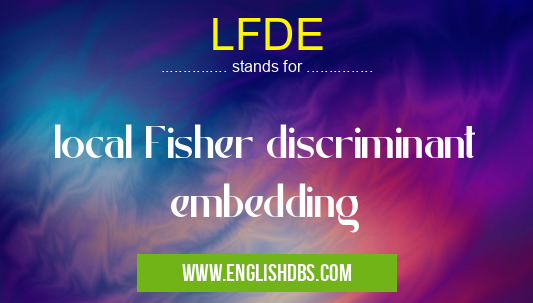
LFDE meaning in Unclassified in Miscellaneous
LFDE mostly used in an acronym Unclassified in Category Miscellaneous that means local Fisher discriminant embedding
Shorthand: LFDE,
Full Form: local Fisher discriminant embedding
For more information of "local Fisher discriminant embedding", see the section below.
Essential Questions and Answers on local Fisher discriminant embedding in "MISCELLANEOUS»UNFILED"
What is Local Fisher Discriminant Embedding?
Local Fisher Discriminant Embedding (LFDE) is a type of discriminant analysis that is used to reduce the dimensionality of data while preserving the maximum amount of class-discriminatory information. This technique works by calculating only those features from a dataset with significant, class-distinguishing differences. The resulting feature set is then mapped onto a lower dimensional space, which can be used to classify data more accurately and efficiently.
How is LFDE different from traditional discriminant embedding?
Unlike traditional discriminant embedding which works on global analysis across all variables in the dataset, LFDE focuses only on those variables that elicit significant class-separating differences between classes. This approach allows for better localization of the discriminative features and results in higher accuracy when applied to classification tasks. Additionally, since LFDE only considers relevant variables, it helps reduce overfitting and improves generalization capabilities of models.
What kind of problems can LFDE be used for?
LFDE can be used for any type of supervised learning task where there are multiple output classes. Typical applications include image recognition, natural language processing, text classification, behavior analysis, medical diagnosis and customer segmentation tasks. It allows for efficient feature selection using fewer computations than regular discriminant embedding methods and provides improved results when compared to conventional linear or logistic regression techniques.
How does one calculate LFDE?
Calculating LFDE involves a two-step process – firstly one must calculate the within-class scatter matrix which represents the matrix containing variance information about features within each class; secondly one must calculate the between-class scatter matrix which captures variance between different classes in terms of their respective features. These two matrices are then combined and solved with an eigenvalue decomposition to obtain the linear transformations needed to map input dimensions into a new lower dimensional space with maximum discriminatory information retained.
What is a scatter matrix?
A scatter matrix is simply a table that contains pairs of means for every variable under consideration in relation to all other variables present in the dataset. Each cell within this table contains either sums or squares or both related to particular points in the overall dataset. For example if we consider three distinct variables X1, X2 and X3 then this table would contain 9 cells representing all possible combinations between these three variables - (X1-X1), (X2-X2), (X3-X3), (X1-X2), (X1-X3), (X2-X3).
What advantages does using LFDE bring?
Utilizing LRFE can result in several advantages over traditional feature selection and extraction methods such as improved accuracy due to its increased focus on local class discriminating information; faster training times due to reduction in dimensionality; more robust models against noisy datasets; less prone to overfitting; better interpretability thanks to its visual nature; less computational complexity; better generalization performance thanks reduced complexity.
What challenges is associated with using LFDE?
Although seeking local Fisher discriminative information has been found beneficial for classification tasks it comes at certain costs such as increased computational burdens due preparation steps required before running LDFE algorithm while also requiring tuning certain parameters depending on individual problem formulations.
Final Words:
In conclusion, Local Fisher Discriminant Embedding (LFDE) is an algorithm for supervised data dimensionality reduction that preserves or even enhances discriminative power in datasets with multiple classes. It achieves this by mapping samples from high dimensional feature spaces into lower ones while maintaining their relative distances through linear transformations such as eigenvector computation and projection onto these vectors. Popular uses cases for LFDE include face recognition applications since it allows individual identification through facial features alone with improved accuracy due to fewer dimensions being considered when making comparisons across classes.