What does KGDA mean in MEDICAL
Kernel Generalised Discriminant Analysis (KGDA) is a feature extraction technique used in machine learning. KGDA works by transforming input data so that it can be separated into two or more distinct classes or clusters using a mathematical function. It is commonly used in classification problems to reduce the dimensionality of the problem space and improve accuracy.
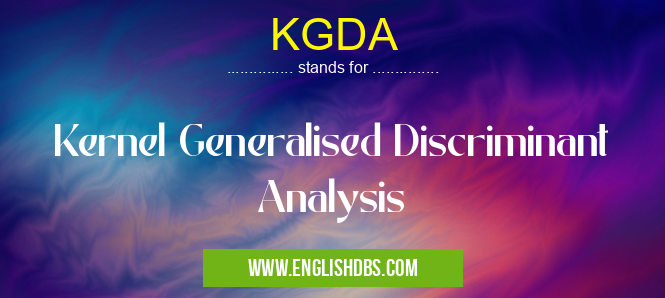
KGDA meaning in Medical in Medical
KGDA mostly used in an acronym Medical in Category Medical that means Kernel Generalised Discriminant Analysis
Shorthand: KGDA,
Full Form: Kernel Generalised Discriminant Analysis
For more information of "Kernel Generalised Discriminant Analysis", see the section below.
Essential Questions and Answers on Kernel Generalised Discriminant Analysis in "MEDICAL»MEDICAL"
What is Kernel Generalised Discriminant Analysis (KGDA)?
KGDA is a feature extraction technique used in machine learning. It works by transforming input data so that it can be separated into two or more distinct classes or clusters using a mathematical function.
How does KGDA work?
KGDA uses a mathematical function to transform input data so that it can be divided into two or more distinct classes or clusters. This helps to reduce the dimensionality of the problem space and improve accuracy in tasks such as classification.
What is KGDA commonly used for?
KGDA is commonly used for classification problems, where it can reduce the dimensionality of the problem space and help to improve accuracy.
Does KGDA work on all types of data?
No, KGDA only works with continuous numeric data, meaning it cannot be applied to categorical or text data without first being converted into numeric representations.
Is there any other benefit of using KGDA?
Yes, as well as reducing the dimensionality of the problem and improving accuracy in classification tasks, KGDA can also increase the interpretability of results since its transformations are easy to understand and explain to end users.
Final Words:
Kernel Generalised Discriminant Analysis (KGDA) is an effective machine learning technique which can be used to achieve accurate classifications on continuous numeric data sets while also reducing the complexity of the computation required and providing an increased level of interpretability in results.