What does KEE mean in ENGINEERING
KEE stands for Knowledge Engineering and is a branch of artificial intelligence that focuses on the process of capturing, representing, and debugging knowledge by machines. It also involves the development of effective algorithms that use knowledge representations to perform tasks such as problem solving, planning, and natural language processing. Knowledge Engineering explores methods for extracting knowledge from expert systems, data mining, machine learning, and other sources to build intelligent computer systems.
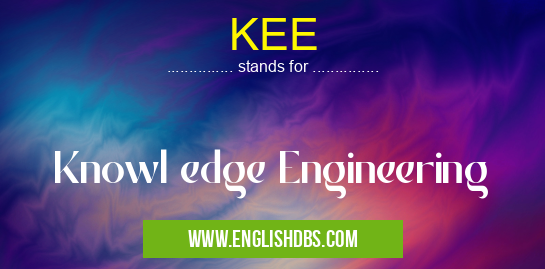
KEE meaning in Engineering in Academic & Science
KEE mostly used in an acronym Engineering in Category Academic & Science that means Knowl edge Engineering
Shorthand: KEE,
Full Form: Knowl edge Engineering
For more information of "Knowl edge Engineering", see the section below.
What is KEE
Knowledge engineering is the process of acquiring knowledge from various sources including experts in a field or from existing databases and then encoding it in a form that can be understood by computer programs. This acquired knowledge is stored in the form of rules or models which can then be used by intelligent software applications to automate decision-making processes or solve complex problems. The process of designing such software applications requires expertise from several disciplines including artificial intelligence (AI), information engineering, linguistics, reasoning algorithms, data modeling, statistics and optimization techniques.
Applications of KEE
KEE has many applications in different fields. In medicine, doctors use AI technologies to assist with diagnoses and treatments. In business and finance, financial advisors are beginning to use automated decision-making tools based on KEEs for investing and portfolio management. In logistics warehouses are using automated sorting systems partially based on rules extracted with KEEs. Other applications include driverless cars using sensors combined with rule-based models for driving decisions; surveillance cameras analyzing incoming streams with vision algorithms; predictive maintenance systems using fault-detection models; marketing campaigns automating customer segmentation with clustering algorithms; personalized health coaches providing specialized wellness advice; robot assistants helping people around their homes; internet search engines improving user experience using semantic analysis; virtual assistants recognizing voice commands using deep learning models; product recommendation engines suggesting products based on customer preferences.
Essential Questions and Answers on Knowl edge Engineering in "SCIENCE»ENGINEERING"
How does knowledge engineering work?
Knowledge engineering is a field of artificial intelligence that focuses on the development of knowledge-based systems. In this field, engineers use machine learning algorithms and expert systems to create systems or devices that can think and act like humans. These machines can then be used to solve problems, extract knowledge from data, make decisions and perform other intelligent tasks.
What are the roles of a knowledge engineer?
A Knowledge Engineer plays a critical role in creating and maintaining knowledge based system. They work with multiple stakeholders to understand their requirements, build models for these requirements using techniques such as machine learning, natural language processing, semantic web technologies and data mining. They also review existing models, suggest improvements where necessary and ensure that the system operates as designed.
What are some advantages of knowledge engineering?
The main advantage of knowledge engineering is that it helps to automate decision making processes thereby improving overall productivity. It also allows users to have access to richer and more complex information sources which can lead to better decision-making capabilities. Moreover, by utilizing automated methods of reasoning it accelerates problem-solving processes thereby leading to more accurate results in shorter time frames.
How is knowledge engineering related to artificial intelligence (AI)?
Knowledge Engineering is closely related to Artificial Intelligence (AI) as both involve the development of automated methods for problem solving or decision making support. However, AI takes into account factors such as neural networks in order to generate automated decisions while Knowledge Engineering mainly relies on logical inference for its decision making capability.
What are some applications of knowledge engineering?
Knowledge Engineering has become increasingly important over recent years due its potential applications in various fields including finance, healthcare and logistics planning among others. For example, in healthcare it could be used for medical diagnosis while in finance it could be used for financial forecasting or stock market predictions. Additionally, it could also be employed for automation of customer service operations or retail analytics within organizations.
How is knowledge engineering applied in industry?
One example that demonstrates the application of Knowledge Engineering within industry would be Machine Learning Powered analytics platform; an automation system that provides insights on customer behaviours through analysis of large datasets concerning customer trends & preferences such as purchase history & activity history etc. Such AI powered platforms will help companies better understand customer behaviour & improve engagement with customers enhancing overall user experience & thus increasing consumer satisfaction.
What are some challenges associated with using knowledge engineering?
Some common issues encountered when using Knowledge Engineering include lack of domain expertise amongst engineers working on the project and lack of data availability due to privacy concerns or cost implications associated with collecting further data sets due a need more comprehensive understanding of the problem domain being analysed by the model . Additionally unstructured data availability often requires significant effort into pre-processing before usable results can be obtained from analyses.
What technology do you need for implementing knowledge engineering projects?
There are several tools available which can assist developers when building Knowledge Engineering powered solutions; most commonly these include rule-based engines (such as Drools), deep learning libraries (like Tensorflow) , NLP frameworks (such as spaCy)and graph databases(such as Neo4J). Additionally many supporting libraries exist around these core components enabling developers t o quickly implement custom solutions tailored towards specific requirements
Final Words:
KEE has become increasingly important due to its wide range of practical applications—from medical diagnostics to financial investments—and continues to be an active research topic in AI today. By combining the wisdom of experts with powerful machine learning algorithms KEE has enabled significant progress towards building more autonomous machines across all industries. As technology progresses across our everyday lives there’s no doubt that KEE will continue to play an integral role in automation.
KEE also stands for: |
|
All stands for KEE |