What does IFKN mean in UNCLASSIFIED
IFKN (Interval Fuzzy Kohonen Network) is a type of self-organizing artificial neural network based on the fundamentals of fuzzy logic and Kohonen networks. It is widely used in various applications such as data mining, industrial process control, image processing, predictive modeling, and geographical information systems. IFKNs are capable of learning from their environment and can be adapted readily to any new set of inputs. IFKNs work by clustering a set of data points into distinct groups that represent some underlying structure or relationship between them. By doing so, they are able to identify patterns in the data which can then be used to make decisions regarding input variables.
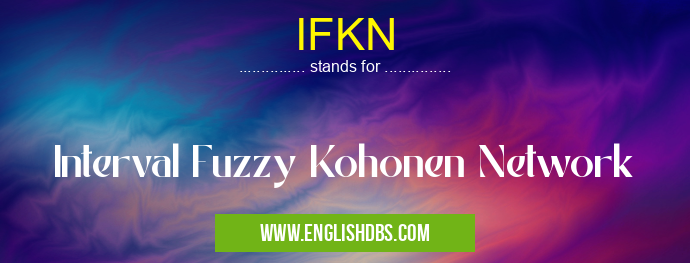
IFKN meaning in Unclassified in Miscellaneous
IFKN mostly used in an acronym Unclassified in Category Miscellaneous that means Interval Fuzzy Kohonen Network
Shorthand: IFKN,
Full Form: Interval Fuzzy Kohonen Network
For more information of "Interval Fuzzy Kohonen Network", see the section below.
Explanation
Interval Fuzzy Kohonen Networks (IFKN) combine two powerful techniques for pattern recognition – fuzzy logic and Kohonen networks. Fuzzy logic allows IFKNs to interpret vague or imprecise input information by assigning it to one of several possible categories; this helps them recognize patterns which may not otherwise be detected due to noise in the input data. On the other hand, Kohonen networks enable IFKNs to learn from their environment by clustering input variables into groups which form new associations between them. This is done through an unsupervised learning process called ‘self-organization’ where the IFKN automatically adjusts its parameters as needed in order to best fit the given dataset. Because of this ability, IFKNs are particularly well-suited for use in applications such as data mining and image processing where large volumes of intricate data need to be processed quickly and accurately.
Essential Questions and Answers on Interval Fuzzy Kohonen Network in "MISCELLANEOUS»UNFILED"
What is an Interval Fuzzy Kohonen Network?
An Interval Fuzzy Kohonen Network (IFKN) is a type of artificial neural network where neurons undergo unsupervised learning through competitive processes. This type of network typically uses fuzzy logic, which allows for a much larger pool of input data and more flexibility. The aim of this type of network is to model the behavior of complex systems, such as those used in pattern recognition, control systems, forecasting, optimization problems, image processing, and robotics.
How does an IFKN work?
An IFKN consists of multiple neurons arranged in layers that communicate with each other through connections. Each layer has its own characteristics that determine how the neuron will react to the inputs from other neurons. Through this interlayer communication, the IFKN can learn and adapt over time to different inputs. Instead of using traditional simulations or supervised learning techniques like backpropagation, an IFKN works by adjusting its weights iteratively until desired output is achieved.
What are the benefits of using an IFKN?
Compared to traditional neural networks, IFKNs are more adaptive and flexible because they better modulate input features according to their importance in solving a problem. They also have a higher level of computational efficiency due to their distributed nature and can provide better generalization capabilities since they can extract multiple levels of abstraction from data sets. Finally, these networks show excellent performance even when exposed to noisy inputs or changes in parameters over time.
How is an IFKN able to classify new patterns?
An IFKN is able to assign a class label based on associating a given pattern with the most similar one already stored in its memory system by means of comparing weights associated with both patterns (in fuzzy terms). The new pattern gets assigned to whichever class it is most closely related based on these similarities. In addition, it can use supervised training mechanisms if needed for further refinement during classification tasks.
What types of problems are best tackled with an IFKN?
An IFKN’s ability to recognize patterns combined with its flexibility make it suitable for many types of machine learning challenges including object detection and tracking as well as natural language processing tasks involving classification or clustering. It can also be applied in robotics for navigation and obstacle avoidance tasks as well as in predicting stock prices or weather forecasts.
How does training an IFKN differ from training other networks?
Training an Interval Fuzzy Kohonen Network differs from traditional neural networks mainly because it doesn't rely on backpropagation algorithms but instead uses probability-based methods within the training procedure itself. For example, it uses fuzzy clustering techniques which allow for greater adaptability depending on certain external factors such as noise or changing parameters throughout execution time.
What kind of data do I need for training an Interval Fuzz Kohonen Network?
Training a network requires you to feed it with labeled data sets so that certain patterns can be learned and recognized during execution time. These datasets should contain real values rather than binary ones since online learning capabilities will likely involve some numerical computation along the way.
Is there any advantage for using fuzzy logic with Interval Fuzzy Kohonen Networks?
Yes! By using fuzzy logic techniques within the network structure itself, you'll get access to more accurate predictions faster without worrying about having exact numbers sometimes needed by more traditional methods such as backpropagation or other supervised techniques which might need slightly different input values.
Is there anything special I need to pay attention while creating my own Interval Fuzzy Kohonen Network implementation?
Definitely! One important thing consider is that changing neuron weights should be done cautiously since too drastic adjustments could potentially damage your entire network over time leading them into suboptimal solutions so pay close attention when doing so.
Final Words:
In summary, Interval Fuzzy Kohonen Networks (IFKN) offer a powerful combination of fuzzy logic and self-organizing networks for pattern recognition tasks. Their ability to quickly identify patterns in large datasets makes them invaluable tools for applications such as image processing, data mining, predictive modeling, and geographical information systems. Additionally, their flexibility also allows them to easily adapt to changing input conditions with little additional effort required from the user. With these advantages, IFKNs remain an indispensable tool for many areas of research today.