What does AMIS mean in UNCLASSIFIED
Adaptive Mixture Importance Sampling (AMIS) is an algorithm for probabilistic inference that enables the efficient exploration of complex distributions. It has been used to solve challenging Bayesian inference problems in statistics, machine learning, and artificial intelligence. AMIS combines a number of powerful techniques into one unified method, such as adaptive importance sampling, annealing, and mixture-based approaches. This makes it a valuable tool for performing approximate probabilistic inference over large datasets or in the presence of multiple strongly correlated variables. In this article, we present an overview of AMIS – what it is and how it works – to help you better understand its potential applications for your data science projects.
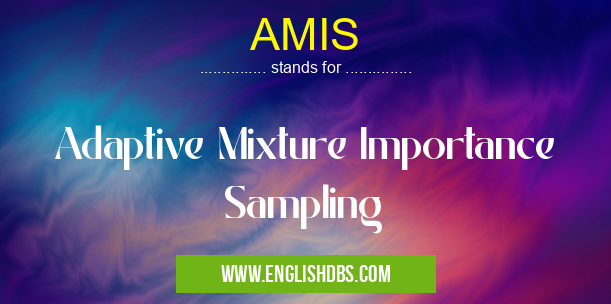
AMIS meaning in Unclassified in Miscellaneous
AMIS mostly used in an acronym Unclassified in Category Miscellaneous that means Adaptive Mixture Importance Sampling
Shorthand: AMIS,
Full Form: Adaptive Mixture Importance Sampling
For more information of "Adaptive Mixture Importance Sampling", see the section below.
Explanation
Adaptive Mixture Importance Sampling (AMIS) is a powerful technique for approximating probability distributions in situations where it is difficult or impossible to obtain exact solutions. It combines two well-known methods - importance sampling and annealing - with a novel optimization approach based on mixtures of separate components. The result is a robust, highly scalable method of probabilistic inference that can be used to accurately solve large scale problems involving multiple parameters and correlations between them. Importance sampling involves randomly generating samples from a chosen probability distribution. These samples are compared to the observable data points and assigned weights based on their relevance. This allows us to estimate the probability of any given result without relying on exact knowledge about the underlying process generating the data. Annealing refers to adjusting the temperature parameter so that optimization can find its way through local minima, instead of getting stuck in these traps during search operations. Mixtures, meanwhile, involve combining different probability distributions into one weighted sum according to their significance relative to each other within a given context. By employing these three techniques together within ARC's AMIS framework, we are able to accurately approximate complex probability distributions quickly and efficiently with maximum accuracy using minimal computational resources.
Essential Questions and Answers on Adaptive Mixture Importance Sampling in "MISCELLANEOUS»UNFILED"
What is Adaptive Mixture Importance Sampling (AMIS)?
Adaptive Mixture Importance Sampling (AMIS) is a numerical method used to simulate the behavior of complex dynamical systems. It combines Monte Carlo sampling and importance sampling to produce efficient simulations of stochastic dynamical systems, which makes it useful for problems such as optimal control and optimization.
How does Adaptive Mixture Importance Sampling work?
AMIS works by drawing samples from multiple distributions and fitting a mixture model to those samples in order to determine the most probable trajectory in the system. This allows for an efficient simulation of even very complex systems without sacrificing accuracy.
What kind of problems can be solved using AMIS?
AMIS can be used to solve many types of problems including dynamic programming, stochastic optimization, and control engineering. Additionally, it can be used to explore how different parameters affect the behavior of certain systems.
Are there any limitations associated with AMIS?
While AMIS is a powerful tool for simulating complex dynamical systems, it has its limits. Specifically, it does not easily scale up when dealing with large state spaces or when interactions between states are very complicated. In these cases a more sophisticated algorithm may be necessary.
What type of data do I need in order to use AMIS?
In order to apply AMIS you need data that describes your system at various points in time or space, such as position, intensity, temperature etc. The more data you have the better your results will be.
Does using AMIS require any special hardware or software?
No special hardware is required for using AMIS; however some software packages are available specifically designed for this purpose. These include GFM Toolbox and MOESP Toolbox, among others.
Final Words:
Adaptive Mixture Importance Sampling (AMIS) provides an effective algorithm for solving large scale inference problems that would otherwise be too computationally intensive or difficult to solve using traditional techniques. By combining importance sampling with annealing and mixture approaches within its framework, AMIS can accurately approximate even highly intricate probability distributions while making optimal use of computational resources available at hand.
AMIS also stands for: |
|
All stands for AMIS |