What does IDBN mean in MATHEMATICS
IDBN stands for Infinite Dynamic Bayesian Network. It is a statistical machine learning model that is used to represent a system with multiple, interacting components. The features of an IDBN are similar to those of a standard Dynamic Bayesian Network (DBN), however, it goes beyond what a DBN can do by allowing for arbitrarily large numbers of nodes in the network and by dynamically adapting connections between them over time. IDBNs are particularly useful in areas such as natural language processing, pattern recognition, and anomaly detection. In this article, we will discuss what an IDBN is and how it works
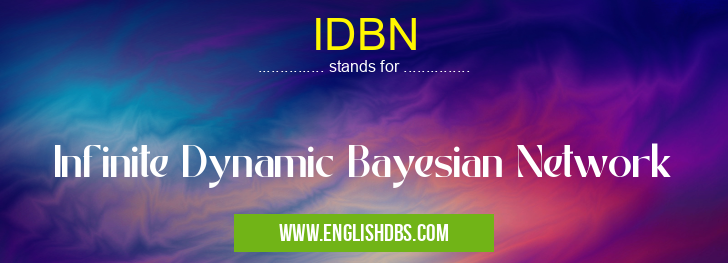
IDBN meaning in Mathematics in Academic & Science
IDBN mostly used in an acronym Mathematics in Category Academic & Science that means Infinite Dynamic Bayesian Network
Shorthand: IDBN,
Full Form: Infinite Dynamic Bayesian Network
For more information of "Infinite Dynamic Bayesian Network", see the section below.
Essential Questions and Answers on Infinite Dynamic Bayesian Network in "SCIENCE»MATH"
What is a Infinite Dynamic Bayesian Network?
An Infinite Dynamic Bayesian Network (IDBN) is a type of probabilistic graph model for representing and reasoning about dynamically changing networks of variables over time. In an IDBN, each variable is represented by a node in the graph, and the edges between them represent their relationship. IDBNs can be used to model complex temporal systems, such as those found in robotics, natural language processing, medical diagnosis, and more.
How do Infinity Dynamic Bayesian Networks work?
An IDBN works by applying probability theory to represent uncertain relationships between different variables over time. It consists of two components; a belief network which represents beliefs about past events or states, and an influence network which updates the beliefs given new information. By combining these two networks, an IDBN can learn from experience and accurately predict future outcomes.
What are the benefits of using an Infinite Dynamic Bayesian Network?
An IDBN has several advantages over traditional machine learning models. First, it allows for temporal modeling where the current state depends on past states instead of just taking independent measurements at each timestep. This allows for more accurate predictions because it takes into account how data points may change over time due to external influences or hidden variables that weren’t accounted for in the original model. Additionally, because an IDBN uses probability theory it's able to represent uncertainty in its predictions and allow for robust decision making based on incomplete information or prior knowledge. Lastly, it's highly scalable since it works with large datasets without needing to make any modifications to the network structure or parameters.
Where can I use an Infinite Dynamic Bayesian Network?
An IDBN can be used in many different types of applications where temporal data needs to be predicted or modeled accurately over time. Some examples include natural language processing tasks like dialog systems or sentiment analysis; robot navigation tasks where decisions need to be made based on environmental conditions; medical diagnostics where patterns need to be identified from dynamic patient data; financial forecasting tasks where future stock prices need to be extrapolated from historical trends; and many more scenarios which involve temporal reasoning and decisions under uncertainty.
Does training take a long time with an Infinite Dynamic Bayesian Network?
Training times vary depending on the size and complexity of your dataset but generally speaking they don't take too long compared with other machine learning models such as deep neural networks. This is because an IDBN runs multiple iterations until convergence which usually takes less time than training other models from scratch each time you need updated results.
What types of algorithms are used in Infinity Dynamic Bayesian Networks?
The core algorithm used in most implementations of IDsNs is called Stochastic Gradient Descent (SGD), although some variations use other optimization techniques such as Markov Chain Monte Carlo (MCMC). SGD works by iteratively updating weights assigned to each edge in order to maximize performance on a chosen metric such as accuracy or precision.