What does HMM mean in MATHEMATICS
Hidden Markov Model (HMM) is a powerful tool for modeling stochastic process which can be used in a wide variety of applications. HMM provides a way to build complex systems by using simple components, allowing us to better approximate and understand natural phenomena. HMM is a type of probabilistic model that consists of a set of states connected by probabilistic transitions over time. It is commonly used in Natural Language Processing (NLP), speech recognition systems and bioinformatics.
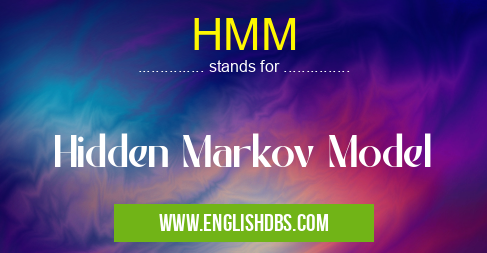
HMM meaning in Mathematics in Academic & Science
HMM mostly used in an acronym Mathematics in Category Academic & Science that means Hidden Markov Model
Shorthand: HMM,
Full Form: Hidden Markov Model
For more information of "Hidden Markov Model", see the section below.
Meaning
In Science, HMM stands for Hidden Markov Model - an adaptable mathematical tool used to predict the most likely sequence of events given an initial state. A hidden Markov model has three main components – states, transition probabilities and emission probabilities. The states represent certain conditions or events in the environment while transition probabilities indicate how likely it is that one state will transition into another state over time. Emission probabilities indicate the probability of observing certain output given some input from the environment. Together, all these components make up an HMM’s structure which can then be applied to real-world data sets in order to make predictions or gain insight into the system’s behavior over time.
Full Form
HMM stands for Hidden Markov Model, which is a discrete-time stochastic process whose latent variables are not directly observed but inferred from the observations you have available upon running your simulations. This means that based on the observations that you take from your simulations you can use them to calculate how probable or possible it is for a certain event or outcome to occur and this allows you to estimate the probability distribution of future outcomes or events based on previous observations making it an incredibly useful tool when analyzing data and making predictions about future outcomes.
Essential Questions and Answers on Hidden Markov Model in "SCIENCE»MATH"
What is a Hidden Markov Model?
A Hidden Markov Model (HMM) is a probabilistic model used for predicting the likelihood of sequence patterns in data. The model assumes that each state in the sequence can only be observed indirectly, making it an unsupervised learning technique. HMM’s are also used in speech and handwriting recognition, robotics, and automatic image segmentation.
How do HMMs work?
An HMM is based on a Markov chain, which is a system with components that are connected by transitions between states. The components are represented as probability distributions over “hidden” variables. The transition probabilities represent how likely it is for the system to move from one state to another. Based on these transitions, we can calculate the likelihood of observing certain sequences of events given certain prior states.
What are the different types of HMMs?
There are two main types of HMMs – Forward-Backward and Baum-Welch algorithms. In Forward-Backward algorithms, observations at each time step are only dependent on the current and previous states, while Baum-Welch algorithms allow more flexibility by using forward and backward messages to update transition probabilities over time. Both types can be used to identify patterns in data sets or to make predictions about future events based on past observations.
What are some applications of HMMs?
HMMs have been used extensively in speech recognition, handwriting recognition, image segmentation, robotics control systems, and bioinformatics research such as prediction of protein structures and gene regulation networks. They have also been employed for machine translation and topic identification tasks involving natural language processing (NLP).
What advantages does an HMM have over other machine learning techniques?
One advantage of using an HMM is that it allows for flexible modeling of temporal dependence among observations without having to explicitly define all possible combinations of states or parameters for each observation point separately. This makes it easier to build models that capture complex temporal relationships without requiring too much manual intervention or exploratory searching through state space configurations like other machine learning techniques require.
How do I build an HMM?
Building an HMM requires two steps – first you must define the model structure which includes specifying the number of states in your model as well as how they interact with each other; then you need to estimate its parameters based on training data using either a maximum likelihood estimation or Bayesian inference approach. Once these two steps have been completed you can use your trained HMM model to make predictions about unseen data points.
Final Words:
Overall, we can see that Hidden Markov Model (HMM) is an important tool used across many disciplines such as Natural Language Processing (NLP), Speech Recognition Systems and Bioinformatics due its ability to work with various types of data sets and produce accurate results even under incomplete information scenarios. By leveraging HMM's ability to make hidden data visible through observation and inference processes, scientists are able to identify patterns and trends in their research more effectively than before today's modern techniques.
HMM also stands for: |
|
All stands for HMM |