What does HAC mean in UNCLASSIFIED
HAC stands for heteroscedasticity and autocorrelation consistent. These terms are used in statistics to describe errors that can occur when looking at data. Heteroscedasticity is the largest of the two sources, which means that the variance of the data is not constant, but varies depending on other factors. Autocorrelation is a smaller issue and refers to errors in data due to past values influencing current values. Both issues can lead to inaccurate analysis and conclusions if not properly accounted for.
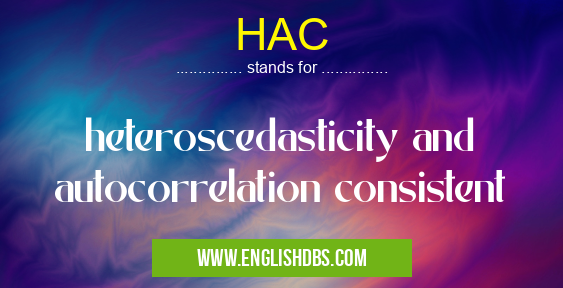
HAC meaning in Unclassified in Miscellaneous
HAC mostly used in an acronym Unclassified in Category Miscellaneous that means heteroscedasticity and autocorrelation consistent
Shorthand: HAC,
Full Form: heteroscedasticity and autocorrelation consistent
For more information of "heteroscedasticity and autocorrelation consistent", see the section below.
Heteroscedasticity
Heteroscedasticity occurs when a dataset or variable presents a non-constant variance. This means that as one variable increases or decreases, so does its variance. This makes it difficult to detect linear trends and make estimates with any accuracy as they are dependent upon knowing the variance of the dataset accurately. There are a few commonly used methods to identify heteroscedasticity such as plotting residuals against fitted values, plotting residuals against time or categorical variables, or plotting residual mean squares against fitted values.
Autocorrelation
Autocorrelation occurs when the present value of a variable is correlated with itself from earlier points in time. When this happens it can affect both the magnitude and direction of predictions made from linear regression models due to past values influencing current ones. Methods used for detecting autocorrelation include correlograms and partial correlation plots which show change over time for specified lag periods and/or variables related to each other at different points in time respectively.
HAC
HAC stands for heteroscedasticity and autocorrelation consistent which together refer to two sources of errors that can occur when examining data sets for statistical analysis or forecasting purposes. Heteroscedasticity refers to non-constant variance while autocorrelation refers to past values affecting current ones both of which can lead to inaccurate results if left unaccounted for properly from either manual or automated analysis techniques such as linear regression models or RNNs (Recurrent Neural Networks). To identify these sources of error various methods have been developed such as correlograms or fitting residuals against categorical variables etc., so that they can be accounted for prior to running automated modeling techniques.
Essential Questions and Answers on heteroscedasticity and autocorrelation consistent in "MISCELLANEOUS»UNFILED"
What is HAC?
HAC stands for heteroscedasticity and autocorrelation consistent. This term is used to describe an econometric estimation technique, specifically to address any potential issues related to heteroskedasticity or serial correlation. This technique uses a “sandwich” estimator which is constructed with the goal of eliminating such effects.
How does HAC work?
The HAC estimator sandwiches the estimated variance covariance matrix of the parameter estimates between two “weights” matrices. These weights are designed to capture any potential errors related to heteroskedasticity or serial correlation that may be present in the data set. In this way, the resulting estimate will be asymptotically unbiased and robust against both of these effects, provided they exist in the data set.
What is heteroskedasticity?
Heteroskedasticity occurs when the variance of residuals in a regression model vary across values of explanatory variables. It often leads to inconsistent parameter estimates in standard linear regressions and thus can have substantial impacts on results.
What is serial correlation?
Serial correlation occurs when past residuals are correlated with current ones, making it difficult for errors to remain independent from one another and resulting in bias estimates of parameters.
Why is HAC important?
HAC allows researchers to make more accurate estimations when dealing with data sets that may contain either heteroskedastisty or serial correlation effects due its robust design and use of sandwiching weights matrices. Without addressing these potential issues through techniques such as HAC, the reliability and accuracy of obtained estimations could be compromised.
Is there alternative ways to address heterokedasticity and serial correlation?
Yes, other techniques such as weighted least squares (WLS) exist which can also help account for the presence of either effect, though studies suggest that in some cases WLS may not produce satisfactory results while also being potentially more computationally expensive than Necessary compared to HAC techniques due it's design methodology.
Are there any drawbacks associated with using HAC?
Potential inconsistencies could arise if other sources of bias not addressed by this technique are present in a given data set . In addition, computational costs may increase significantly if your dataset becomes too large or complex since computations rely heavily on matrix operations.
Final Words:
In conclusion, HAC stands for heteroscedasticity and autocorrelation consistent which describes two sources of errors present in most datasets used in data science applications today such as those used for statistical analysis or forecasting tasks like linear regression modeling etc.. If not identified and corrected early then these errors can result in incorrect predictions being made from automated models leading ultimately lead to less reliable decision-making processes at least within its context thus it's important during any data science task that these be accounted for before proceeding onto more complex modeling procedures like RNNs etc..
HAC also stands for: |
|
All stands for HAC |