What does GBHCC mean in RESEARCH
GBHCC stands for Graph Based Hierarchical Conceptual Clustering which is a type of data mining and machine learning technique that is applied to classify different types of objects in a vast database. GBHCC helps the user to learn and understand the relationships between concepts in databases, allowing for effective decision-making and knowledge acquisition. As such, it is an important tool in areas such as business intelligence, financial analysis, and healthcare research. In this article, we will explore how GBHCC works and why it is so useful in these fields.
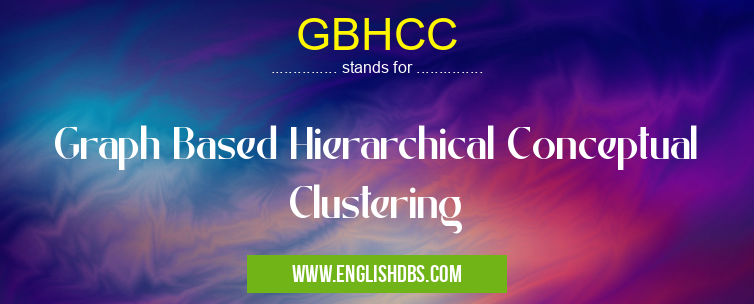
GBHCC meaning in Research in Academic & Science
GBHCC mostly used in an acronym Research in Category Academic & Science that means Graph Based Hierarchical Conceptual Clustering
Shorthand: GBHCC,
Full Form: Graph Based Hierarchical Conceptual Clustering
For more information of "Graph Based Hierarchical Conceptual Clustering", see the section below.
What is Graph Based Hierarchical Conceptual Clustering
Graph-Based Hierarchical Conceptual Clustering (GBHCC) is a form of data mining which uses graphs to identify relationships between objects or concepts that exist within large databases. The process involves drawing connections between the nodes on a graph, where each node represents an object or concept from the database. This allows users to quickly identify patterns and relationships between various objects without having to manually search through them all. Additionally, GBHCC can be used to cluster similar objects together into more meaningful groups for further exploration and understanding.
Applications of GBHCC
GBHCC has found practical applications in many industries and fields such as Healthcare, Retail/Wholesale Businesses, Marketing Analytics, Logistics Management, Financial Services Analysis among others. Specifically, the ability of GBHCC to search through huge amounts of data allow healthcare researchers to draw insightful conclusions about patient populations which are necessary for better decision making when it comes to providing medical services. Similarly businesses use GBHCC's pattern recognition capabilities to uncover hidden correlations in customer behavior or market trends which would otherwise go unnoticed while big-data financial analysts also make use of this technology when it comes time to deal with large datasets representing stocks or real estate properties.
Benefits Of GBHC
The biggest advantage of using Graph-based Hierarchical Conceptual Clustering (GBHC) over traditional clustering methods is its ability to uncover conceptual correlations within very complex datasets which would normally require manual exploration or data processing techniques. By visualizing relationships between attributes on a graph structure users can quickly identify patterns and groupings without needing any prior knowledge about the dataset which makes it easier for them tackle complex analysis tasks without having any prior domain experience or familiarity with the data involved in the task at hand. Additionally GBHC algorithms provide high accuracy when compared side by side with other clustering methods since they perform well even when dealing with nonlinear relationships existing among attributes found within large datasets making them extremely reliable tools within most domains.
Essential Questions and Answers on Graph Based Hierarchical Conceptual Clustering in "SCIENCE»RESEARCH"
What is graph-based hierarchical conceptual clustering?
Graph-based hierarchical conceptual clustering (GBHCC) is a machine learning technique used to organize data into meaningful categories. This technique is based on graphs that represent relationships and hierarchies between the data points. GBHCC allows data sets to be visually organized in ways that are not possible with other clustering techniques, such as k-means or support vector machines.
What types of data can be clustered using GBHCC?
GBHCC can be used for any type of data, including numerical, categorical, text and image data. It can also be used for features extracted from text such as sentiment analysis and topic modeling.
How does GBHCC work?
GBHCC works by constructing a hierarchy of concepts based on a graph representation of the dataset. This graph starts with nodes representing individual elements in the dataset, which are then grouped according to various criteria such as similarity or dissimilarity. The resulting hierarchy can then be used to identify patterns in the data, allowing for more accurate predictions or insights into a given problem.
What are the advantages of using GBHCC?
One major advantage of using GBHCC is that it allows for much more precise categorization than other machine learning methods. Unlike k-means or support vector machines, which rely on distance metrics to group similar points together, GBHCC employs graph representations which allow for much deeper insights into how relationships between elements work within a dataset. Additionally, it can provide greater accuracy when it comes to identification patterns in the data and making predictions or decisions based on them.
What types of problems can GBHCC help solve?
GBHCC can be useful for solving many types of problems including prediction tasks such as classification or regression, anomaly detection, segmentation tasks like customer segmentation and unsupervised clustering tasks like finding patterns in transaction records or web usage logs. It is also useful for discovering hidden relationships between heterogeneous datasets and objects and providing meaningful recommendations from large datasets through concept mapping.
Does GBHCC require labeled data?
No, unlike supervised learning algorithms which require labeled training datasets in order to make predictions with accuracy, labels are not required when using GBHCC since it relies only on the dataset itself rather than external input variables. As a result, this makes it an ideal tool for processing unstructured datasets which may not have existing labels associated with them