What does AIME mean in MEDICAL
Artificial Intelligence (AI) has become a major area of focus for the healthcare industry in recent years. AI-driven applications have the potential to help with data analysis, diagnostics, and many other areas of medical care. AIME, or Artificial Intelligence in Medical Epidemiology, is a term used to describe the use of AI technologies to aid in managing and studying health data. This is especially useful for epidemiological research since it can help researchers uncover patterns and relationships among large datasets that would otherwise be difficult to uncover on their own.
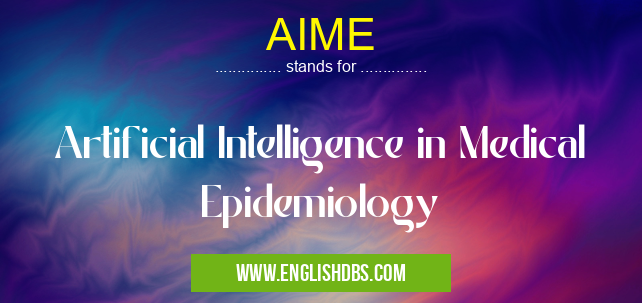
AIME meaning in Medical in Medical
AIME mostly used in an acronym Medical in Category Medical that means Artificial Intelligence in Medical Epidemiology
Shorthand: AIME,
Full Form: Artificial Intelligence in Medical Epidemiology
For more information of "Artificial Intelligence in Medical Epidemiology", see the section below.
What does AIME Stand for?
AIME stands for Artificial Intelligence in Medical Epidemiology. This refers to the application of AI algorithms and technologies to analyze medical data from epidemiological studies. These studies typically involve examining various populations over time in order to understand how diseases are spread across various regions and demographics, as well as identify any trends or correlations between different factors such as environment and lifestyle choices. The application of AI-driven technology can help researchers make sense of large datasets quickly and accurately while providing detailed insights into specific trends or correlations.
What AIME Means In Medical?
In medical terms, AIME stands for Artificial Intelligence in Medical Epidemiology which is used by public health organizations and scientists to uncover patterns within health-related data points from multiple sources. Using specialized machine learning algorithms created specifically for this purpose, researchers can quickly analyze large amounts of data from different sources like population surveys, observational studies or meta-analyses without having to manually look at each record individually – speeding up the process significantly compared to traditional methods. The results are then used to better understand certain diseases’ prevalence among diverse demographics, identify correlations between lifestyle choices or environmental factors that may be contributing factors to disease spread, pinpoint effective interventions that could reduce mortality rates etc., allowing public health organizations around the world take steps towards better managing these conditions before they cause serious damage.
Essential Questions and Answers on Artificial Intelligence in Medical Epidemiology in "MEDICAL»MEDICAL"
What is AIME?
AIME stands for Artificial Intelligence in Medical Epidemiology and involves the use of machine learning algorithms to analyze large sets of data from medical records and clinical trials. It can be used to identify trends, predict outcomes, and better understand disease progression so that treatment plans can be tailored to the individual patient.
What type of data is used in AIME?
AIME utilizes structured data such as demographic information, medical history, laboratory results, and imaging scans to develop predictive models. Unstructured data including doctor’s notes, medical journal articles, and social media posts are also utilized to help interpret the results.
How can AIME improve healthcare delivery?
AIME can reduce errors caused by human fatigue or bias as well as improving decision-making by allowing physicians to quickly review large amounts of data when making clinical decisions. Additionally, it can provide personalized care plans based on an individual patient’s data which can lead to improved health outcomes.
Are there any ethical concerns with using AI in medicine?
Yes, there may be potential ethical concerns with using AI in medicine such as privacy issues if large amounts of personal health information is collected or algorithmic bias if the algorithms are not trained on diverse datasets. Additionally, if physicians begin relying too heavily on AI systems it could lead to decreased skills in making clinical judgement on their own.
What are some applications of AIME?
Some applications include early detection of diseases such as cancer or cardiovascular disease; assisting with diagnosis through pattern recognition; monitoring patient conditions over time; forecasting epidemiological trends; aiding in drug discovery and development; personalizing treatments based on a particular person's characteristics; and providing population health insights.
How does AIME compare with traditional epidemiological methods?
Traditional epidemiological methods rely predominantly on descriptive statistics while AI techniques have opened up possibilities for more advanced predictive modelling approaches which allow for more accurate results when looking at both individual and population-level health behaviours. Additionally, AI is able to process significantly larger datasets than traditional methods due to its ability to leverage automated processes such as machine learning algorithms.
How does accuracy vary when using AIME versus traditional methods?
Accuracy varies depending on the datasets being analyzed but generally speaking AI techniques tend produce higher accuracy rates due to their ability to identify complex patterns within large datasets that may otherwise go unnoticed by humans. However accuracy should never be accepted without validation using traditional statistical approaches first due their proven track record over many decades.
Are there any challenges associated with implementing AIME?
Yes, some challenges associated with implementing AIME include ensuring accurate identification and processing of high quality medical datasets; having access sufficient computing power needed for processing the large volumes of data required for training models; inadequate validation protocols for assessing accuracy levels; difficulty integrating AI techniques into existing workflows and systems; lack of skilled personnel who understand both the technical aspects as well as medical terminology needed to successfully implement these solutions; and ensuring ethical compliance when collecting sensitive personal health information from patients
Final Words:
In conclusion, AIME stands for Artificial Intelligence in Medical Epidemiology which efficient analysis of large datasets through the use of AI-driven technologies has greatly improved our understanding of different diseases’ prevalence across various demographics as well as helping us recognize interesting correlations between factors such as environmental exposures or lifestyle choices that we may not have discovered before using traditional methods alone. With its potential implications in improving public health outcomes around the world – particularly those related to infectious diseases – AIME represents an exciting example of how technology can be used effectively in order to create positive change when properly applied.