What does FLD mean in UNCLASSIFIED
Fisher Linear Discriminants (FLD) is a linear discriminant method used in Machine Learning models and Statistical data analysis. FLD is used to separate two or more classes of data by projecting the data onto a line, plane, or higher-dimensional space such that the classes can be separated as far as possible from each other. The goal is to maximize the overall separation between different classes while having minimal overlap between them. This technique was first introduced by Sir R.A Fisher in 1936 and since then has been applied in various fields of study ranging from pattern recognition to medical diagnosis.
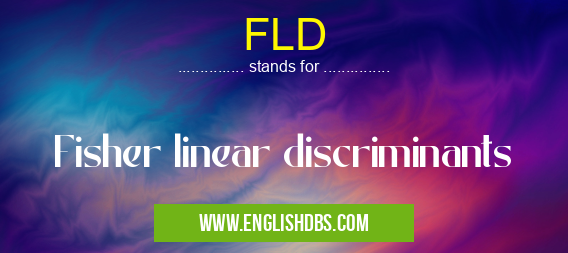
FLD meaning in Unclassified in Miscellaneous
FLD mostly used in an acronym Unclassified in Category Miscellaneous that means Fisher linear discriminants
Shorthand: FLD,
Full Form: Fisher linear discriminants
For more information of "Fisher linear discriminants", see the section below.
What Does FLD Mean?
FLDs measure how well two different classes of data can be distinguished based on their feature vectors. A feature vector is an n-dimensional array of values which represents a single unit of data (e.g., for images: Red intensity, Green intensity, Blue intensity). By applying a linear combination of feature vectors, we can project our data into a higher dimensional space in order to maximize the separation between different classes. In this way, FLDs enable us to distinguish one class from another with greater precision and accuracy than would otherwise be possible with simpler methods such as k-nearest neighbors.
Essential Questions and Answers on Fisher linear discriminants in "MISCELLANEOUS»UNFILED"
What is Fisher Linear Discriminants?
Fisher Linear Discriminants is a supervised machine learning technique that measures the differences between two or more classes of data and assigns each class a set of coefficients. It uses the information gleaned from division points to classify new data points according to their relative similarity to pre-classified data points.
What are the advantages of using Fisher Linear Discriminants?
Using Fisher Linear Discriminants provides an informative method of classification, allowing for data with multiple dimensions to be easily classified. Additionally, this algorithm works well in situations where there are limited training examples and can perform robustly under noisy conditions.
How does Fisher Linear Discriminant work?
Fisher Linear Discriminant operates by calculating the mean values for each class and mapping out an optimal dividing line between them. This line is then used as a classification boundary, so when a new data point comes in it can be assessed against it to determine which class it belongs to.
How useful is Fisher Linear Discriminant in Machine Learning?
Fisher Linear Discriminant has been found very useful in many different machine learning applications, such as facial recognition, natural language processing, biomedical image analysis etc. Furthermore, its robust performance under noisy conditions makes it well suited for practical real world problems where there may be significant amounts of noise in the data.
Are there any limitations to using Fisher Linear Discrimination?
The main limitation is that it can only work effectively with linearly separable data sets; if two classes cannot be clearly separated by a straight line then this technique will not work correctly. Additionally, this technique requires significant manual tuning in order to obtain optimal results, which can make it time consuming and difficult to implement correctly.
What are some common algorithms compared to FISHER linear discriminant methods?
Fisher linear discriminants are often compared against other linear discriminant algorithms such as logistic regression and support vector machines (SVM). While all three are capable of performing binary or multi-class classification tasks they differ based on how they handle different types of data distributions and what parameters need to be tuned for optimal performance.
Is FISHER linear discriminant suitable for high dimensional datasets?
Yes, although FISHER linear discriminant is most effective when dealing with two-dimensional datasets it can also work well with higher dimensional datasets due to its ability to calculate separating lines regardless of dimensionality. However, as mentioned previously these cases require greater manual tuning so extra care must be taken when implementing them.
What type of datasets should you use FISHER linear discriminant with?
FISHER linear discriminant works best when applied with datasets containing linearly separable classes; ie if two classes can easily be distinguished by drawing a straight line between them then this algorithm will give good results quickly and reliably. Additionally any dataset containing multiple dimensions would benefit from this technique.
Final Words:
FLDs are powerful tools that can be used to improve the accuracy and precision of classification models in numerous disciplines including text analysis and image processing. They have wide applications across many industries due to their ability to efficiently match patterns within datasets and find separations between groups that are not always obvious at first glance. With its long history and robust implementation, FLDs can help researchers quickly discover nuanced differences among data points that may prove critical for successful outcomes in research projects or industry studies.
FLD also stands for: |
|
All stands for FLD |