What does AHM mean in UNCLASSIFIED
Applied Hierarchical Modeling (AHM) is an intuitive approach to data analysis and modeling that combines the advantages of statistical and machine learning using a hierarchical structure. It provides a way to collect large amounts of data, create models, and visualize outcomes in an effective and efficient manner. AHM has been widely used for predictive modeling of outcomes in various fields such as healthcare, finance, marketing research, energy forecasting, and sales forecasting. This article provides an overview of AHM's features and how it can be used to solve complex tasks effectively.
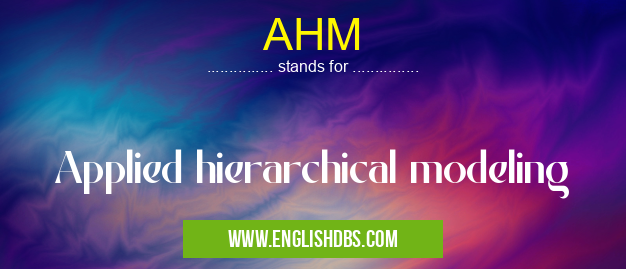
AHM meaning in Unclassified in Miscellaneous
AHM mostly used in an acronym Unclassified in Category Miscellaneous that means Applied hierarchical modeling
Shorthand: AHM,
Full Form: Applied hierarchical modeling
For more information of "Applied hierarchical modeling", see the section below.
What is Applied Hierarchical Modeling (AHM)?
Applied Hierarchical Modeling (AHM) is a data analysis technique that allows for the integration of multiple analytical techniques into a single unified framework. The concept behind AHM is based on the idea that by combining several different techniques such as regression models, neural networks, decision trees, clustering algorithms or even some machine learning approaches into focused groups or levels (or hierarchy), one can achieve superior results compared to methods that use only single technique. The levels in AHM are referred to as “hierarchy” because they represent different levels of abstraction - from lower level descriptive analytics up to higher level predictive analytics. In addition, each level contains different types of data or variables which add value when combined together with other levels.
Benefits of Using Applied Hierarchical Modeling
There are numerous benefits to using AHM as a tool for data analysis and modeling. For starters, the technique enables us to extract useful information from large datasets with high accuracy and low computational cost due to its hierarchical nature allowing for more efficient information processing abilities which result in faster processing times than conventional models can achieve. Additionally, due to its modular structure it is relatively easy to modify specific parts without affecting other components leading to better scalability and flexibility enabling researchers to easily adapt their models when faced with changes in the dataset’s structure or the research goals themselves. Finally, since this method allows for combining multiple analytical techniques at once it creates an ideal environment for dealing with nonlinear relationships between dependent variables by utilizing all tools available instead of relying on one type at a time resulting in much more precise predictions compared those generated by single-model approaches.
Essential Questions and Answers on Applied hierarchical modeling in "MISCELLANEOUS»UNFILED"
What is applied hierarchical modeling?
Applied Hierarchical Modeling (AHM) is an advanced statistical modeling technique used to analyze individual or group-level behaviors and processes over time. It works by analyzing structured data from different sources such as surveys, observations, and experiments. AHM can be used to identify causal relationships between variables and it can also provide insight on how the relationships between these variables might have changed over time.
How is AHM different than other forms of data analysis?
AHM is unique because it takes into account the hierarchical structure of the data when analyzing information. This method of analysis more accurately captures changes over time as well as influences from related factors. Unlike other forms of regression analysis, hierarchical model estimation accounts for correlation structure within each level as well as across levels.
What kind of data does AHM use?
AHM typically uses multi-level or longitudinal data with a nested structure, meaning that there are several distinct levels in the hierarchy that contain related information. Examples of this type of data include student grades organized by classes, by schools and/or by districts; consumer purchases grouped into customer segments; or health outcomes across patients at different hospitals in a region.
What are some applications of AHM?
Applied Hierarchical Modeling can be used for various applications such as understanding customer behavior, predicting employee turnover, forecasting market trends, optimizing business strategies, or examining the impact of medical treatments on patient health outcomes. Additionally, researchers often use it to examine and compare changes in population characteristics over time or study trends in cause-and-effect relationships among multiple variables.
Are there any advantages to using AHM compared to traditional methods?
Yes! Compared to traditional linear regression models, Applied Hierarchical Modeling has several advantages including improved accuracy due to its ability to capture correlations between variables; increased efficiency through the use of fewer coefficients per level; stronger inference power due to its ability to evaluate interactions among multiple levels; and better interpretability since results can be easily visualized in the form of graphs or tables.
Are there any drawbacks associated with using this technique?
The main drawback with applied hierarchical modeling is that it requires specialized expertise and may require additional resources for more complex analyses involving large and heterogeneous datasets. Additionally, if assumptions about the data structure are not verified beforehand they may lead to incorrect results.
What types of software are available for running analyses with this technique?
There are various options available depending on your needs such as R software packages like lme4 and nlme; Python packages like PyMC3; STATA programs like random effects models (REM); SAS procedures including PROC MIXED; or open source solutions such as Heiridata.
Where can I find tutorial materials about this type of modeling?
Tutorials specific to Applied Hierarchical Modeling can usually be found online either through open course materials provided by universities, research papers on the subject, or webinars from third party providers such as Heiridata. Alternatively you could also reach out directly for expert advice from experienced professionals who specialize in this field.
Is there any way I can assess my skills before attempting an AHM problem set?
You can start by looking for introductory courses or tutorials online related to Applied Hierarchical Modeling which will help you understand the basics first if you’re not familiar with it already. If you feel comfortable enough after reviewing those materials then you could try tackling a real dataset which would give you a better sense for how difficult it might actually be once faced with a live problem set.
Final Words:
In conclusion, Applied Hierarchical Modeling (AHM) is a powerful tool for data analysis and modeling offering multiple benefits over conventional approaches like faster processing times due to its hierarchical nature as well as better scalability due its modular structure allowing quick modification when needed without affecting other levels making it ideal for dealing with nonlinear relationships between dependent variables while still being accurate and reliable when generating predictions making it an invaluable asset in many fields such as healthcare or finance where accuracy is vital for success.
AHM also stands for: |
|
All stands for AHM |