What does EPAM mean in UNCLASSIFIED
EPAM stands for Elementary Perception And Memory. It is a type of artificial intelligence (AI) that deals with Computer Vision and Machine Learning. EPAM is used to enable machines to interpret visual data in the same way as humans do, and use it to learn and make decisions.
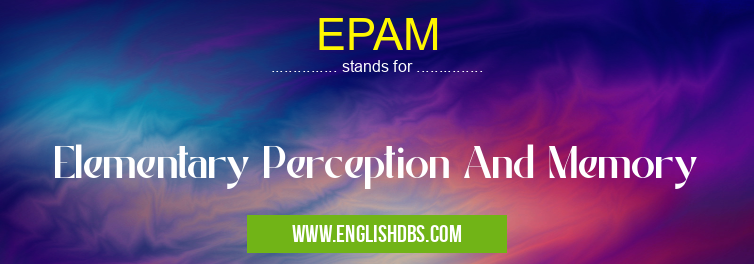
EPAM meaning in Unclassified in Miscellaneous
EPAM mostly used in an acronym Unclassified in Category Miscellaneous that means Elementary Perception And Memory
Shorthand: EPAM,
Full Form: Elementary Perception And Memory
For more information of "Elementary Perception And Memory", see the section below.
Essential Questions and Answers on Elementary Perception And Memory in "MISCELLANEOUS»UNFILED"
What is EPAM?
EPAM stands for Elementary Perception And Memory. It is a type of artificial intelligence (AI) that deals with Computer Vision and Machine Learning.
What can EPAM be used for?
EPAM can be used to enable machines to interpret visual data in the same way as humans do, and use it to learn and make decisions.
How does EPAM work?
EPAM uses algorithms to process visual data such as images, videos and text documents, analyze them, compare them to other data sources, and then generate predictions based on the processed data.
What are some examples of applications that use EPAM?
Examples of applications that use EPAM include natural language processing (NLP), autonomous vehicles, facial recognition, video analytics, robotics and medical diagnosis tools.
Is there any limitation in using EPAM technologies?
Yes, there are limitations when using AI-based systems such as EPAM since they're still limited by their architecture design which may lead to biases or mistakes if not carefully designed. Additionally, since these systems are inspired by biological systems they tend to require large amounts of training data which may be difficult or costly to obtain depending on the application.
Final Words:
EPAM provides powerful tools for building computer vision-based applications with machine learning capabilities which can help automate complex tasks both quickly and accurately. Despite its many benefits however, there are still challenges related to its usage including biases in the data being processed due to architecture design limitations or difficulty in obtaining training datasets.