What does ADSRF mean in UNCLASSIFIED
Adaptive Designs for Stochastic Root Finding (ADSRF) is a method which uses algorithms to solve optimization problems even when the data is uncertain. It has been used to find root nodes in various types of graphs, such as Binary and Generalized Search Trees. ADSRF allows the user to get accurate results when only a few noisy data points are available.
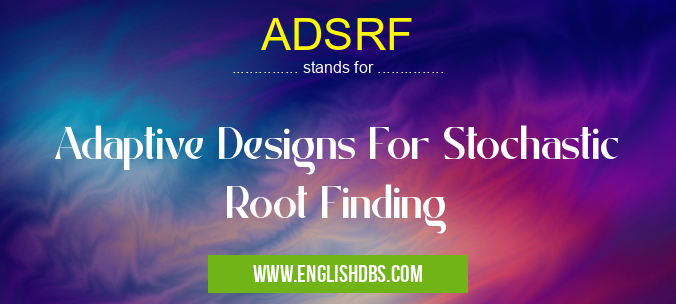
ADSRF meaning in Unclassified in Miscellaneous
ADSRF mostly used in an acronym Unclassified in Category Miscellaneous that means Adaptive Designs For Stochastic Root Finding
Shorthand: ADSRF,
Full Form: Adaptive Designs For Stochastic Root Finding
For more information of "Adaptive Designs For Stochastic Root Finding", see the section below.
Essential Questions and Answers on Adaptive Designs For Stochastic Root Finding in "MISCELLANEOUS»UNFILED"
What is Adaptive Designs For Stochastic Root Finding (ADSRF)?
Adaptive Designs for Stochastic Root Finding (ADSRF) is a method which uses algorithms to solve optimization problems even when the data is uncertain.
What type of graphs can be solved with ADSRF?
ADSRF has been used to find root nodes in various types of graphs, such as Binary and Generalized Search Trees.
How does ADSRF work?
ADSRF uses algorithms that adaptively modify and update their search space given the limited amount of noisy data points that are available so that accurate results can still be obtained.
Is ADSRF widely used?
Yes, many researchers have successfully applied this method to optimize large-scale real-world datasets too.
Does ADSRF offer any advantages compared to other search methods?
Yes, since it requires very little data, it offers time savings compared to other search methods which might require more information before providing a solution. Additionally, its adaptive strategies helps improve accuracy when only a few noisy data points are available.
Final Words:
Adaptive Designs for Stochastic Root Finding (ADSRF) is an important tool for solving optimization problems with limited or noisy data sets quickly and accurately. It provides users with flexible adjustment strategies and helps them exploit any existing information efficiently. As such, it has become an increasingly popular method among researchers working on large scale real world datasets.