What does DDMF mean in UNCLASSIFIED
DDMF stands for Dilation Dependent Matched Filtering, which is an algorithm that is useful for recognizing patterns in a data set. It works by looking at how similar two pieces of information are and then determining if they can be used together to determine the pattern that is being looked for. The algorithm can help researchers identify trends in their data sets and make predictions about future trends. This acronym is commonly used in the fields of machine learning, statistics, and computer science.
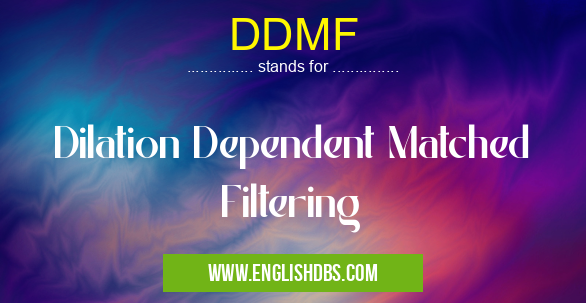
DDMF meaning in Unclassified in Miscellaneous
DDMF mostly used in an acronym Unclassified in Category Miscellaneous that means Dilation Dependent Matched Filtering
Shorthand: DDMF,
Full Form: Dilation Dependent Matched Filtering
For more information of "Dilation Dependent Matched Filtering", see the section below.
Essential Questions and Answers on Dilation Dependent Matched Filtering in "MISCELLANEOUS»UNFILED"
What does DDMF mean?
DDMF stands for Dilation Dependent Matched Filtering, which is an algorithm that compares two pieces of information and looks for patterns in a data set.
How does DDMF work?
DDMF uses the similarity of two pieces of information to determine if they can be used together to identify a pattern. It utilizes machine learning, statistics, and computer science techniques to assess the data and make predictions about future trends.
In what areas is DDMF used?
DDMF is mainly used in machine learning, statistics, and computer science research as a way to recognize patterns in datasets and make predictions based on them.
What are some advantages of using the DDMF algorithm?
Using this algorithm reduces the amount of time needed to find patterns in large datasets because it quickly identifies similarities between data points. Additionally, it is able to accurately predict future trends based on the patterns found within current datasets.
Final Words:
Dilation Dependent Matched Filtering (DDMF) is an effective algorithm for recognizing patterns in large datasets and predicting future trends based on these findings. This method has been widely adopted by research teams across varied disciplines such as Machine Learning, Statistics, and Computer Science due its ability to quickly identify similarities between data points while also making accurate predictions about future trends. With its ability to analyze complex datasets quickly efficiently, this method has become increasingly popular over recent years due its many practical applications.