What does O mean in UNCLASSIFIED
Optimal Transport Dataset Distance (OTDD) is a metric used to measure the similarity between two datasets. It calculates the minimum cost required to transform one dataset into the other through a series of operations, such as inserting, deleting, or replacing elements. OTDD is a powerful tool for data analysis, machine learning, and optimization.
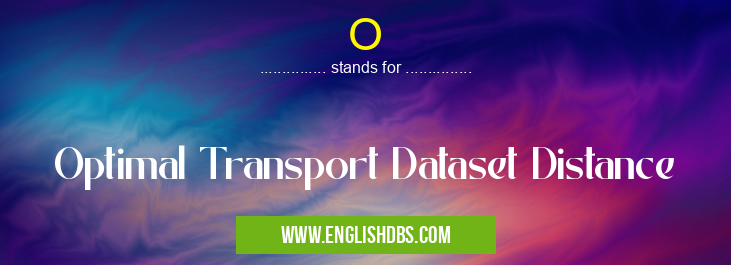
O meaning in Unclassified in Miscellaneous
O mostly used in an acronym Unclassified in Category Miscellaneous that means Optimal Transport Dataset Distance
Shorthand: O,
Full Form: Optimal Transport Dataset Distance
For more information of "Optimal Transport Dataset Distance", see the section below.
Key Features
- Flexibility: OTDD can be applied to datasets of any size or type, including continuous, categorical, and mixed data.
- Robustness: OTDD is robust to noise and outliers in the data.
- Interpretation: The cost of the optimal transport plan provides insights into the differences between the two datasets.
Applications
OTDD has a wide range of applications, including:
- Data clustering: Identifying similar groups of data points within a dataset.
- Machine learning: Improving the performance of machine learning models by finding the best transformation to apply to the input data.
- Optimization: Solving problems that involve finding the minimum cost solution, such as resource allocation and transportation scheduling.
Essential Questions and Answers on Optimal Transport Dataset Distance in "MISCELLANEOUS»UNFILED"
What is the Optimal Transport Dataset Distance (OTDD)?
The Optimal Transport Dataset Distance (OTDD) is a metric that measures the distance between two datasets. It is based on the idea of optimal transport, which is a way of finding the most efficient way to transport one distribution of mass to another. In the context of dataset distance, the two distributions of mass represent the two datasets, and the OTDD is the minimum cost of transporting one dataset to the other.
How is the OTDD calculated?
The OTDD is calculated by solving an optimization problem. The objective of the optimization problem is to minimize the total cost of transporting one dataset to the other. The cost of transporting a single data point from one dataset to another is determined by a distance function. The distance function can be any metric that measures the similarity between two data points.
What are the advantages of using the OTDD?
The OTDD has a number of advantages over other dataset distance metrics. First, it is a non-parametric metric, which means that it does not make any assumptions about the distribution of the data. Second, it is a robust metric, which means that it is not sensitive to outliers in the data. Third, it is a computationally efficient metric, which means that it can be calculated quickly.
What are the applications of the OTDD?
The OTDD can be used in a variety of applications, including:
- Clustering
- Classification
- Anomaly detection
- Data visualization
Final Words: OTDD is a versatile and powerful metric for measuring the similarity between datasets. Its flexibility, robustness, and interpretability make it a valuable tool for data analysis, machine learning, and optimization. OTDD has the potential to contribute to advances in these fields and provide new insights into data.
O also stands for: |
|
All stands for O |