What does PKD mean in UNCLASSIFIED
PKD stands for Patient Knowledge Distillation. It is a technique used in machine learning to improve the performance of a student model by transferring knowledge from a larger, more powerful teacher model. PKD is particularly useful in scenarios where the student model has limited data or computational resources, and the teacher model has access to a larger dataset or more powerful training infrastructure.
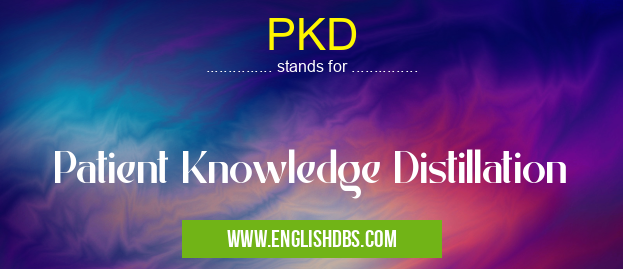
PKD meaning in Unclassified in Miscellaneous
PKD mostly used in an acronym Unclassified in Category Miscellaneous that means Patient Knowledge Distillation
Shorthand: PKD,
Full Form: Patient Knowledge Distillation
For more information of "Patient Knowledge Distillation", see the section below.
How PKD Works
PKD involves two main steps:
- Knowledge Extraction: The teacher model is trained on a large dataset, extracting valuable knowledge and patterns.
- Knowledge Transfer: The knowledge extracted from the teacher model is transferred to the student model through a distillation process. This process involves aligning the predictions and outputs of the teacher and student models, ensuring that the student model learns the essential concepts and relationships captured by the teacher model.
Benefits of PKD
PKD offers several advantages, including:
- Improved Performance: By transferring knowledge from a more powerful model, PKD enables the student model to achieve better performance on downstream tasks.
- Reduced Training Time: The student model can leverage the knowledge already acquired by the teacher model, reducing the training time required.
- Efficient Use of Resources: PKD allows for the creation of smaller, more resource-efficient student models without sacrificing performance.
- Enhanced Generalization: The knowledge distilled from the teacher model helps the student model generalize better to unseen data.
Applications of PKD
PKD has found applications in various domains, including:
- Natural Language Processing (NLP): Transferring knowledge from large language models to smaller models for tasks like text classification and question answering.
- Computer Vision: Distilling knowledge from pre-trained image recognition models to improve the performance of object detection and segmentation models.
- Speech Recognition: Leveraging knowledge from trained speech recognition models to enhance the accuracy of smaller models.
Essential Questions and Answers on Patient Knowledge Distillation in "MISCELLANEOUS»UNFILED"
What is Patient Knowledge Distillation (PKD)?
PKD is a machine learning technique used in medical AI to transfer knowledge from a large, pre-trained AI model (teacher model) to a smaller, more efficient AI model (student model). This allows the student model to achieve near-expert-level performance even with limited training data.
What are the benefits of using PKD in healthcare?
PKD offers several advantages:
- Improved performance: Student models trained with PKD achieve higher accuracy and better generalization capabilities.
- Reduced training time: By leveraging knowledge from the teacher model, student models can converge faster during training.
- Lower computational cost: Smaller student models require less computational resources for training and deployment.
- Increased interpretability: PKD helps make AI models more explainable, facilitating understanding and trust among clinicians.
How is PKD applied in medical AI?
PKD is used in various medical AI applications, including:
- Disease diagnosis: Transferring knowledge from models trained on large datasets to smaller models for accurate diagnosis.
- Treatment planning: Distilling knowledge from models that have learned optimal treatment strategies for specific diseases.
- Prognosis prediction: Using PKD to build models that predict disease outcomes and guide patient care.
What are the challenges associated with PKD?
While PKD offers significant benefits, it also faces challenges:
- Selecting the appropriate teacher model: Choosing the optimal teacher model that provides valuable knowledge is crucial for PKD's success.
- Overfitting to the teacher model: Student models can potentially过度拟合到teacher model的特定训练数据,导致泛化能力下降。
- Balancing knowledge transfer and adaptation: PKD requires careful balancing between transferring knowledge from the teacher model and adapting it to the specific task and data at hand.
Is PKD a new technique?
The concept of knowledge distillation has been applied in various fields for several years. However, its application in medical AI is relatively recent and has gained significant attention due to the need for more efficient and accurate AI models in healthcare.
Final Words: PKD is a powerful technique that enables the transfer of knowledge from a teacher model to a student model, improving the performance and efficiency of the latter. By leveraging the knowledge and patterns learned by larger models, PKD empowers smaller models to achieve state-of-the-art results while optimizing resource utilization. As machine learning models continue to grow in size and complexity, PKD is expected to play an increasingly important role in training and deploying efficient and effective models.
PKD also stands for: |
|
All stands for PKD |