What does MHMM mean in UNCLASSIFIED
MHMM stands for Mixed Hidden Markovian Model, which is a type of statistical model used to study underlying mechanisms behind some observed phenomena. This model has been extensively studied in different fields, such as bioinformatics and signal processing. MHMM is a powerful tool that can be used to track the evolution of complex systems by combining multiple models or using multiple time steps.
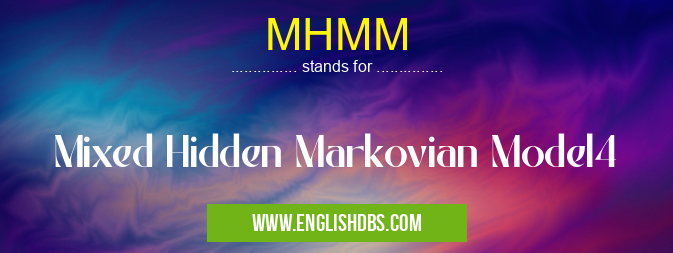
MHMM meaning in Unclassified in Miscellaneous
MHMM mostly used in an acronym Unclassified in Category Miscellaneous that means Mixed Hidden Markovian Model4
Shorthand: MHMM,
Full Form: Mixed Hidden Markovian Model4
For more information of "Mixed Hidden Markovian Model4", see the section below.
Overview
A Mixed Hidden Markovian Model (MHMM) is a probabilistic model that combines two or more different sets of data from observational or experimental observations to create an overall model. The purpose of this type of modelling is to uncover any hidden patterns or relationships across multiple sets and uncover any correlations between them. Such models are useful in understanding complex behavior within biological systems, particularly when there are large amounts of data available from different sources. They are also great tools for predicting responses to certain stimuli and changes in environment conditions. The MHMM involves a series of steps which together form a single framework that allows us to make predictions based on all the data available. The first step is the observation phase, which takes data collected by observation (either from experiments or other sources) and uses it to estimate parameters associated with each set. Then the transition phase combines both sets of parameters into an integrated set so that transitions between states can be modeled as a whole instead of individually. Finally, the prediction phase uses this integrated model to make future predictions about system behavior based on current and past observations.
Advantages
One major advantage of MHMM is its flexibility in dealing with multiple datasets at once without any need for additional expertise or manual reformatting. It also allows us to identify trends over time or explore correlations between different variables without having to manually search through all our data points and rearrange them into separate groups for analysis purposes. Additionally, these models provide accurate results even when only limited or incomplete information is available, making them useful for research applications where access to comprehensive datasets may be limited or unavailable altogether. This makes MHMM particularly attractive for exploring emergent behaviors in complex systems where traditional modeling approaches may be inadequate due to lack of accessible data points or improper parameter selection processes.
Essential Questions and Answers on Mixed Hidden Markovian Model4 in "MISCELLANEOUS»UNFILED"
What is the difference between a Markov Chain and a Markov Model?
A Markov Chain is a type of probabilistic model that shows the probability of transitioning from one state to another within a given sequence. A Markov Model is an extended version of the Markov Chain that incorporates additional features such as memory and other variables.
What Is a Hidden Markov Model (HMM)?
Hidden Markov Models (HMMs) are stochastic models used for modeling sequential data, where some aspects of the model are hidden or unknown. HMMs make predictions based on previous observations while taking into account any probabilistic dependencies between those observations.
How Do I Use a Hidden Markov Model?
To use an HMM, you must first specify the parameters of your model, such as the number of states, possible transitions between states, and emission probabilities for each state. Once these parameters have been determined, you can then use an algorithm to find the most likely sequence of hidden states given your observations.
What Is the Baum-Welch Algorithm?
The Baum-Welch algorithm is an iterative technique used to estimate the parameters of an HMM from a set of known observations. It works by using expectation maximization techniques to find the most likely values for each parameter.
What Are Some Applications for HMMs?
HMMs can be used in a variety of applications such as speech recognition, natural language processing, image processing, audio synthesis and more. They are also commonly used in machine learning tasks such as clustering and classification.
How Does Variational Inference Work with HMMs?
Variational inference is an approximate inference method for Bayesian networks that can be used to estimate probabilistic relationships within large datasets. When used with HMMs, variational inference allows users to infer missing information within their data and generate more accurate statistical models.
What Are Viterbi Paths and How Do They Work?
Viterbi paths are algorithms that calculate the most probable path through a sequence given known transition probabilities between states in an HMM. These can be used in tasks such as predicting gene expression from DNA sequences or recognizing spoken words from audio signals.
What Is Maximum Likelihood Estimation (MLE)?
Maximum likelihood estimation (MLE) is a statistical technique used to estimate parameters such as means and variances from observed data points so they best match those expected under a given probability distribution function. This technique is often employed when working with HMMs due to its efficiency in finding optimal solutions quickly without needing large amounts of data points or intensive computations.
How Can I Visualize My Results with HMMs?
Many visualization tools exist that allow users to explore graphical representations of their results when working with HMMs such as basic graphs showing transition probabilities between states or heat maps illustrating observation probability distributions over time.
What Is EM Algorithm?
The Expectation Maximization (EM) algorithm is an iterative procedure which uses training data samples to infer unknown properties about them like means and variances which favor maximum likelihood estimates within HMMs.
How Does Reinforcement Learning Work With HMMs?
Reinforcement learning is a type of machine learning which involves using rewards to encourage certain actions while penalizing others in order to maximize rewards over time - this approach can be applied when working with HMMs by providing rewards for reaching desired outcomes while penalizing undesired ones.
Final Words:
Mixed Hidden Markovian Models (MHMM) have become increasingly popular in many scientific fields due to their ability to accurately capture patterns found among multiple datasets simultaneously while avoiding manual manipulation steps until absolutely necessary. While not an ideal solution for every problem posed by complex environments, they offer great potential for automated identification processes and can be used effectively in analyzing behaviors found within various types of biological systems such as gene expression networks, metabolic pathways and drug response pathways just to name a few applications.