What does MPL mean in UNCLASSIFIED
MPL (Marginal Path Likelihood) is a statistical technique used in Bayesian inference to estimate the probability of a specific path through a graphical model. It plays a crucial role in various applications, such as machine learning, artificial intelligence, and data analysis.
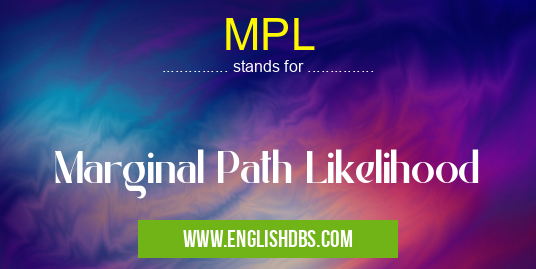
MPL meaning in Unclassified in Miscellaneous
MPL mostly used in an acronym Unclassified in Category Miscellaneous that means Marginal Path Likelihood
Shorthand: MPL,
Full Form: Marginal Path Likelihood
For more information of "Marginal Path Likelihood", see the section below.
What is Marginal Path Likelihood?
MPL measures the likelihood of a particular path in a Bayesian network, which represents the joint probability distribution of a set of random variables. It is calculated by summing the product of conditional probabilities along the path from the root node to the leaf node. Each conditional probability represents the likelihood of a specific state of a child node given the states of its parent nodes.
Key Features of Marginal Path Likelihood
- Efficient Computation: MPL can be computed efficiently using dynamic programming algorithms.
- Model Selection: It is used to compare different Bayesian network structures and select the one with the highest MPL.
- Inference: MPL is employed for probabilistic inference, such as finding the most probable explanation or predicting the state of unobserved variables.
Applications of Marginal Path Likelihood
- Machine Learning: MPL is used in classification, regression, and clustering algorithms.
- Artificial Intelligence: It is applied in expert systems, natural language processing, and computer vision.
- Data Analysis: MPL is employed for data mining, knowledge discovery, and statistical modeling.
Essential Questions and Answers on Marginal Path Likelihood in "MISCELLANEOUS»UNFILED"
What is Marginal Path Likelihood (MPL)?
MPL is a statistical technique used to estimate the parameters of a directed acyclic graph (DAG) model. It is based on the idea of marginalizing over the latent variables in the DAG, allowing for the estimation of conditional probabilities and other parameters of interest.
How does MPL differ from other methods for estimating DAG models?
Unlike other methods, MPL does not require the specification of a specific causal ordering of the variables in the DAG. Instead, it estimates the parameters of the model directly from the data, without making any assumptions about the underlying causal relationships. This makes MPL more robust to model misspecifications and more applicable to complex DAGs.
What are the advantages of using MPL?
MPL offers several advantages over other methods for estimating DAG models. These include:
- Flexibility: MPL can be used to estimate a wide range of DAG models, including those with latent variables and feedback loops.
- Robustness: MPL is robust to model misspecifications and can handle complex DAGs with many variables.
- Efficiency: MPL is computationally efficient and can be used to estimate large DAG models.
What are the limitations of MPL? A: MPL also has some limitations, including: Sensitivity to dat
MPL also has some limitations, including:
- Sensitivity to data: MPL can be sensitive to the quality and quantity of the data available.
- Computational complexity: MPL can be computationally intensive for large DAG models.
- Interpretability: The parameters estimated by MPL may not always be easy to interpret in terms of causal relationships.
What are some applications of MPL?
MPL has been used in a variety of applications, including:
- Causal inference: MPL can be used to infer causal relationships between variables in observational data.
- Predictive modeling: MPL can be used to build predictive models that take into account the causal relationships between variables.
- Network analysis: MPL can be used to identify the structure of complex networks, such as social networks and biological networks.
Final Words: MPL is a powerful statistical tool that enables analysts to estimate the probability of specific paths through graphical models. Its efficient computation, model selection capabilities, and wide range of applications make it a valuable technique in various fields. By understanding MPL, data scientists and researchers can effectively leverage Bayesian networks for decision-making, inference, and solving complex problems.
MPL also stands for: |
|
All stands for MPL |