What does JADL mean in EDUCATIONAL
JADL (Joint Advanced Distribution Learning) is a collaborative research initiative aimed at advancing the field of machine learning by developing new methods for learning complex distributions. JADL brings together researchers from academia, industry, and government to work on this challenging problem.
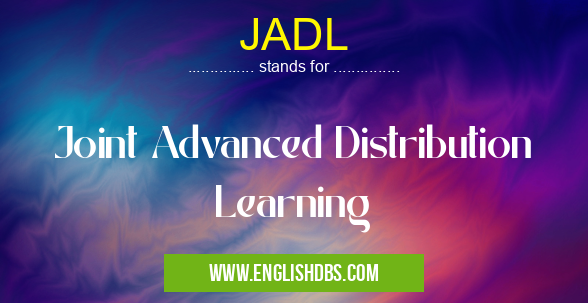
JADL meaning in Educational in Community
JADL mostly used in an acronym Educational in Category Community that means Joint Advanced Distribution Learning
Shorthand: JADL,
Full Form: Joint Advanced Distribution Learning
For more information of "Joint Advanced Distribution Learning", see the section below.
» Community » Educational
Meaning of JADL in Community
Within the machine learning community, JADL is recognized as a significant effort to address the limitations of existing distribution learning methods. By fostering collaboration and knowledge sharing, JADL aims to create a vibrant and supportive community of researchers dedicated to pushing the boundaries of this field.
Full Form of JADL
- Joint
- Advanced
- Distribution
- Learning
What Does JADL Stand For?
JADL stands for Joint Advanced Distribution Learning. It represents the collaborative nature of the initiative, where researchers from diverse backgrounds come together to tackle the complexities of distribution learning.
Essential Questions and Answers on Joint Advanced Distribution Learning in "COMMUNITY»EDUCATIONAL"
What is Joint Advanced Distribution Learning (JADL)?
JADL is a machine learning technique that combines multiple distribution learning algorithms to improve the prediction accuracy of machine learning models. It aims to leverage the strengths of different distribution learning methods to capture complex relationships and patterns in data, leading to more accurate predictions.
What are the benefits of using JADL?
JADL offers several benefits, including:
- Improved prediction accuracy: By combining multiple distribution learning algorithms, JADL can capture diverse perspectives and avoid overfitting, resulting in more accurate predictions.
- Robustness: JADL reduces the risk of relying on a single distribution learning algorithm that may be susceptible to specific data characteristics.
- Adaptability: JADL can adapt to different data types and distributions, making it suitable for a wide range of machine learning applications.
How does JADL work?
JADL typically involves the following steps:
- Data preparation: Data is preprocessed and transformed to make it suitable for distribution learning.
- Distribution learning: Multiple distribution learning algorithms are applied to the data to learn different perspectives on the data distribution.
- Model training: The learned distributions are combined to train a machine learning model.
- Prediction: The trained model is used to make predictions on new data.
What are the applications of JADL?
JADL has potential applications in various domains, such as:
- Natural language processing: Improving the accuracy of text classification, sentiment analysis, and language translation.
- Image recognition: Enhancing the performance of object detection, image segmentation, and facial recognition.
- Healthcare: Predicting patient outcomes, disease diagnosis, and drug effectiveness.
What are the challenges associated with JADL?
JADL can face certain challenges:
- Computational cost: Training multiple distribution learning algorithms can be computationally expensive.
- Model interpretability: Combining multiple distributions can make it difficult to understand and interpret the underlying machine learning model.
- Data availability: JADL requires a substantial amount of data to learn accurate distributions.
Final Words: JADL serves as a catalyst for innovation and progress in the field of machine learning. By bringing together experts from various domains, it fosters interdisciplinary collaboration and accelerates the development of novel distribution learning methods. JADL's contributions are expected to have a profound impact on a wide range of applications, including:
- Natural language processing
- Computer vision
- Robotics
- Healthcare
- Finance