What does BIBO mean in UNCLASSIFIED
BIBO (Bias In Bias Out) is a term used in the context of artificial intelligence (AI) and machine learning (ML) to describe the phenomenon where biased data used to train an AI or ML model results in biased output. This can lead to inaccurate or unfair results when the model is used to make predictions or decisions.
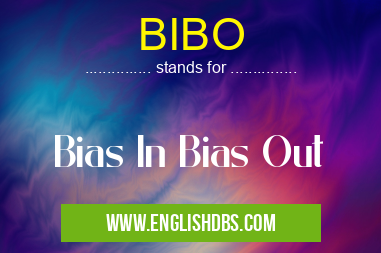
BIBO meaning in Unclassified in Miscellaneous
BIBO mostly used in an acronym Unclassified in Category Miscellaneous that means Bias In Bias Out
Shorthand: BIBO,
Full Form: Bias In Bias Out
For more information of "Bias In Bias Out", see the section below.
Understanding BIBO
- Bias in Data: When the training data used to build an AI or ML model contains systematic errors or prejudices, it can introduce bias into the model. This bias can be intentional or unintentional and can arise from various sources, such as limited representation of certain groups or flawed data collection methods.
- Bias in Output: The biased data used for training the model influences the model's decision-making process, leading to biased output. This means that the model may exhibit unfairness or inaccuracy when applied to real-world scenarios, particularly for underrepresented or marginalized groups.
Addressing BIBO
- Identify and Mitigate Bias in Data: To address BIBO, it is crucial to identify and mitigate biases in the training data. This can include collecting more diverse and representative data, using data cleaning techniques to remove outliers and errors, and employing bias mitigation algorithms during data preprocessing.
- Regularly Monitor and Evaluate: Once the AI or ML model is deployed, it should be regularly monitored and evaluated for potential biases. This involves testing the model's performance across different subsets of data and identifying any discrepancies or unfair outcomes.
- Promote Transparency and Accountability: Transparency in the development and deployment of AI and ML models is essential to address BIBO. Researchers and practitioners should clearly document the data sources, algorithms, and evaluation methods used to ensure accountability and mitigate bias.
Essential Questions and Answers on Bias In Bias Out in "MISCELLANEOUS»UNFILED"
What is BIBO (Bias In Bias Out)?
BIBO stands for Bias In Bias Out. It refers to the phenomenon where AI systems perpetuate and amplify existing biases in the data they are trained on, leading to biased outputs.
How does BIBO occur?
BIBO occurs when training data contains inherent biases. These biases are then incorporated into the model during the training process, leading to outputs that reflect the same biases.
What are the consequences of BIBO?
BIBO can have significant consequences, including:
- Unfair outcomes: Biased models can lead to unfair outcomes for certain groups of people, such as in hiring, credit scoring, or criminal justice.
- Erosion of trust: Biased systems can erode public trust in AI and technology.
- Perpetuation of inequality: BIBO can perpetuate existing inequalities and make it difficult for marginalized groups to access opportunities.
How can BIBO be mitigated? A: Mitigating BIBO requires a multi-faceted approach, including: Bias detection: Identifying and addressing biases in training data and models. Fairness-aware algorithms: Developing algorithms that explicitly consider fairness and reduce bias. Diverse training dat
Mitigating BIBO requires a multi-faceted approach, including:
- Bias detection: Identifying and addressing biases in training data and models.
- Fairness-aware algorithms: Developing algorithms that explicitly consider fairness and reduce bias.
- Diverse training data: Collecting and using training data that represents the diversity of the population the model will serve.
- Human oversight: Regularly reviewing and monitoring AI systems for bias and making adjustments as needed.
Why is it important to address BIBO?
Addressing BIBO is essential for building fair, equitable, and trustworthy AI systems. By mitigating biases, we can ensure that AI benefits all members of society and promotes inclusivity and fairness.
Final Words: BIBO is a significant concern in the field of AI and ML, as it can lead to unfair or inaccurate results. By understanding the causes and implications of BIBO, researchers and practitioners can take proactive steps to mitigate bias in data, improve model fairness, and promote responsible AI and ML practices.