What does MFPN mean in UNCLASSIFIED
MFPN is an acronym for Multiple Feature Pyramid Network. It is a type of deep learning architecture that is used for object recognition, targeting, and segmentation tasks. This network utilizes a pyramid-like structure to extract features from different levels of feature maps. In comparison to other similar networks, the MFPN takes advantage of the shared information between two or more stages in the architecture. By doing so, it allows for better representation and learning of complex objects while also reducing the model size. The use of multiple feature pyramids can help improve both accuracy and efficiency when compared to single feature pyramid networks.
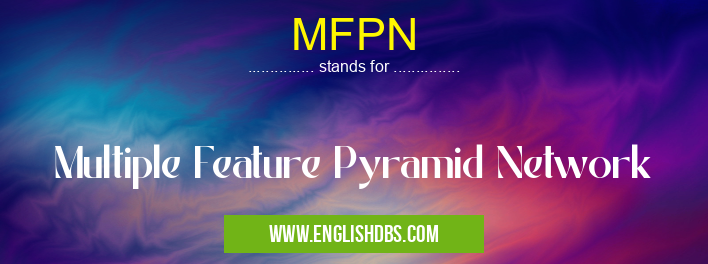
MFPN meaning in Unclassified in Miscellaneous
MFPN mostly used in an acronym Unclassified in Category Miscellaneous that means Multiple Feature Pyramid Network
Shorthand: MFPN,
Full Form: Multiple Feature Pyramid Network
For more information of "Multiple Feature Pyramid Network", see the section below.
Description
The MFPN architecture is composed of multiple levels that are connected by what are known as “feature pyramidsâ€. These pyramids serve to hierarchically group different types of features into distinct bins according to their importance and relevance for the task at hand. Within each level, several layers are used which progressively increase in complexity as more features are added to the network. Starting from the bottom level up, each layer contains a set of pre-trained weights which are responsible for extracting different types of features from the input image or video frame. With additional layers being added sequentially on top, more complex features can be extracted with improved accuracy and speed. At each level within the MFPN architecture, there exists an identical structure in terms of number and type of layers being utilized as well as their layout amongst one another in relation to input data being fed through them. In addition to this uniformity across levels, sharing information between these different tiers also helps reduce redundancies and improves overall performance with respect to memory usage, inference latency and most importantly accuracy metrics such as mean Average Precision (mAP).
Benefits
The main benefit associated with using an MFPN is its ability to improve both accuracy and speed when compared to traditional single-pyramid-based architectures commonly employed in deep learning solutions for computer vision tasks such as object detection, recognition, segmentation etc…. As mentioned previously, this architecture utilizes multiple feature pyramids which enables sharing information between various levels — consequently improving model performance by eliminating redundant features present at each stage of processing data inputs (e.g., images or videos). Furthermore, utilizing more than one pyramid also makes tasks like locating small objects much easier due to increased resolution over various scales — something which single-pyramid architectures tend not be effective at doing by themselves. As a result accuracy metrics such as mAP can benefit from these improvements; making solutions involving MFNP much more attractive options when dealing with large datasets or environments where low latency & high accuracy is required within computationally constrained environments such as embedded systems & mobile applications that often lack hardware resources necessary for traditional methods (i.e., Convolutional Neural Networks).
Essential Questions and Answers on Multiple Feature Pyramid Network in "MISCELLANEOUS»UNFILED"
What is a Multiple Feature Pyramid Network (MFPN)?
A multiple feature pyramid network (MFPN) is a convolutional neural network architecture used in computer vision tasks. It combines different levels of features extracted from an image set to help improve the accuracy of recognition and classification tasks. The network has been used for image-based object detection, semantic segmentation, and instance segmentation.
How does an MFPN work?
An MFPN consists of a series of convolutional layers that extract features from an image set. These features then go through a pyramidal structure where they are combined and further refined at each level. This refined feature map is then classified by the deep learning system to generate the expected results.
What type of applications can benefit from using MFPNs?
MFPNs have been successfully used for object detection and recognition, including pedestrian detection, facial recognition, and vehicle detection. They have also been utilized for medical imaging applications such as tumor segmentation, liver segmentation, and breast cancer diagnosis. In addition, these networks can be used for other image segmentation tasks including natural language processing problems such as sentiment analysis and speech recognition.
Can MFPNs produce more accurate results than traditional methods?
Yes, MFPNs have higher accuracy than traditional methods when working on high-dimensional data sets because they can extract detailed features from each level of the pyramid while combining them in sophisticated ways to refine their predictions at each step of the process. This allows them to produce more accurate results compared to traditional methods in certain situations.
Are there any other advantages provided by MFPNs besides accuracy?
Yes, an advantage of using MFPNs compared to other networks is that they require less computing power since they only need minimal training data to produce reliable outputs. Furthermore, they are more robust due to their ability to recognize various shapes or objects even if those are distorted or occluded in the images being processed.
Are there any limitations with using MFPNs?
One limitation that may arise with using an MFPN is its inability to perform well on extremely small images due to the depth of its architecture which may cause it to miss important details about objects in smaller imagesets. Additionally, since some tasks may require high resolution output such as facial recognition and vehicle detection, this can further decrease the accuracy of prediction produced by the network when dealing with smaller sizes inputs.
Final Words:
In conclusion, the MFPN has many advantages over other architectural forms when it comes down to object recognition - delivering improved speed & accuracy over alternative approaches while also reducing overhead costs associated with redundancies present at each individual tier of processing within traditional convolutional neural networks (CNNs). Moreover its ability make objects easier locate over varying resolutions makes it even more attractive solution for sophisticated computer vision problems - including those found in autonomous vehicles & robotics applications where quick identification & precision actuation/manipulation are essential requirements.