What does THMM mean in UNCLASSIFIED
THMM stands for Topic Hidden Markov Model, which is a statistical machine learning algorithm used to determine the topics in a given text or document. It is commonly used in natural language processing (NLP) for automated text classification, such as sentiment analysis and topic detection. The model relies on both supervised and unsupervised techniques to identify the underlying structures in the text. With this method, it is possible to learn from large amounts of data without manually labeling them. THMM helps automate the process of recognizing patterns in texts and classifying them into various topics. It can also be used to detect anomalies or outliers that may not be easily identified by other means.
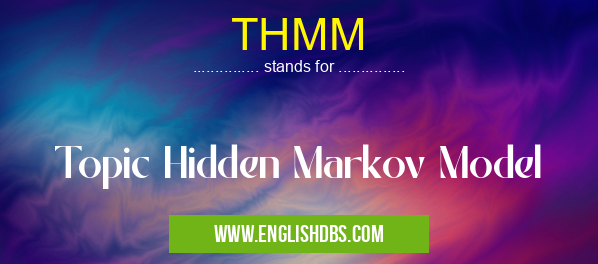
THMM meaning in Unclassified in Miscellaneous
THMM mostly used in an acronym Unclassified in Category Miscellaneous that means Topic Hidden Markov Model
Shorthand: THMM,
Full Form: Topic Hidden Markov Model
For more information of "Topic Hidden Markov Model", see the section below.
What is THMM?
The Topic Hidden Markov Model works by assigning probabilities to each word based on its context, then using those probabilities to determine the most probable sequence of words that make up a topic. The model takes into account various features of the input document such as word frequency, syntax, and part-of-speech tags when calculating these probabilities. Once calculated, these probabilities are used together with additional information about the corpus structure and semantic content to infer the hidden topics present in the document. This allows for accurate text classification without manual intervention.
Essential Questions and Answers on Topic Hidden Markov Model in "MISCELLANEOUS»UNFILED"
What is a Topic Hidden Markov Model (THMM)?
A Topic Hidden Markov Model (THMM) is an unsupervised method for discovering and cluster topics from text documents. It assigns a probability to each word in the documents and then adjusts the probabilities over time as new words are read by the model. THMMs can be used for automatic summarization of text documents, clustering, and document retrieval.
How does a THMM work?
A THMM uses hidden Markov models to discover correlations between words in a document and topics associated with those words. The model looks at how often two words appear together and assigns probabilities based on this information. The model then adjusts these probabilities as it reads more of the document, gradually assigning more weight to certain topics.
What are the benefits of using a THMM?
There are several benefits to using a THMM for natural language processing tasks such as automatic summarization, clustering, and document retrieval. Since the model does not require labeled data or manual feature engineering, it is relatively fast and efficient for extracting important features from large amounts of text data. Additionally, since it does not rely on supervised approaches that require heavy training of models, it allows less experienced users to quickly make use of NLP tools without having to go through complex setup procedures.
How accurate is a THMM?
The accuracy of a THMM depends largely on the quality of the input data and parameters used during training as well as how well-defined the topic clusters are in advance. In general, however, THMMs achieve good results when applied correctly to real-world problems such as topic classification or summarization tasks.
Does a THMM take into account prior knowledge?
Yes, prior knowledge can be incorporated into a THMM through what is known as an “Informative Prior Distribution” which helps guide the algorithm towards finding topics that fit with previously learned information. This means that even if no labeled documents are available, relevant topics can still be discovered using prior knowledge from related domains or research fields.
Are there any limitations with using a THMM?
One limitation with using a THMM for natural language processing tasks is that its performance may suffer when faced with large amounts of noisy input data due to its reliance on statistical methods rather than semantic understanding. Another limitation could be its current lack of support for multi-class classification and sentiment analysis tasks compared to supervised learning techniques such as support vector machines or neural networks which have been widely used in these areas.
Is there any software available that supports creating THMMs?
Yes, there are several libraries available that provide access to implementations of both basic and advanced versions of Topic Hidden Markov Models (THMMs). These include libraries like GenSim (Python), Mallet (Java), Stanford CoreNLP (Java), OpenNLP (C++/Java), Apache UIMA (Java), NLTK (Python) etc.
How long does it typically take to train a Topic Hiddem Markov Model?
The time required for training depends heavily on factors such as size and complexity of dataset being used, hardware specification used etc., however typically for smaller datasets it can take several minutes up to an hour depending upon specific requirements.
Is it possible to combine multiple different types of probabilistic models into one single unified system?
Yes – probabilistic graphical modelling techniques such as Bayesian networks make this possible by allowing us to represent different kinds of relationships between variables within one unified system.
Are there any pre-trained models available for deploying Topic Hidden Markov Models directly out-of-the box?
No – since each particular implementation requires unique parameters tailored specifically to each particular application domain they must all be trained from scratch in order get acceptable results.
Final Words:
In summary, THMM stands for Topic Hidden Markov Model which is a machine learning algorithm used for natural language processing applications such as automated text classification, sentiment analysis and topic detection. It uses supervised and unsupervised techniques to identify underlying structures within text data and assign probabilities to words based on their context within larger documents or corpora of documents. By taking advantage of this model’s powerful ability to detect patterns within texts, organizations can automate their processes more efficiently than ever before while gaining valuable insights from their data sets.