What does PPML mean in ARTIFICIAL INTELLIGENCE
Privacy Preserving Machine Learning (PPML) is a field of data science that uses mathematical methods and computer algorithms to protect the privacy of customers and companies when collecting, storing and analyzing information. The goal of PPML is to make sure that sensitive information such as personal identifying information, credit card numbers and health records are handled securely by limiting the amount of data shared with third parties. By using PPML techniques, organizations can preserve customer privacy while still gathering enough data to make informed decisions.
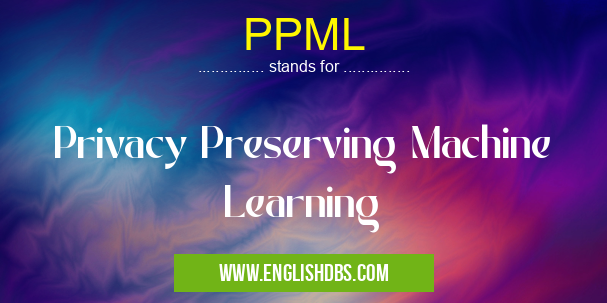
PPML meaning in Artificial Intelligence in Computing
PPML mostly used in an acronym Artificial Intelligence in Category Computing that means Privacy Preserving Machine Learning
Shorthand: PPML,
Full Form: Privacy Preserving Machine Learning
For more information of "Privacy Preserving Machine Learning", see the section below.
Benefits Of PPML
The key advantage of using Privacy Preserving Machine Learning (PPML) is its ability to provide organizations with insights into customer activity without revealing their identity or other sensitive information. As companies strive to understand their customers better and provide better services, they need access to large amounts of personal data which often has privacy implications. Tech giants such as Facebook, Google and Amazon have faced numerous lawsuits due to their use of user data. By utilizing PPML, companies can collect and analyze the necessary data without sacrificing privacy. This also helps build trust with customers as they know their sensitive information is being handled responsibly.
Essential Questions and Answers on Privacy Preserving Machine Learning in "COMPUTING»AI"
What is Privacy Preserving Machine Learning (PPML)?
Privacy Preserving Machine Learning (PPML) is a set of techniques, tools and technologies used to protect data privacy and security during training and prediction tasks. It enables developers to preserve the privacy of the data while still being able to extract valuable insights from it. These techniques include, but are not limited to encryption, cloaking, secure multi-party computation, homomorphic encryption, federated learning and differential privacy.
Why privacy-preserving machine learning is important?
Machine learning models are increasingly being used for more decision processes in a variety of sectors. It is important that any implementation of this technology ensures users’ private data remains secure, as these models can learn about distinct characteristics of individuals if they have access to sensitive information. Privacy-preserving machine learning helps ensure that the user’s data remains protected and confidential throughout the ML process.
How does PPML work?
PPML works by implementing various methods such as homomorphic encryption and differential privacy which help protect data while still allowing models to learn important insights or patterns in the data. Homomorphic encryption allows computations over encrypted values without decrypting them, while differential privacy adds “noise” or perturbation into the dataset so that individual records cannot be identified with accuracy.
What are some benefits of PPML?
The main benefit of using Privacy Preserving Machine Learning (PPML) is that it provides organizations with a secure method for performing analytics on their datasets without compromising user privacy or security. Furthermore, cryptographic techniques such as homomorphic encryption allow model developers to keep all sensitive information secured at each stage along the way– from training through deployment. This means that even if an attacker manages to get access to part of the system, they will unlikely gain meaningful information about individuals represented in the dataset due to its mathematical obfuscation from these techniques.
Is PPML vulnerable?
As with any security tool or method there is always potential for vulnerabilities– especially when it comes protecting user data as it gets passed around multiple nodes during ML operations. Despite this vulnerability risk– organizations should recognize that PPML offers a greater level security than traditional modeling approaches since most attacks occur through unsecured shared storage or transmission systems which are often reduced or eliminated when incorporating these methods into a ML pipeline.
What security guidelines need to be followed when using PPML?
Organizations using PPML should follow standard best practices for securing their infrastructure including employing multi-factor authentication, encrypting all communication channels using TLS protocol versions greater than 1.2 and utilizing regular scans & vulnerability assessments for their networks & databases amongst other measures.
Which algorithms can be used for implementing PPML?
There are several different algorithms that may be employed depending on the specific use case requirements such as Secure Multi Party Computation (MPC), Homomorphic Encryption (HE), Differential Privacy (DP) and Federated Learning (FL). Depending on how secure one wishes his/her application to be – different algorithms may need to be combined in order to reach desired levels of protection.
What is Secure Multi Party Computation (MPC)?
MPC is a cryptographic technique which enables two or more parties who do not trust each other to securely compute joint results together without having any direct knowledge of each other’s input values nor revealing any private details during this process making it ideal for situations involving large datasets between multiple stakeholders.
Is Federated Learning based on virtualized environments?
Federated learning is generally NOT based upon virtualized environment but rather employs distributed computing strategies where compute requests are made across many edge devices simultaneously enabling more efficient processing times as well as increased security since no private data ever leaves its originating device– eliminating possibility of malicious actors accessing said information.
Final Words:
Privacy Preserving Machine Learning (PPML) provides organizations with a way to collect sensitive customer data while still ensuring that customer privacy remains intact. Utilizing advanced techniques such as anonymization, perturbation and encryption help organizations handle customer data responsibly while extracting meaningful insights from it at the same time helping build trust between customers and brands alike.