What does PPML mean in EDUCATIONAL
Privacy Preserving Machine Learning (PPML) is a machine learning technique that helps to protect the security and privacy of sensitive data while still allowing for analysis and predictions. Unlike traditional machine learning algorithms, PPML algorithms take into consideration the potential misuse of data or malicious actors trying to access it. PPML algorithms use encryption, data masking, and other techniques to prevent unauthorized access to sensitive information while still allowing predictive models to be built. The goal of PPML is to allow companies and organizations to safely store and analyze personal and private data while remaining compliant with applicable privacy laws.
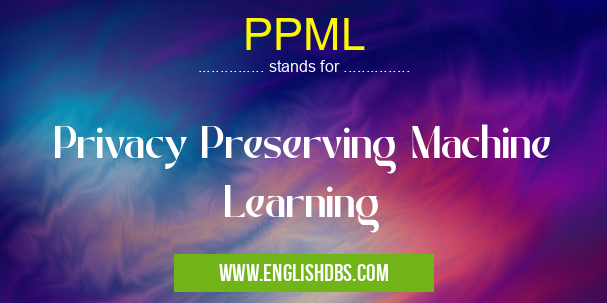
PPML meaning in Educational in Community
PPML mostly used in an acronym Educational in Category Community that means Privacy Preserving Machine Learning
Shorthand: PPML,
Full Form: Privacy Preserving Machine Learning
For more information of "Privacy Preserving Machine Learning", see the section below.
» Community » Educational
What Does Privacy Preserving Machine Learning Do?
The goal of privacy preserving machine learning (PPML) is to develop algorithms that can accurately analyze and predict outcomes based on encrypted or masked data without compromising security or privacy protocols. This allows organizations to build predictive models that are secure yet allow for an adequate level of accuracy. To accomplish this, PPML algorithms employ several encryption methods including homomorphic encryption, secure multiparty computation, secret sharing, attribute-based encryption, secure databases, obfuscation techniques, and differential privacy. These techniques allow for the efficient analysis of encrypted datasets without compromising its security by ensuring only authorized users have access to the decryption key necessary to interpret the results correctly. Furthermore, these methods also provide robust protections against attackers attempting maliciously attempt to access the stored data using brute-force attacks or other intrusive techniques.
Essential Questions and Answers on Privacy Preserving Machine Learning in "COMMUNITY»EDUCATIONAL"
What is Privacy Preserving Machine Learning?
Privacy Preserving Machine Learning (PPML) is a type of data analysis where algorithms are used to create predictive models from data while protecting the privacy of individual users. This technique works by masking or obscuring personal information while still allowing for successful machine learning operations. The result is that companies can use machine learning to gain insights from their data without infringing on the privacy of the people who provided it.
What types of data does PPML protect?
PPML protects any kind of sensitive information such as user ID, location, age, gender, and medical history that can be used to identify an individual. By masking or obfuscating this information, machine learning algorithms can still work accurately without compromising anyone’s privacy.
How does PPML guarantee privacy?
PPML uses anonymization techniques like differential privacy and homomorphic encryption to ensure that private user data remains secure throughout the process. Differential privacy adds noise to the dataset so that it’s impossible to connect back to individuals while homomorphic encryption allows for computations to take place on encrypted data without ever having access to its contents.
Is PPML difficult to implement?
Implementing PPML requires expertise in both machine learning and cryptography, so it is not a simple task for most organizations. However, there are many open-source libraries available which make it easier for developers with less experience in these fields to still take advantage of this powerful technology.
What are some examples of how PPML can be used?
PPML can be used for any application involving predictive analytics based on user data such as personalized recommendations, targeted advertising, fraud detection and disease diagnostics. In each case, the algorithm must be trained using private user information while preventing any potential leakage of sensitive information during this process.
What are some benefits of using PPML?
By using PPML algorithms, companies have access to accurate predictions without infringing upon the privacy rights of their customers. Furthermore, they will benefit from improved trust since individuals feel more secure when their personal data is kept private.
Is it possible for someone's identity to be reconstructed if I use PPML?
No - even if someone were able to gain access to all the anonymized data produced by a PPML algorithm they would not be able to reconstruct an individual's identity due reconstructability attack modeling with non-private techniques such as clustering or social network analysis.
Are there any risks associated with using PPML?
Security breaches could lead malicious actors gaining access sanitized datasets — putting confidential user information at risk. It is important that companies invest in robust security protocols and tools in order protect their datasets against potential threats.
Final Words:
Privacy preserving machine learning is an important tool in protecting the security and privacy of sensitive datasets while still allowing predictive models and analytics operations to be performed on them. Its combination of encryption techniques with other protections gives organizations greater confidence when processing sensitive customer information without having worry about malicious actors accessing their database or violating applicable privacy laws. Ultimately this helps ensure that organizations can securely store and process large amounts of confidential data while giving peace of mind knowing their customer's personal information is being protected from malicious third-parties.
PPML also stands for: |
|
All stands for PPML |