What does RMRF mean in FORESTRY
RMRF stands for Repeated Measures Random Forest, a machine learning algorithm designed for analyzing longitudinal data, where multiple measurements are collected over time for the same set of subjects.
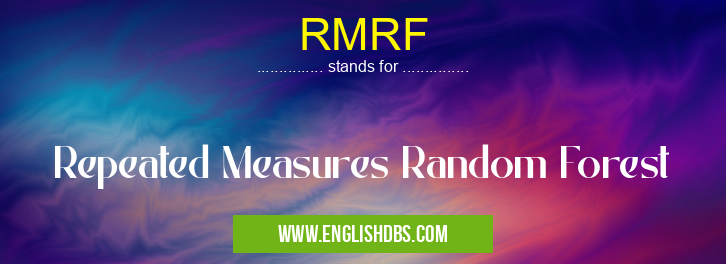
RMRF meaning in Forestry in Community
RMRF mostly used in an acronym Forestry in Category Community that means Repeated Measures Random Forest
Shorthand: RMRF,
Full Form: Repeated Measures Random Forest
For more information of "Repeated Measures Random Forest", see the section below.
Understanding RMRF
RMRF is an ensemble learning method that combines multiple decision trees to enhance prediction accuracy. Each tree is trained on a different subset of the data, and the predictions from all trees are aggregated to make a final decision.
Key features of RMRF include:
- Handling Repeated Measures: RMRF accounts for the correlation between measurements taken over time, improving the model's ability to capture temporal patterns.
- Robustness to Outliers: The ensemble nature of RMRF makes it less susceptible to the influence of outliers in the data.
- Variable Importance: RMRF provides a measure of variable importance, identifying the most influential predictors in the model.
Applications of RMRF
RMRF is widely used in various fields, including:
- Medical research: Analyzing longitudinal health data to identify factors associated with disease progression or treatment outcomes.
- Neuroscience: Studying brain activity over time to understand cognitive processes and disorders.
- Finance: Predicting financial time series and forecasting market trends.
Advantages of RMRF
- Accurate prediction of longitudinal outcomes
- Handling complex data structures with repeated measures
- Robustness and stability in the presence of outliers
- Flexible modeling capabilities for different types of data
Essential Questions and Answers on Repeated Measures Random Forest in "COMMUNITY»FORESTRY"
What is Repeated Measures Random Forest (RMRF)?
RMRF is a machine learning technique that combines the power of random forest with the ability to handle repeated measures data. It is designed to analyze data that is collected over multiple time points or observations from the same subjects.
How does RMRF work?
RMRF operates by building multiple decision trees, each trained on a different subset of the data and a random sample of features. It then combines the predictions from these individual trees to make an overall prediction. This process helps reduce overfitting and improve the accuracy of the model.
What are the advantages of using RMRF? A: RMRF offers several advantages over traditional random forest models: Handles repeated measures data: RMRF can effectively model the correlation and temporal dependencies within repeated measures data. Robust to missing dat
RMRF offers several advantages over traditional random forest models:
- Handles repeated measures data: RMRF can effectively model the correlation and temporal dependencies within repeated measures data.
- Robust to missing data: RMRF is robust to missing data, as it can impute missing values using the values from other observations in the same subject.
- Identifies important features: RMRF provides insights into the importance of different features in predicting the outcome, making it valuable for feature selection.
What are the applications of RMRF?
RMRF has a wide range of applications, including:
- Medical research: Predicting disease progression, treatment response, and prognosis.
- Social sciences: Analyzing longitudinal studies to investigate changes in behavior or attitudes over time.
- Environmental science: Modeling environmental processes and predicting future outcomes.
What software can I use to implement RMRF?
RMRF can be implemented using various software packages, such as:
- R: The R package "rmrfs"
- Python: The Python package "rmrfs"
- SAS: The SAS procedure "PROC RMFOREST"
Final Words: RMRF is a powerful machine learning algorithm designed for analyzing repeated measures data. Its ability to account for the correlation between measurements over time and its robustness make it a valuable tool for researchers and practitioners in various fields. By leveraging RMRF, researchers can gain deeper insights into temporal patterns and make more accurate predictions in longitudinal studies.