What does CGDL mean in EDUCATIONAL
CGDL stands for Conditional Gaussian Distribution Learning. It is a machine learning technique used for solving computer vision and natural language processing tasks like image classification, object detection, semantic segmentation, and machine translation. This technique uses conditional distributions to improve the accuracy of the model by incorporating prior knowledge and additional context into the learning process.
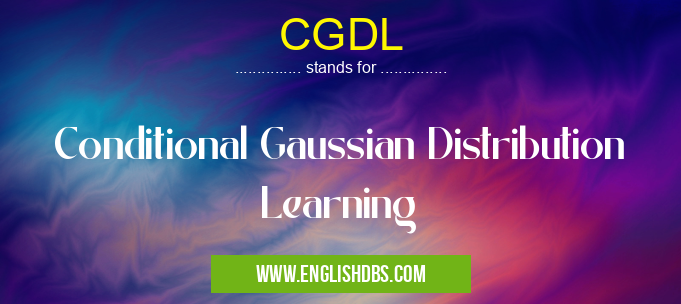
CGDL meaning in Educational in Community
CGDL mostly used in an acronym Educational in Category Community that means Conditional Gaussian Distribution Learning
Shorthand: CGDL,
Full Form: Conditional Gaussian Distribution Learning
For more information of "Conditional Gaussian Distribution Learning", see the section below.
» Community » Educational
Essential Questions and Answers on Conditional Gaussian Distribution Learning in "COMMUNITY»EDUCATIONAL"
What Does CGDL Stand For?
CGDL stands for Conditional Gaussian Distribution Learning.
How Is CGDL Used?
CGDL is typically used in computer vision and natural language processing tasks such as image classification, object detection, semantic segmentation, and machine translation.
What Is Unique About CGDL?
The unique thing about this type of machine learning is that it uses conditional distributions to improve accuracy by incorporating prior knowledge and additional context into the learning process.
What Are Some Benefits Of Using CGDL?
Some benefits include improved accuracy due to prior knowledge being incorporated into the model, faster training times since fewer parameters need to be adjusted for each task, greater flexibility when dealing with complex problems with multiple variables, and decreased risk of overfitting since only relevant information is included.
Where Can I Find More Information On CGDL?
You can find more information on CGDL from online resources such as tutorials and research papers that focus on this type of machine learning technique.
Final Words:
In conclusion, CGDL stands for Conditional Gaussian Distribution Learning which is a type of machine learning technique used in computer vision and natural language processing tasks such as image classification, object detection, semantic segmentation, and machine translation. It has several benefits including improved accuracy due to prior knowledge being incorporated into the model, faster training times since fewer parameters need to be adjusted for each task, greater flexibility when dealing with complex problems with multiple variables, and decreased risk of overfitting since only relevant information is included. Further information about this type of ML can be found through online tutorials and research papers focusing on it.